The added value of a deep learning-based computer-aided detection system on prostate cancer detection among readers with varying level of multiparametric MRI expertise
Over the last decade, localized prostate cancer management had a considerable advance with the worldwide adoption of multiparametric MRI (mpMRI) in clinical practice. Apart from prostate cancer detection, mpMRI has the capability of guiding clinicians during biopsies when combined with transrectal ultrasound (TRUS). MRI not only offers a precise sampling of the suspicious lesion through MRI/TRUS fusion guided biopsy, but also decreases the unnecessary invasive procedures due to its high negative predictive value (NPV) (1). However, the diagnostic accuracy of mpMRI is highly dependent on subjective image interpretation. Introduction of Prostate Imaging Reporting & Data System (PI-RADS) was a substantial step towards a more consistent and standardized reporting. Nonetheless, even with the recent adjustments on the classification system (2), prostate MRI assessment still suffers from poor reproducibility among different readers (3).
Artificial intelligence (AI) in prostate cancer imaging is a rapidly growing field of research that strives to automate processes which otherwise would be time-consuming and challenging. Numerous AI-based prostate cancer algorithms were generated for certain tasks or problems observed in clinical practice, but most could be categorized under (I) lesion detection/localization, (II) lesion/organ segmentation, (III) lesion classification models (4). Purpose of lesion detection algorithms is to facilitate human-based image interpretation and point out suspicious lesions on imaging to the reader. Prior evidence suggests that AI models have the potential to improve cancer detection rates and aid in prostate cancer diagnostic pathway (5). Given the dependency of MRI assessment on interpreter experience, theoretically, readers under training or who are relatively new to the prostate MRI field could boost their diagnostic accuracy with the assistance of AI. The question is can AI supplant the extensive knowledge gained through years of experience?
Lesion detection algorithms have shown promising results, however, their implication on clinical practice needs to be investigated. At this stage, it is too early for the algorithms to completely replace the human readers, however, they might provide a refined data to the radiologists, facilitating their workflow. Multi-reader studies are intended to simulate how AI models would perform in aiding the radiologists in clinical practice (6). These studies generally assess the utility and the added value of AI models by comparing the diagnostic accuracy of radiologist image interpretations with and without AI assistance.
In this study, Labus et al. tested the contribution of a deep learning-based computer-aided detection (DL-CAD) system on prostate MRI evaluation among radiologists with varying levels of experience (7). One hundred and seventy-two consecutive patients and four radiologists (two expert (6 years of experience in prostate MRI: >600 studies, >1,000 studies) and two less experienced (residents with 2 years of experience in MRI: ~50 prostate MRI) were included in that study. Each mpMRI scan was evaluated by the readers with and without DL-CAD assistance with a 4-week wash-out interval. The readers assigned PI-RADS and level of suspicion (LoS) scores to each lesion identified on MRI. Two reads were compared for ISUP Grade Group (GG) ≥1 and ISUP GG ≥2 prostate cancer detection using combined targeted (MRI/TRUS fusion guided) and 12-core systematic biopsy as gold standard.
Multi-reader studies may utilize different workflows such as first reader, second reader and concurrent reader paradigms (8). In the second reader paradigm, the readers are given the CAD predictions only after they evaluate the images without any assistance. The concern with this method is that the role of CAD may be minimal to decision making. Especially for readers who are not familiar with AI and are likely to disregard any assistance from CAD, it may be difficult to incorporate CAD to their clinical workflow with a second reader paradigm. On the other hand, in the first reader paradigm, readers are first given the AI filtered images (i.e., heatmaps, attention boxes) before assessing the conventional images. Notable proportion of prior multi-reader studies have adapted the first-reader paradigms and the readers were only allowed to make their interpretation based on the CAD outputs (9-11) (Table 1). This approach does not leave room for any additional lesion detection apart from algorithm predictions. The reasoning behind this is to assess the sole contribution of CAD on cancer detection. However, integration of AI algorithms to clinical practice will be a gradual process where they will be first used as adjunct tools for aiding the radiologists. Thus, multi-reader studies investigating the impact of CAD systems should allow the readers to make substantial changes on the CAD output. This is possible with a concurrent reader workflow, where the reader is provided both the raw images and CAD output at the same time for interpretation. Labus et al. utilized concurrent reader paradigm in which readers had the freedom of confirming, disregarding, or modifying any CAD output (7). Application of this reading method can be a more realistic approach on of how AI could be translated into the clinical practice.
Table 1
Giannini et al. (12) | Gaur et al. (9) | Greer et al. (10) | Mehralivand et al. (11) | Giannini et al. (13) | Winkel et al. (14) | Labus et al. (7) | |
---|---|---|---|---|---|---|---|
Number of institutions | 1 | 5 | 1 | 5 | 1 | 1 (Publicly available dataset) | 1 |
Cases | 35 | 144 | 110 | 152 | 45 | 43 (PI-RADS ≥4) | 77 |
Controls | 54 | 72 | 53 | 84 | 45 | 57 (PI-RADS <4) | 95 |
Reference standard | Positive Bx (cases), negative Bx or follow-up with PSA/MRI (controls) | RP (cases), negative 12-core Sbx or 24-core TTMB (controls) | RP (cases), negative standard 12-core Sbx (controls) | RP (cases), negative standard 12-core systematic biopsy (controls) | Positive biopsy (cases), negative biopsy or follow-up with PSA/MRI (controls) | Consensus opinion of 3 experienced radiologists | Positive combined TBx and 12-core Sbx (cases), negative Bx (controls) |
No. of readers | 3 readers (experienced) | 9 readers (6 high, 3 moderate) | 9 readers (3 high, 3 moderate, 3 low) | 9 readers (3 high, 3 moderate, 3 low) | 3 readers (non-experienced) | 7 readers (mixed) | 4 readers (2 experienced, 2 less-experienced) |
Definition of experience | Experienced (2–4 years (400–800 scans) | High (>2,000 scans), moderate (500–1,000 scans) | High (>2,000 scans), moderate (500–1,000 scans), low (<500 scans) | High (>3 years or >300 scans/year), moderate (1–3 years or 100–300 scans/year), low (<1 year or <100 scans/year) | Non-experienced (120 to 200 scans) | NA (2–11 years, 35 to 1,000 MRI per year) | Experienced (6 years (>600 scans and >1,000 scans), less-experienced (≈50 prostate scans) |
Reading assignment | All cases | Hybrid | Hybrid | Balanced incomplete block design | All cases | All cases | All cases |
Mean no. of scans per reader | 89 | 76 | 58 | 78 | 90 | 100 | 172 |
1st reading round | T2WI read with CAD assistance | mpMRI read without CAD assistance | mpMRI read without CAD assistance | mpMRI read without CAD assistance | T2WI read with CAD assistance | mpMRI read with/without DL-CAD assistance. (alternating assignments) | mpMRI read with/without DL-CAD assistance. (alternating assignments) |
Washout period | 6 weeks | 4 weeks | 5 weeks | 4 weeks | 6 weeks | 2 weeks | 4 weeks |
2nd reading round | mpMRI read without CAD assistance using favorite reading protocol | CAD output evaluation followed by mpMRI read | CAD output evaluation followed by mpMRI read | CAD output evaluation followed by mpMRI read | mpMRI read without CAD assistance using favorite reading protocol | mpMRI read with/without DL-CAD assistance. (Alternating assignments) | mpMRI read with/without DL-CAD assistance. (Alternating assignments) |
Reading order in 2nd round | Randomized | Randomized | Randomized | Did not indicate | Randomized | Semi-random (two arms were utilized) | Semi-random (two arms were utilized) |
Method of assistance | Probability map superimposed on T2 | Probability map | Probability map | Attention map boxes | Probability map superimposed on T2 | Lesion annotation (mpMRI) and heatmap (T2WI). Lesion-specific PI-RADS and LoS scores | Lesion annotation (mpMRI) and heatmap (T2WI). Lesion-specific PI-RADS and LoS scores |
Reader paradigm | First reader | First reader | First reader | First reader | First reader | Concurrent | Concurrent |
Sample cases | 10 | 3 | 7 | 3 | 10 | 5 | 6 |
Lesion data recorded by readers | Diameter, zone, confidence score and PI-RADS scores (unassisted read only) | Screenshots, zone and PI-RADS scores | Screenshots, zone and PI-RADS scores | Screenshots, zone and PI-RADS scores | Diameter, zone, 5-point confidence score and PI-RADS scores (unassisted read only) | Max diameter annotation, location, PI-RADS and LoS scores | Max diameter annotation, location, PI-RADS and LoS scores |
Reading Time with CAD | Reduced from 220 to 60 sec (mean) | Shorter by 1.2 min (mean) | Low RE: Shorter by 1 min; Moderate RE: Longer by 1.5 min; High RE: Comparable (mean) | Longer by 40 sec (mean) | Reduced from 170 to 66 sec (median) | Reduced from 103 to 81 sec (median) | Reduced from 157 to 150 sec (median) |
Main results of the study | (I) CAD improved sensitivity for both GS >6 disease and lesions with a diameter ≥10 mm; (II) Specificity did not change | (I) Overall sensitivity did not improve; (II) CAD improved detection sensitivity of PI-RADS v2 <3 lesions among moderately experienced readers; (III) CAD improved the patient-level specificity from 45% to 72%, at PI-RADSv2 ≥3 | (I) Sensitivity for index lesion detection improved with CAD assistance; (II) CAD improved sensitivity for PZ lesions, but not for TZ lesions; (III) Sensitivity rates were comparable for index lesions PI-RADS ≥3. (IV) Inter-reader agreement rates improved with CAD-assistance | (I) Overall sensitivity did not improve; (II) CAD improved the sensitivity for TZ lesions; (III) inter-reader agreements did not improve with AI assistance | (I) Sensitivity increased with CAD assistance for clinically significant PCa detection; (II) specificity was comparable between unassisted and assisted reads | (I) DL-CAD improved detection of PI-RADS ≥4 cases across all 7 readers; (II) inter-reader agreement improved with DL-CAD assistance (Fleiss κ from 0.22 to 0.36, P=0.003) | (I) CAD improved the sensitivity for less-experienced readers only; (II) specificity and NPV improved for both experienced and less-experienced readers; (III) DL-CAD assistance improved the correlation between PI-RADS categories and histopathological grades |
CAD, computer-aided detection; PI-RADS, Prostate Imaging Reporting & Data System; Bx, biopsy; PSA, prostate-specific antigen; RP, radical prostatectomy; Sbx, systematic biopsy; TTMB, transperineal template mapping biopsy; TBx, targeted biopsy; NA, not applicable; T2WI, T2-weighted MRI; MRI, magnetic resonance imaging; DL, deep learning; GS, Gleason score; LoS, level of suspicion; mpMRI, multiparametric magnetic resonance imaging; RE, reader experience; PCa, prostate cancer; PZ, peripheral zone; TZ, transition zone; NPV, negative predictive value.
A unique approach in the study was the fully crossed reading design. Four readers were divided into two groups each having both a less experienced and an experienced reader. In the 1st reading round, group 1 evaluated all 172 scans in multiple reading sessions (18–20 scan in each session). They have started the first session without DL-CAD assistance. After each session, the DL-CAD assistance mode was switched on and off in rotation. The 1st reading round was followed by a 4 weeks of washout period. In the 2nd reading round, the scans that were previously read by DL-CAD assistance were assessed without assistance; and the images evaluated without AI assistance in the 1st round were reviewed with DL-CAD assistance in the 2nd reading round. Group 1 evaluated the first session with DL-CAD assistance and continued reading cases with alternating order of assisted read and normal MRI read. On the other hand, group 2 followed an opposite order, where they have started the initial session of 1st reading round with AI assistance, similar to the 2nd reading round of group 1. In the end, all 172 scans were read twice by all readers with and without AI assistance. This design intended to avoid the ‘learning effect’ among readers. Some of the prior multi-reader studies adopted a more straightforward approach where they have had the readers evaluate the scans without any assistance, and after the washout period, the readers interpreted the images with AI assistance (9-11). In this traditional reading design, radiologists are more likely to learn AI’s method of operation and adapt to the faulty predictions by AI. This could lead to higher estimation of specificity in AI-assisted reads. Therefore, crossed reading design may yield more accurate results when assessing CAD performance. However, in Labus et al.’s work, the order of reading sessions was apparently the same in two reading rounds (7). This might be concerning as there is a small possibility that the readers could recall the lesion characteristics from the prior reading round. Complete randomization of image orders and reading sessions could have ensured a more objective evaluation of CAD contribution to cancer detection.
Moreover, Labus and colleagues had all 4 readers evaluate all 172 cases twice with and without CAD assistance (7). The sample size was relatively large and number of scans per reader was higher than previous studies (9-14). Complete overlap of assigned cases between readers allowed assessing inter-reader agreements for each pair of readers and offered a high statistical power. Although there are other multi-reader studies where all readers had to review each case in the study sample (12-14), this method may not be feasible with a larger number of readers from multiple institutions. Therefore, some of the earlier studies have utilized different randomization strategies (such as hybrid, balanced incomplete block design) to reduce the number of scans per reader (9-11). These methods ensure that a reasonable statistical power is maintained and each case is reviewed by more than one reader (15). Future multi-reader studies may utilize these designs to reduce the reading burden.
AI models trained on a specific subset of patients might not be generalizable to overall population. For instance, an algorithm trained only on high quality scans acquired in a single institution might not perform as well on an outside center patient population. Efforts on improving the quality of prostate MRI exams are underway with the recently published guidelines such as PI-RADS v2.1 and prostate imaging quality (PI-QUAL) (2,16). However, the impact of this standardization attempt is likely to take effect in years in many institutions as the quality assurance and quality control steps are dependent on the collective effort of a multi-disciplinary team (17,18). On the other hand, AI could potentially aid in challenging cases (i.e., low quality MRI), if the training dataset consisted of images obtained from scanners with different magnetic strength (3T or 1.5T), MRI vendors, receiver coils (endorectal or surface) (19) (Figure 1). Such diversity could be achieved by multi-institutional efforts, and the algorithm used in Labus et al. study was trained on a diverse dataset of 2,170 prostate scans from 7 institutions (7). The training dataset utilized radiologist assessments as the ground truth rather than histopathological grading. This is a reasonable approach which was also utilized by other algorithms in literature (20), considering that the model intended to aid radiologists in image interpretations. Moreover, training datasets should not be confined to abnormal MRIs only, it should also include MRI exams without any suspicious findings (negative MRIs). The training dataset of the aforementioned AI model comprised of 944 MRI without no lesions, 1,226 MRI with ≥1 lesion with a PI-RADS category of 3 and above. Large, heterogenous, and multi-institutional training datasets are essential to for ensuring the generalizability of an AI model.
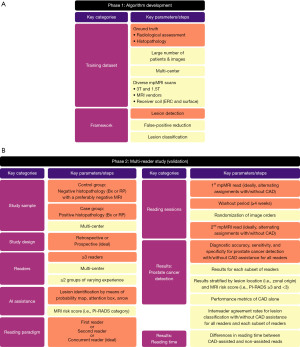
Inherent to the study design, most of recent the multi-reader studies in literature are retrospective (7,9-14). The clinical decisions have already been made on a different, prospective MRI read and patients are either referred to undergo biopsy or relieved from further evaluation. The blinding of readers to the prospective MRI reads, histopathological reports, patient medical history is critical to avoid any bias in their assessment. In Labus et al.’s work, readers only knew that all patients underwent biopsy based on a previous, prospective read which identified at least one lesion with a score ≥3 (7). This might have influenced the reading sessions as the radiologists were likely to be motivated to find at least one intraprostatic lesion. However, in a real-life scenario, patients may present with a PI-RADS 2 lesion or with no lesions at all. Restricting the study sample to a certain subgroup of patients may limit the generalizability of the study findings. Therefore, multi-reader studies should also strive to include patients with negative MRIs and negative systematic biopsy results (also referred as a true control group). Such heterogeneity in study sample is vital in multi-reader studies investigating the clinical utility of an algorithm (9-11). Of course, this may not be possible in an institution where only patients with PI-RADS ≥3 undergo biopsy, but inclusion of another clinical center where 12-core systematic biopsy is performed to the patients without any lesions on MRI based on clinical suspicion may prove useful. Therefore, multi-institutional efforts are much needed not only for training datasets of AI algorithms, but also for the validation datasets as well.
DL-CAD used in Labus et al.’s publication is among the few commercially available AI products designed for prostate cancer detection (7). The algorithm operates following the sequential steps of (I) preprocessing, (II) lesion detection, (III) false-positive reduction, and (IV) classification. False positive reduction step has a particular importance as many AI models suffers from low specificity due to significant number of false positive calls (12,21). In Labus et al.’s study, DL-CAD assistance improved the specificity of less-experienced readers from 0.44 to 0.56 in prostate cancer detection (7). This could be attributed to the integrated false-positive reduction framework. A recent study used a benign prostatic hyperplasia (BPH) nodule filtering step in their algorithm and decreased the mean number of false positives per patient from 0.99 to 0.44 (20). However, inclusion of extra layers in the algorithm to minimize the false positives will naturally come at the cost of decreased sensitivity, due to the inverse association of sensitivity and specificity. Nonetheless, any attempts on improving specificity without compromising sensitivity too much will mitigate unnecessary biopsies in actual patient care.
Besides lesion detection, another challenge with MRI interpretation is variability in lesion risk stratification using classification systems such as PI-RADS. After detecting intraprostatic lesions, the DL-CAD in Labus et al. study (7) provided level of suspicion (LoS) scores along with PI-RADS scores to readers with an interval of 0.1 (ranging from 3.0 to 5.0). This allowed readers to make elaborate interpretations of the AI output. For instance, among two lesions with a PI-RADS score of 4, LoS score of 4.3 is more likely to convince the readers than a score of 3.5. Mere PI-RADS scores do not indicate how certain the AI is in its classification.
Apart from LoS scores of the algorithm, radiologists also provided readers’ level of suspicion (rLoS) scores for each lesion during the reading sessions. rLoS was a more detailed version of PI-RADS scoring as it ranged from 3.0 to 5.0 with an interval of 0.5 allowing the reader to assign intermediate scores such as 3.5 and 4.5. The authors investigated the association between MRI-based scoring and cancer grades. They have concluded that correlation between the MRI-scores and cancer grades improved from 0.45 to 0.57 (P<0.03) with DL-CAD assistance. However, the authors did not focus on the lesion location or MRI-scores as possible factors influencing the DL-CAD performance. Prior evidence suggested that CAD may be more helpful in lesions with lower MRI scores (9) or lesions located in transition zone (11). Further assessment of the CAD contribution on cancer detection stratified by MRI risk categories and lesion localizations would be valuable for investigation.
In addition to the reader performances, multi-reader studies should also report the statistical results of the algorithm alone. This will allow the other investigators to have an overall idea about the capability of the AI model. For instance, an AI model achieving 70% sensitivity on its own is less likely to improve the performance of a reader with a sensitivity of 80%. In their study, Labus et al. indicated the statistical metrics for the DL-CAD system alone (7). The apparent increase in average sensitivity of all readers for ISUP GG ≥1 PCa detection was not high enough (from 79% to 84%) to be statistically significant which could be explained by the limitations of the algorithm as it achieved 79% sensitivity with an AUC of 0.83 on its own. Similarly, the AUC of the less-experienced readers improved from 0.66 to 0.80 (P=0.001) with the assistance of DL-CAD, however, this improvement was not confirmed (0.81 vs. 0.86, P=0.146) among the experienced radiologists.
Labus et al. have noted that the median reading time improved by 7 seconds across all readers (7). However, prior research has shown controversial results regarding the reading times with CAD assistance (10,11,13,14). The reader experience, CAD mode of assistance (heatmap, bounding box, arrow), and the robustness of CAD are among the potential factors affecting the reading times. One should be cautious when interpreting the results regarding reading time comparisons. The slight changes in image evaluation times may not be clinically meaningful, as the readers are aware they are participating in ongoing research and none of their reports will change the patient management. Although these measurements are necessary for guiding the future studies, the influence of CAD on reading times can only be assessed in prospective studies.
In conclusion, multi-reader studies such as Labus et al.’s work (7), hold a great value in simulating the real-life utilization of computer-aided detection (CAD) systems in prostate cancer detection. The knowledge gap between novice and expert readers may be filled with the assistance of CADs. However, the true benefit/caveat of CAD systems will be uncovered in prospective studies, where the CAD-assistance will be used for actual clinical decision making, specifically for prostate biopsies.
Acknowledgments
Funding: None.
Footnote
Provenance and Peer Review: This article was commissioned by the editorial office, Chinese Clinical Oncology. The article did not undergo external peer review.
Conflicts of Interest: Both authors have completed the ICMJE uniform disclosure form (available at https://cco.amegroups.com/article/view/10.21037/cco-22-104/coif). BT reports cooperative research and development agreements with NVIDIA and Philips; royalties from the National Institutes of Health; patents in the field of artificial intelligence. ECY has no conflicts of interest to declare.
Ethical Statement: The authors are accountable for all aspects of the work in ensuring that questions related to the accuracy or integrity of any part of the work are appropriately investigated and resolved.
Open Access Statement: This is an Open Access article distributed in accordance with the Creative Commons Attribution-NonCommercial-NoDerivs 4.0 International License (CC BY-NC-ND 4.0), which permits the non-commercial replication and distribution of the article with the strict proviso that no changes or edits are made and the original work is properly cited (including links to both the formal publication through the relevant DOI and the license). See: https://creativecommons.org/licenses/by-nc-nd/4.0/.
References
- Sathianathen NJ, Omer A, Harriss E, et al. Negative Predictive Value of Multiparametric Magnetic Resonance Imaging in the Detection of Clinically Significant Prostate Cancer in the Prostate Imaging Reporting and Data System Era: A Systematic Review and Meta-analysis. Eur Urol 2020;78:402-14. [Crossref] [PubMed]
- Turkbey B, Rosenkrantz AB, Haider MA, et al. Prostate Imaging Reporting and Data System Version 2.1: 2019 Update of Prostate Imaging Reporting and Data System Version 2. Eur Urol 2019;76:340-51. [Crossref] [PubMed]
- Lee CH, Vellayappan B, Tan CH. Comparison of diagnostic performance and inter-reader agreement between PI-RADS v2.1 and PI-RADS v2: systematic review and meta-analysis. Br J Radiol 2022;95:20210509. [Crossref] [PubMed]
- Belue MJ, Turkbey B. Tasks for artificial intelligence in prostate MRI. Eur Radiol Exp 2022;6:33. [Crossref] [PubMed]
- Suarez-Ibarrola R, Sigle A, Eklund M, et al. Artificial Intelligence in Magnetic Resonance Imaging-based Prostate Cancer Diagnosis: Where Do We Stand in 2021? Eur Urol Focus 2022;8:409-17. [Crossref] [PubMed]
- Hambrock T, Vos PC, Hulsbergen-van de Kaa CA, et al. Prostate cancer: computer-aided diagnosis with multiparametric 3-T MR imaging--effect on observer performance. Radiology 2013;266:521-30. [Crossref] [PubMed]
- Labus S, Altmann MM, Huisman H, et al. A concurrent, deep learning-based computer-aided detection system for prostate multiparametric MRI: a performance study involving experienced and less-experienced radiologists. Eur Radiol 2022; Epub ahead of print. [Crossref] [PubMed]
- Beyer F, Zierott L, Fallenberg EM, et al. Comparison of sensitivity and reading time for the use of computer-aided detection (CAD) of pulmonary nodules at MDCT as concurrent or second reader. Eur Radiol 2007;17:2941-7. [Crossref] [PubMed]
- Gaur S, Lay N, Harmon SA, et al. Can computer-aided diagnosis assist in the identification of prostate cancer on prostate MRI? a multi-center, multi-reader investigation. Oncotarget 2018;9:33804-17. [Crossref] [PubMed]
- Greer MD, Lay N, Shih JH, et al. Computer-aided diagnosis prior to conventional interpretation of prostate mpMRI: an international multi-reader study. Eur Radiol 2018;28:4407-17. [Crossref] [PubMed]
- Mehralivand S, Harmon SA, Shih JH, et al. Multicenter Multireader Evaluation of an Artificial Intelligence-Based Attention Mapping System for the Detection of Prostate Cancer With Multiparametric MRI. AJR Am J Roentgenol 2020;215:903-12. [Crossref] [PubMed]
- Giannini V, Mazzetti S, Armando E, et al. Multiparametric magnetic resonance imaging of the prostate with computer-aided detection: experienced observer performance study. Eur Radiol 2017;27:4200-8. [Crossref] [PubMed]
- Giannini V, Mazzetti S, Cappello G, et al. Computer-Aided Diagnosis Improves the Detection of Clinically Significant Prostate Cancer on Multiparametric-MRI: A Multi-Observer Performance Study Involving Inexperienced Readers. Diagnostics (Basel) 2021;11:973. [Crossref] [PubMed]
- Winkel DJ, Tong A, Lou B, et al. A Novel Deep Learning Based Computer-Aided Diagnosis System Improves the Accuracy and Efficiency of Radiologists in Reading Biparametric Magnetic Resonance Images of the Prostate: Results of a Multireader, Multicase Study. Invest Radiol 2021;56:605-13. [Crossref] [PubMed]
- Huang EP, Shih JH. Assigning readers to cases in imaging studies using balanced incomplete block designs. Stat Methods Med Res 2021;30:2288-312. [Crossref] [PubMed]
- Giganti F, Allen C, Emberton M, et al. Prostate Imaging Quality (PI-QUAL): A New Quality Control Scoring System for Multiparametric Magnetic Resonance Imaging of the Prostate from the PRECISION trial. Eur Urol Oncol 2020;3:615-9. [Crossref] [PubMed]
- Barrett T, de Rooij M, Giganti F, et al. Quality checkpoints in the MRI-directed prostate cancer diagnostic pathway. Nat Rev Urol 2022; Epub ahead of print. [Crossref] [PubMed]
- Stabile A, Giganti F, Kasivisvanathan V, et al. Factors Influencing Variability in the Performance of Multiparametric Magnetic Resonance Imaging in Detecting Clinically Significant Prostate Cancer: A Systematic Literature Review. Eur Urol Oncol 2020;3:145-67. [Crossref] [PubMed]
- Turkbey B, Haider MA. Artificial Intelligence for Automated Cancer Detection on Prostate MRI: Opportunities and Ongoing Challenges, From the AJR Special Series on AI Applications. AJR Am J Roentgenol 2022;219:188-94. [Crossref] [PubMed]
- Mehralivand S, Yang D, Harmon SA, et al. A Cascaded Deep Learning-Based Artificial Intelligence Algorithm for Automated Lesion Detection and Classification on Biparametric Prostate Magnetic Resonance Imaging. Acad Radiol 2022;29:1159-68. [Crossref] [PubMed]
- Hosseinzadeh M, Saha A, Brand P, et al. Deep learning-assisted prostate cancer detection on bi-parametric MRI: minimum training data size requirements and effect of prior knowledge. Eur Radiol 2022;32:2224-34. [Crossref] [PubMed]