Establishment and validation of a prognostic model for gastric cancer patients of Hebei Province in China
Highlight box
Key findings
• The multivariable group outperformed Tumor Node Metastasis (TNM) group. Cox and random survival forest performed better than survival tree and gradient boosting machine. The nomogram may facilitate clinicians to predict survival of gastric cancer patients in Hebei Province.
What is known and what is new?
• Machine learning has been widely applied. It’s unknown whether existing models are suitable for the prognosis of gastric cancer patients in Hebei Province.
• Three tree-based machine learning and Cox, were used to build gastric cancer patients prognostic models. Additionally, California Chinese hospitalized gastric cancer patients in Surveillance, Epidemiology, and End Results (SEER) database were used as external test dataset. We compared multivariable group with TNM group.
What is the implication, and what should change now?
• This study fills in the blank of prognostic models for hospitalized gastric cancer patients in Hebei Province. We will try to compare more models to establish a more comprehensive prognostic model.
Introduction
Background
Gastric cancer is one of the top ten common malignant tumors worldwide (1). According to Global Cancer Statistics 2020 (GLOBOCAN 2020) (1), 43.9% of new cases and 48.6% of deaths occured in China. Hebei Province, located in North China, is the only province that includes all landforms in China. The incidence and mortality of gastric cancer of Hebei Province are higher than that of China (2). In recent decades, the incidence and mortality of gastric cancer patients are declining in many developed countries, but it still causes a huge disease burden (3). More than 90% of early gastric cancer patients survive for 5 years or more after receiving surgery (4,5). However, the prognosis of late gastric cancer patients is poor, with a 5-year survival rate of about 20% for stage III patients and about 7% for stage IV patients (6). The Tumor Node Metastasis (TNM) staging system, established by the American Joint Committee on Cancer (AJCC) and Union for International Cancer Control (UICC), is a practical method for evaluating the prognosis of gastric cancer patients and is widely used worldwide (6). However, TNM staging system, which is only based on tumor infiltration, tumor size, lymph node metastasis, and distant metastasis, has inevitable limitations. The patients within the same stage may have different survival outcomes, and patients in different stages may also have the same survival outcome (7). In addition, many studies have shown that age, gender, tumor size, and therapy are closely related to the survival of gastric cancer patients (7-9). The occurrence and development of gastric cancer are very complex. Many clinical and pathological features can influence prognosis, most of which are multidimensional and non-linear relationships (10). Therefore, it is necessary to integrate prognostic factors selected from comprehensive clinical information and establish a more accurate survival prediction model for gastric cancer patients.
Rationale and knowledge gap
In order to obtain better models for predicting patients’ survival, many survival prediction tools have been developed, including parametric, semi-parametric, and non-parametric methods. The parametric method requires that the survival time meet the normal distribution, and the accelerated failure time (AFT) model is commonly used (11). Most survival data are non-normal distribution, so parametric method is not commonly used. The semi-parametric method is based on the Cox proportional hazard (PH) regression model, which has been applied in many studies to predict the survival of gastric cancer (8,12-18). However, due to the need to comply with the PH assumption, the use of Cox is limited. In order to eliminate these constraints, a series of non-parametric methods have been developed for cancer survival prediction, which can consider the interaction effects among variables. In the non-parametric methods, the most common methods were tree-based machine learning methods [including survival trees (ST), random survival forests (RSF), gradient boosting machines (GBM), etc.] (19-30). The ST is a machine learning method that constructs a tree structure by splitting nodes by maximizing survival differences until all terminal nodes containing only the minimum number of unique events (31,32). Both RSF and GBM are combined of a large number of ST. RSF uses the bootstrap method to extract sub samples from the original samples to construct a ST, averaging the cumulative risk function of each ST and ultimately obtaining the total cumulative risk function (33). GBM is a machine learning method based on gradient descent boosting. The fundamentals of GBM are training a new ST according to the negative gradient information of the loss function based on the current ST, and combining the trained newborn ST with the existing ST (34).
Machine learning has been widely applied in data analysis such as medical care, and is an effective tool for improving clinical strategies. Banerjee et al., Qiu et al., Du et al., van Zutphen et al. and Lin et al. showed that RSF performs better than Cox in predicting the survival of thyroid cancer, glioma, oropharyngeal cancer, colorectal cancer and liver cancer (35-40). Samara’s research shows that RSF performs better than linear support vector machine (SVM), Adaboost, Bagging and other machine learning algorithms in predicting the survival of glioblastoma (41). At present, there is no research on the survival prediction of gastric cancer patients in Hebei Province, and it is unknown whether the existing survival prediction models are suitable for the survival prediction of gastric cancer patients in Hebei Province.
Objective
This study was based on the information of hospitalized gastric cancer patients from the Hebei Cancer Registration Project during 2016 and 2017. Three tree-based machine learning methods (ST, RSF, and GBM) and the Cox PH regression model, were used to build gastric cancer patients survival prediction models. Additionally, California Chinese gastric cancer patients from hospitals in the Surveillance, Epidemiology, and End Results (SEER) database were used as external test dataset. In addition, we compared the multivariable group with TNM group. This study aimed to build the best survival prediction model, identify high-risk gastric cancer patients as soon as possible, and provide reference for clinical doctors to develop specific treatment plans for patients and allocate medical resources reasonably. This manuscript is written in accordance with the TRIPOD reporting checklist (available at https://cco.amegroups.com/article/view/10.21037/cco-23-85/rc).
Methods
Data source
Data of development dataset were recruited from Hebei Cancer Registration Project. We included 1,993 hospitalized gastric cancer patients who were diagnosed between 1 January 2016 to 31 December 2017. The following patients were excluded: (I) repeatable cases (N=6); (II) non-initial diagnosed cases (N=6); (III) previously suffered from other malignant tumors (N=17); (IV) individuals with incomplete survival information (N=124) (Figure 1).
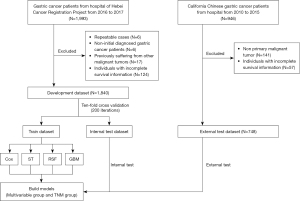
Data of external test dataset were obtained from the “Incidence - SEER Research Plus Data, 17 Registries, Nov 2021 Sub (2000-2019)” of SEER database. To exclude differences among hospital source data and population data, as well as differences between different races, we selected the hospitalized gastric cancer patients of California Chinese from 2010 to 2015. A total of 946 patients were included. The exclusion criteria are as follows: (I) non-initial diagnosed cases (N=141); (II) individuals with incomplete survival information (N=57) (Figure 1).
Predictors and outcomes
This study included 13 predictors, including gender, age, area, marital status, tumor site, grade, TNM stage, T (tumor) stage, N (node) stage, M (metastasis) stage, surgery, radiotherapy, and chemotherapy. The predictors of TNM stage, T stage, N stage, M stage were referring to AJCC Clinical Stage, 7th edition.
The outcome variables included “survival months” and “3-year cancer-specific survival (CSS) status” or “5-year CSS status”.
Data of development dataset were collected and strictly controlled by trained cancer registration professionals. The survival outcomes were obtained by active and passive follow-up. Passive follow-up was mainly obtained through all-causes-of-death survey database, rehospitalization information, outpatient information and medical insurance information. Active follow-up was obtained by trained professionals trimonthly. The deadline date of follow-up was December 31, 2022.
Establishment and validation of the model
The development dataset was used to train and internally test the model with ten-fold cross validation of 200 iterations. The external test dataset was used for external test. Four models (including Cox, ST, RSF and GBM) were used to establish the survival prediction model for gastric cancer in Hebei Province. Additionally, we compared the multivariable group (including multiple variables) with TNM group (including AJCC 7th edition T stage, N stage, and M stage) (Figure 1).
Evaluation indicators of the model
The Harrell’s consistency index (C-index) and area under the receiver operating characteristic curve (AUC) were used to evaluate the model’s differentiation. The calibration curve was used to evaluate the consistency between the predicted values and the actual observed values. The 45-degree straight gray line of calibration curve represents the perfect match between the observed (y-axis) and predicted (x-axis) survival probabilities. A closer distance between two curves indicates higher consistency.
Statistical analysis
Software R (version 4.1.2) was used for data analysis. “randomForestSRC” package was used to impute missing values. “survival”, “rpart”, “randomForestSRC” and “gbm” packages were used to develop the models. The value of P<0.05 indicates a statistically significant difference.
Ethical statement
The study was conducted in accordance with the Declaration of Helsinki (as revised in 2013). For external test dataset from the SEER database, the authors obtained authorization to access the SEER Research Data supported by the National Cancer Institute with approval number 11241-Nov2021. Because public and anonymous data from the SEER database were used, informed patient consent was not required. For development dataset from Hebei Cancer Registration Project, the Ethics Committee of the Fourth Hospital of Hebei Medical University/the Tumor Hospital of Hebei Province has confirmed that no ethical approval is required. Individual consent for this retrospective analysis was waived.
Results
Demographic characteristics of gastric cancer patients
After inclusion and exclusion, 1,840 patients and 748 patients with gastric cancer were included in the development dataset and external test dataset, respectively. The 1-, 3-, and 5-year CSS rates of the development dataset were 82.61%, 57.07%, and 44.48%, respectively. The 1-, 3-, and 5-year CSS rates of the external test dataset were 68.58%, 47.99%, and 42.91%, respectively. The detail of demographic characteristics of the imputation data were shown in Table 1.
Table 1
Variables | Development dataset, n (%) | External test dataset, n (%) | P value† |
---|---|---|---|
Total | 1,840 (100.00) | 748 (100.00) | |
Gender | <0.001 | ||
Male | 1,442 (78.37) | 413 (55.21) | |
Female | 398 (21.63) | 335 (44.79) | |
Age (years) | <0.001 | ||
<55 | 373 (20.27) | 131 (17.51) | |
55–64 | 692 (37.61) | 119 (15.91) | |
65–74 | 626 (34.02) | 183 (24.47) | |
>75 | 149 (8.10) | 315 (42.11) | |
Areas | <0.001 | ||
Urban | 649 (35.27) | 726 (97.06) | |
Rural | 1191 (64.73) | 22 (2.94) | |
Marital status | <0.001 | ||
Married | 1,820 (98.91) | 532 (71.12) | |
Unmarried | 6 (0.33) | 75 (10.03) | |
Others‡ | 14 (0.76) | 141 (18.85) | |
Tumor site | <0.001 | ||
Cardia | 910 (49.46) | 93 (12.43) | |
Overlapping | 31 (1.68) | 53 (7.09) | |
Other site | 899 (48.86) | 602 (80.48) | |
Grade | 0.494 | ||
G1–G2§ | 519 (28.21) | 221 (29.55) | |
G3–G4¶ | 1,321 (71.79) | 527 (70.45) | |
TNM stage | <0.001 | ||
I | 356 (19.35) | 210 (28.07) | |
II | 354 (19.24) | 124 (16.58) | |
III | 910 (49.46) | 195 (26.07) | |
IV | 220 (11.96) | 219 (29.28) | |
T stage | <0.001 | ||
T1 | 267 (14.51) | 217 (29.01) | |
T2 | 201 (10.92) | 92 (12.30) | |
T3–T4 | 1,372 (74.57) | 439 (58.69) | |
N stage | <0.001 | ||
N0 | 671 (36.47) | 389 (52.01) | |
N1 | 298 (16.20) | 192 (25.67) | |
N2 | 372 (20.22) | 70 (9.36) | |
N3 | 499 (27.12) | 97 (12.97) | |
M stage | <0.001 | ||
M0 | 1,626 (88.37) | 538 (71.93) | |
M1 | 214 (11.63) | 210 (28.07) | |
Surgery | <0.001 | ||
No | 347 (18.86) | 321 (42.91) | |
Yes | 1,493 (81.14) | 427 (57.09) | |
Radiotherapy | <0.001 | ||
No | 1,790 (97.28) | 592 (79.14) | |
Yes | 50 (2.72) | 156 (20.86) | |
Chemotherapy | <0.001 | ||
No | 709 (38.53) | 376 (50.27) | |
Yes | 1,131 (61.47) | 372 (49.73) |
†, P value was derived from the Chi-square test performed to compare the development dataset and external test dataset; ‡, others included divorced, separated and widowed; §, G1–G2 represented well differentiated or moderately differentiated; ¶, G3–G4 represented poorly differentiated or undifferentiated. TNM, Tumor Node Metastasis; T stage, tumor stage; N stage, node stage; M stage, metastasis stage.
Figure 2 shows the proportion of stages of gastric cancer patients in the development dataset and external test dataset. The proportion of stage I, II, III, and IV of gastric cancer patients in the development dataset were 19.35%, 19.24%, 49.45%, and 11.96%, respectively. The proportion of stage I, II, III, and IV of gastric cancer patients in the external test dataset were 28.07%, 16.58%, 26.07%, and 29.28%, respectively. The proportion of stage III and IV of overall patients in the development dataset (61.41%), was higher than that in the external test dataset (55.35%). The proportion of stage III and IV of female patients (65.83%) was higher than that of male patients (60.19%) in the development dataset. The proportion of stage III and IV of rural patients (62.46%) was higher than that of urban patients (59.48%) in the development dataset. The proportion of stage III and IV of female patients (58.51%) was higher than that of male patients (52.78%) in the external test dataset. The proportion of stage III and IV of rural patients (59.09%) was higher than that of urban patients (55.24%) in the external test dataset.
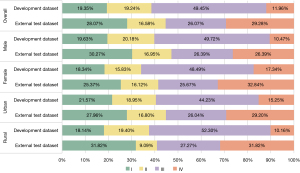
Variables selection
According to Kaplan-Meier curves and log-rank tests on the above variables, it can be concluded that the ten variables (including TNM stage, gender, age, tumor site, grade, T stage, N stage, M stage, surgery, and radiotherapy) met the PH hypothesis (Figures S1,S2).
Considering that TNM stage was obtained by combining T stage, N stage and M stage, we excluded TNM stage from predictors. In the multivariable group of ST, RSF and GBM, we included 12 predictors (including gender, age, area, marital status, tumor site, grade, T stage, N stage, M stage, surgery, radiotherapy, and chemotherapy). In the multivariable group of Cox, we only included nine variables which met the PH assumption (including gender, age, tumor site, grade, T stage, N stage, M stage, surgery, and radiotherapy).
Model performance
Table 2 and Figure 3 showed the C-index of different models for predicting 3- and 5-year CSS of gastric cancer patients in the multivariable group and TNM group. In the 3-year CSS cohort, the Cox [C-index: 0.75, 95% confidence interval (CI): 0.71–0.79], RSF (C-index: 0.79, 95% CI: 0.75–0.82), and GBM (C-index: 0.76, 95% CI: 0.72–0.79) were greater than ST (C-index: 0.72, 95% CI: 0.69–0.76) of train dataset of multivariable group. In the multivariable group, except for ST, the C-index of other models were higher than 0.70. The Cox (C-index: 0.71, 95% CI: 0.67–0.75), RSF (C-index: 0.72, 95% CI: 0.68–0.75), and C-index of GBM (C-index: 0.71, 95% CI: 0.68–0.75) were greater than ST (C-index: 0.69, 95% CI: 0.65–0.73) of train dataset of TNM group. The C-index of Cox, RSF and GBM were greater than ST in every cohort and group.
Table 2
Cohort | Three-year CSS cohort, mean (95% CI) | Five-year CSS cohort, mean (95% CI) | |||||
---|---|---|---|---|---|---|---|
Train dataset | Internal test dataset | External test dataset | Train dataset | Internal test dataset | External test dataset | ||
Multivariable group | |||||||
Cox | 0.75 (0.71–0.79) | 0.73 (0.63–0.84) | 0.75 (0.70–0.80) | 0.71 (0.68–0.74) | 0.71 (0.62–0.80) | 0.76 (0.72–0.81) | |
ST | 0.72 (0.69–0.76) | 0.70 (0.59–0.80) | 0.73 (0.68–0.77) | 0.68 (0.65–0.71) | 0.68 (0.60–0.77) | 0.73 (0.68–0.77) | |
RSF | 0.79 (0.75–0.82) | 0.79 (0.68–0.90) | 0.77 (0.72–0.81) | 0.74 (0.71–0.77) | 0.71 (0.62–0.80) | 0.76 (0.72–0.81) | |
GBM | 0.76 (0.72–0.79) | 0.79 (0.69–0.90) | 0.78 (0.74–0.83) | 0.72 (0.69–0.75) | 0.71 (0.62–0.80) | 0.78 (0.73–0.82) | |
TNM group | |||||||
Cox | 0.71 (0.67–0.75) | 0.71 (0.59–0.82) | 0.66 (0.61–0.71) | 0.67 (0.64–0.71) | 0.68 (0.58–0.78) | 0.68 (0.63–0.73) | |
ST | 0.69 (0.65–0.73) | 0.70 (0.58–0.81) | 0.60 (0.54–0.65) | 0.66 (0.63–0.69) | 0.66 (0.57–0.75) | 0.68 (0.63–0.73) | |
RSF | 0.72 (0.68–0.75) | 0.70 (0.58–0.81) | 0.68 (0.62–0.73) | 0.68 (0.64–0.71) | 0.68 (0.58–0.77) | 0.67 (0.62–0.73) | |
GBM | 0.71 (0.68–0.75) | 0.70 (0.59–0.81) | 0.67 (0.61–0.72) | 0.67 (0.64–0.71) | 0.68 (0.58–0.77) | 0.67 (0.62–0.72) |
CSS, cancer-specific survival; CI, confidence interval; ST, survival trees; RSF, random survival forests; GBM, gradient boosting machines; TNM, Tumor Node Metastasis.
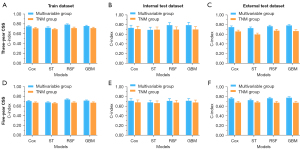
In the 5-year CSS cohort, the C-index of Cox (C-index: 0.71, 95% CI: 0.68–0.74), RSF (C-index: 0.74, 95% CI: 0.71–0.77), and GBM (C-index: 0.72, 95% CI: 0.69–0.75) were greater than ST (C-index: 0.68, 95% CI: 0.65–0.71) of train dataset of multivariable group. The Cox (C-index: 0.67, 95% CI: 0.64–0.71), RSF (C-index: 0.68, 95% CI: 0.64–0.71), and C-index of GBM (C-index: 0.67, 95% CI: 0.64–0.71) were greater than ST (C-index: 0.66, 95% CI: 0.63–0.69) of train dataset of TNM group. All C-index of TNM group were less than 0.70 in 5-year CSS cohort.
Whether in the 3-year CSS cohort or in the 5-year CSS cohort, the C-index of multivariable group was greater than that of the TNM group (Figure 3).
Figure 4 depicted the receiver operating characteristic (ROC) curves of different models for predicting the 3-year CSS of gastric cancer in the multivariable group and TNM group. In the train dataset of multivariable group, the AUC values of Cox, ST, RSF, and GBM were 0.81 (95% CI: 0.79–0.83), 0.78 (95% CI: 0.76–0.80), 0.86 (95% CI: 0.84–0.87), and 0.82 (95% CI: 0.80–0.84), respectively. In the train dataset of TNM group, the AUC values of Cox, ST, RSF, and GBM were 0.76 (95% CI: 0.74–0.79), 0.74 (95% CI: 0.72–0.76), 0.77 (95% CI: 0.75–0.79), and 0.77 (95% CI: 0.74–0.79), respectively. In every dataset or every group, the AUC value of ST was lower than that of other three models. In every dataset, the AUC values of multivariable group were higher than those of the TNM group.
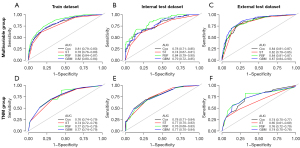
Figure S3 depicted the ROC curves of different models for predicting the 5-year CSS of gastric cancer in the multivariable group and TNM group. In the train dataset of multivariable group, the AUC values of Cox, ST, RSF and GBM are 0.72 (95% CI: 0.70–0.75), 0.70 (95% CI: 0.67–0.72), 0.81 (95% CI: 0.79–0.83) and 0.74 (95% CI: 0.71–0.77), respectively. In the train dataset of TNM group, the AUC values of Cox, ST, RSF, and GBM were 0.69 (95% CI: 0.67–0.72), 0.68 (95% CI: 0.65–0.71), 0.69 (95% CI: 0.66–0.72), and 0.69 (95% CI: 0.67–0.72), respectively. In every dataset or every group, the AUC value of ST was lower than that of other three models. In every dataset, the AUC values of multivariable group were higher than those of the TNM group.
Figure 5 depicted the calibration curves of different models for predicting the 3-year CSS of gastric cancer patients in the multivariable group and TNM group. In multivariable group, the consistency of RSF and Cox were superior than that of GBM and ST; the predicted value of GBM was higher than the actual value, and the predicted value of ST was lower than the actual value. In the TNM group, the performance of the calibration curves for the train dataset and internal test dataset were similar to that in the multivariable group. The consistency of calibration curve of every model in the external test dataset was slightly inferior to that in train dataset and internal test dataset. In the 3-year CSS, the consistency of the calibration curves of all models in the multivariable group were better than that in the TNM group.
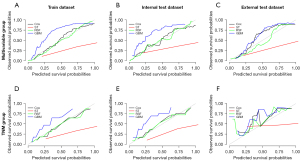
Figure S4 plotted the calibration curves of different models for predicting the 5-year CSS of gastric cancer patients in the multivariable group and TNM group. The results were similar to those of the 3-year CSS cohort. In the multivariable group, the consistency of Cox and RSF were superior to that of GBM and ST. In the TNM group, the performance of the calibration curves of the train dataset and internal test dataset were similar to that of the multivariable group, with RSF and Cox having better consistency than GBM and ST. The calibration curve consistency of each model in the external test dataset was poor. In the 5-year CSS cohort, the consistency of the calibration curves of all models in the multivariable group was better than that of the TNM group.
Outcome of Cox
From above analysis, we can achieve that the predictive performance of Cox and RSF were superior to that of ST and GBM, and the predictive performance of Cox was similar to that of RSF. Due to the fact that the current application of RSF wasn’t as simple as Cox, in order to better apply the model to practice, we visualized the Cox’s results by drawing a forest plot and constructed a nomogram for clinicians to predict patients’ survival.
Figure S5 drawn a forest plot of influencing factor of gastric cancer patients’ survival based on the Cox of development dataset in multivariable group. The results show that age, tumor site, T stage, N stage, M stage, and surgery situation were the influencing factors for the survival prognosis of gastric cancer patients (P<0.05). Poorer CSS was associated with elder in age [≥75 years with hazard ratio (HR) =1.48, 95% CI: 1.15–1.89, P=0.002] compared to <55 years; having overlapping tumor sites (HR =1.68, 95% CI: 1.11–2.55, P=0.014) compared to gastric cardia; T3–T4 stage (HR =2.91, 95% CI: 2.07–4.09, P<0.001) compared to T1 stage; N2 stage (HR =1.68, 95% CI: 1.36–2.06, P<0.001) and N3 stage (HR =2.22, 95% CI: 1.83–2.70, P<0.001) compared to N0 stage; M1 stage (HR =1.85, 95% CI: 1.53–2.24, P<0.001) compared to M0 stage. Improved CSS was associated with other tumor sites (HR =0.79, 95% CI: 0.69–0.91, P=0.001) compared to gastric cardia; and underwent surgery (HR =0.42, 95% CI: 0.35–0.49, P<0.001) compared to didn’t undergo surgery.
Figure 6 depicted a nomogram established based on multivariable Cox PH regression model to predict the 3- and 5-year CSS of gastric cancer patients based on development dataset. By bringing the variables of the patient in nomogram, the scores of each variable can be obtained. Finally, the scores of each variable were added up to obtain the 3- and 5-year CSS of the patient. For example, we brought the variables of patient number 36189409 of external test dataset into the nomogram, we obtained the 3- and 5-year CSS were 0.264 (0.150–0.466) and 0.180 (0.087–0.374), respectively.
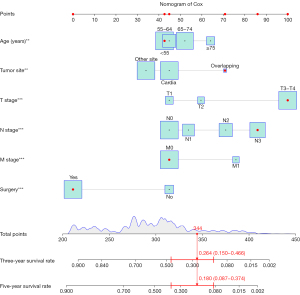
Sensitivity analysis
To eliminate the bias caused by different variables of different models, we built a same variables group for sensitivity analysis. In the same variables group, the variables included in the ST, RSF, and GBM were consistent with that in Cox. The variables included gender, age, tumor site, grade, T stage, N stage, M stage, surgery, and radiotherapy. Table 3 and Figures S6,S7 respectively showed the C-indexes, ROC curves and calibration curves of different models that predicted the 3- and 5-year CSS of gastric cancer patients in the same variables group. From the obtained results, it can be seen that the predicting performance of the same variables group were similar to those of the multivariable group.
Table 3
Models | Train dataset, mean (95% CI) | Internal test dataset, mean (95% CI) | External test dataset, mean (95% CI) |
---|---|---|---|
Three-year CSS cohort | |||
Cox | 0.75 (0.72–0.79) | 0.70 (0.58–0.82) | 0.75 (0.70–0.80) |
ST | 0.73 (0.69–0.76) | 0.68 (0.56–0.80) | 0.73 (0.69–0.78) |
RSF | 0.77 (0.74–0.80) | 0.76 (0.65–0.86) | 0.76 (0.71–0.81) |
GBM | 0.75 (0.72–0.79) | 0.77 (0.67–0.87) | 0.77 (0.73–0.82) |
Five-year CSS cohort | |||
Cox | 0.71 (0.67–0.74) | 0.71 (0.61–0.80) | 0.76 (0.72–0.80) |
ST | 0.68 (0.65–0.71) | 0.68 (0.59–0.76) | 0.73 (0.68–0.77) |
RSF | 0.72 (0.70–0.75) | 0.70 (0.61–0.80) | 0.76 (0.71–0.80) |
GBM | 0.71 (0.68–0.74) | 0.71 (0.62–0.80) | 0.76 (0.72–0.81) |
CSS, cancer-specific survival; CI, confidence interval; ST, survival trees; RSF, random survival forests; GBM, gradient boosting machines.
Table 3 presents the C-indexes of different models for predicting 3- and 5-year CSS of gastric cancer patients in same variables group. In the 3-year CSS cohort, the C-index of Cox (C-index: 0.75, 95% CI: 0.72–0.79), RSF (C-index: 0.77, 95% CI: 0.74–0.80), and GBM (C-index: 0.75, 95% CI: 0.72–0.79) in the train dataset were greater than that of ST (C-index: 0.73, 95% CI: 0.69–0.76). In the 5-year CSS cohort, the C-index of Cox (C-index: 0.71, 95% CI: 0.67–0.74), RSF (C-index: 0.72, 95% CI: 0.70–0.75), and GBM (C-index: 0.71, 95% CI: 0.68–0.74) in the train dataset were greater than that of ST (C-index: 0.68, 95% CI: 0.65–0.71). The C-index of Cox, RSF, and GBM were greater than ST in every dataset.
Figure S6 draws the ROC curves of different models that predict the 3- and 5-year CSS of gastric cancer in the same variables group. In the train dataset of the 3-year CSS cohort, the AUC values of Cox, ST, RSF, and GBM were 0.81 (95% CI: 0.79–0.83), 0.78 (95% CI: 0.76–0.80), 0.84 (95% CI: 0.83–0.86), and 0.82 (95% CI: 0.80–0.84), respectively. In the train dataset of the 5-year CSS cohort, the AUC values of Cox, ST, RSF, and GBM were 0.73 (95% CI: 0.70–0.76), 0.70 (95% CI: 0.67–0.73), 0.78 (95% CI: 0.75–0.80), and 0.73 (95% CI: 0.71–0.76), respectively. Among the four models in every dataset, the AUC value of ST was lower than other three models.
Figure S7 plots the calibration curves of different models for predicting 3- and 5-year CSS of gastric cancer in the same variables group. Whether in 3- or 5-year CSS cohorts, the consistency of RSF and Cox were better than those of GBM and ST. The predicted value of GBM was higher than the actual value, while the predicted value of ST was lower than the actual value.
Discussion
Key findings
This study developed four models (Cox, ST, RSF and GBM) to predict the survival of gastric cancer patients. Whether in 3-year CSS cohort or 5-year CSS cohort, the C-index or AUC of Cox, RSF, and GBM were greater than ST in every dataset. The performance of calibration curves of Cox and RSF were better than those of GBM and ST in both the multivariable group and TNM group. The performance of the calibration curves of all models in the multivariable group were better than that in the TNM group. In addition, we also established a nomogram to predict the 3- and 5-year CSS of gastric cancer patients.
Explanations of findings
The proportion of stage III and IV hospitalized gastric cancer patients in the Hebei Cancer Registration Project (61.41%) was higher than that of Chinese California gastric cancer patients (55.35%). Previous studies have shown that the prognosis of patients with stage III and IV gastric cancer is much worse than that of patients with stage I and II gastric cancer (42,43). The survival rate of hospitalized gastric cancer patients in Hebei Province (1-, 3-, and 5-year CSS were 82.61%, 57.07%, and 44.48%, respectively) were higher than that of Chinese California hospitalized gastric cancer patients (1-, 3-, and 5-year CSS were 68.58%, 47.99%, and 42.91%, respectively), which may be due to Hebei Province was a high-risk area for gastric cancer and had relatively advanced gastric cancer diagnosis and treatment technologies. In addition, it may be related to treatment, tumor biology or follow-up time. Further exploration is still needed.
The C-index or AUC of Cox, RSF, and GBM were greater than ST in every dataset. The performance of calibration curves of Cox and RSF were better than those of GBM and ST. The results of this study were similar to our previous results of osteosarcoma (44). It indicated that Cox and RSF not only outperform ST and GBM in the survival prognosis of osteosarcoma, but also in the survival prognosis of gastric cancer, which may be extrapolated to other cancers in future studies. ST splitting nodes by maximizing survival differences among nodes using log-rank testing. However, the prediction error is large, resulting in low prediction accuracy (31,32). Both RSF and GBM are combined of a large number of ST. The fundamentals of GBM are training a new ST according to the negative gradient information of the loss function based on the current ST, and combining the trained newborn ST with the existing ST (34). In this study, The C-index and AUC of GBM are similar to that of Cox or RSF. However, the consistency of calibration curve of GBM performs poorer, which means it needs to be improved. RSF uses the bootstrap method to extract sub-samples from the original samples to construct a ST, averaging the cumulative risk function of each ST and ultimately obtaining the total cumulative risk function (33). RSF considers all possible connections among outcome variables and predictors, as well as all possible interactions among variables. Therefore, it approximates the data generation mechanism in observed value, obtains the predicted value that is closest to the actual value (37). RSF may be an alternative non-parametric method to Cox.
Comparison with similar researches
The survival prediction model constructed based on multiple variables was superior to that based on T stage, N stage, and M stage. This may be due to the fact that the TNM staging system only considers tumor infiltration, lymph node metastasis, and tumor metastasis, without considering biological differences among different patients. This leads to different survival outcomes for patients in the same stage, and same survival outcome for patients in different stages. Therefore, the TNM staging system is not sufficient to predict the prognosis of tumors (45-47). The results of Cox PH regression model showed that age, tumor site, T stage, N stage, M stage, and surgery situation were the influencing factors for the survival prognosis of gastric cancer patients. The survival prognosis of gastric cancer patients aged ≥75 years old is significantly worse than those aged <55 years old, which is similar to previous research results (42). This may because that compared to young patients, elderly patients have relatively poor physical condition and more basic diseases. They are prone to recurrence after receiving treatment such as surgery or chemotherapy, so the survival prognosis is poor (48-51). The survival prognosis of patients with overlapping sites were significantly worse than those with cardiac sites; the survival prognosis of patients with T3–T4 stage were significantly worse than those with T1 stage; the survival prognosis of patients with N2 stage and N3 stage were significantly worse than those with N0 stage; the survival prognosis of patients with M1 stage were significantly worse than those with M0 stage; the survival prognosis of patients who underwent surgery were better than those who didn’t undergo surgery, and these research results were consistent with previous studies (52-56). The results of this study showed that there was no statistical difference in survival prognosis between different genders, which was consistent with previous research results (57,58).
Strengths and limitations
The advantages of this study were as follow: firstly, in this study, we set hospitalized gastric cancer patients of the Hebei Cancer Registration Project as the development dataset to develop models, and used Chinese California hospitalized gastric cancer patients as the external test dataset to external validate models. This external test dataset can exclude differences between hospital source data and population data, as well as differences among different races. The external test dataset proves that our model is not only suitable for hospitalized gastric cancer patients in Hebei Province, but also for Chinese California hospitalized gastric cancer patients. Such differences between development dataset and external test dataset can serve as a valuable tool for effective external validation. At the same time, all models were established using ten-fold cross validation with 200 iterations, which further increased the reliability of the model. Secondly, in this study, we compared multivariable group with TNM group. In our study, we used a TNM group, composed of three variables (T stage, N stage, and M stage), which were more detailed and had more accurate predictive effects than TNM stage. Thirdly, we set up same variables group (Cox, ST, RSF and GBM include the same variables) for sensitivity analysis to avoid bias caused by different models include different variables. Fourthly, we use the “random forest” multiple imputation method to impute the missing data, which can reduce the information bias caused by excluding samples due to the missing of some variables. Fifthly, we developed a nomogram based on Cox PH regression model to predict the CSS rate of gastric cancer patients. By bringing various variables of the patient in nomogram, we can obtain the 3- and 5-year CSS of the patient. This prognostic tool not only allows doctors and patients to know the patients’ probability of survival, but also provides recommendations for clinical doctors’ treatment decisions and methods.
This study still has some limitations: firstly, the variables included in this study are limited. Previous studies have shown that some other variables, such as patient nutritional status (42,59), Eastern Cooperative Oncology Group (ECOG) performance (60), radiomics (61), and Helicobacter pylori infection (62), may be related to the survival of gastric cancer patients. In future research, we will attempt to incorporate these factors into the model. Secondly, due to technical reasons, we only used three tree-based machine learning methods (ST, RSF, and GBM) to compare with the Cox model.
Implications and actions needed
This study fills in the blank of prognostic models for hospitalized gastric cancer patients in Hebei Province. The proposed nomogram can be used to calculate the 3- and 5-year CSS of gastric cancer patients based on the clinical information. It may be utilized practically to help clinicians to obtain individualized survival prediction and provide better treatment allocation. In the future, we will try to compare more models [such as SVM, artificial neural networks, XGBoost (eXtreme gradient boosting), etc.] to establish a more comprehensive and excellent prognostic model.
Conclusions
The performance of the multivariable group was superior to TNM group. Cox and RSF have better predictive effects than ST and GBM. The nomogram was useful for facilitating clinicians to predict the survival of gastric cancer patients, and identifying high-risk patients so as to adopt more reasonable treatment plans.
Acknowledgments
We are very grateful to the staff in Hebei Province cancer registries for their kind work in data collection and follow up. Anyone else who contributed to the manuscript but does not qualify for authorship has been acknowledged with their permission.
Funding: None.
Footnote
Reporting Checklist: The authors have completed the TRIPOD reporting checklist. Available at https://cco.amegroups.com/article/view/10.21037/cco-23-85/rc
Data Sharing Statement: Available at https://cco.amegroups.com/article/view/10.21037/cco-23-85/dss
Peer Review File: Available at https://cco.amegroups.com/article/view/10.21037/cco-23-85/prf
Conflicts of Interest: All authors have completed the ICMJE uniform disclosure form (available at https://cco.amegroups.com/article/view/10.21037/cco-23-85/coif). The authors have no conflicts of interest to declare.
Ethical Statement: The authors are accountable for all aspects of the work in ensuring that questions related to the accuracy or integrity of any part of the work are appropriately investigated and resolved. The study was conducted in accordance with the Declaration of Helsinki (as revised in 2013). For external test dataset from the SEER database, the authors obtained authorization to access the SEER Research Data supported by the National Cancer Institute with approval number 11241-Nov2021. Because public and anonymous data from the SEER database were used, informed patient consent was not required. For development dataset from Hebei Cancer Registration Project, the Ethics Committee of the Fourth Hospital of Hebei Medical University/the Tumor Hospital of Hebei Province has confirmed that no ethical approval is required. Individual consent for this retrospective analysis was waived.
Open Access Statement: This is an Open Access article distributed in accordance with the Creative Commons Attribution-NonCommercial-NoDerivs 4.0 International License (CC BY-NC-ND 4.0), which permits the non-commercial replication and distribution of the article with the strict proviso that no changes or edits are made and the original work is properly cited (including links to both the formal publication through the relevant DOI and the license). See: https://creativecommons.org/licenses/by-nc-nd/4.0/.
References
- Sung H, Ferlay J, Siegel RL, et al. Global Cancer Statistics 2020: GLOBOCAN Estimates of Incidence and Mortality Worldwide for 36 Cancers in 185 Countries. CA Cancer J Clin 2021;71:209-49. [Crossref] [PubMed]
- Zheng R, Zhang S, Zeng H, et al. Cancer incidence and mortality in china, 2016. Journal of the National Cancer Center 2022;2:1-9. [Crossref]
- Global burden of 369 diseases and injuries in 204 countries and territories, 1990-2019: a systematic analysis for the Global Burden of Disease Study 2019. Lancet 2020;396:1204-22. [Crossref] [PubMed]
- Choi IJ, Lee JH, Kim YI, et al. Long-term outcome comparison of endoscopic resection and surgery in early gastric cancer meeting the absolute indication for endoscopic resection. Gastrointest Endosc 2015;81:333-41.e1. [Crossref] [PubMed]
- Pyo JH, Lee H, Min BH, et al. Long-Term Outcome of Endoscopic Resection vs. Surgery for Early Gastric Cancer: A Non-inferiority-Matched Cohort Study. Am J Gastroenterol 2016;111:240-9. [Crossref] [PubMed]
- Washington K. 7th edition of the AJCC cancer staging manual: stomach. Ann Surg Oncol 2010;17:3077-9.
- Li X, Zhai Z, Ding W, et al. An artificial intelligence model to predict survival and chemotherapy benefits for gastric cancer patients after gastrectomy development and validation in international multicenter cohorts. Int J Surg 2022;105:106889. [Crossref] [PubMed]
- Woo Y, Son T, Song K, et al. A Novel Prediction Model of Prognosis After Gastrectomy for Gastric Carcinoma: Development and Validation Using Asian Databases. Ann Surg 2016;264:114-20. [Crossref] [PubMed]
- Zu H, Wang F, Ma Y, et al. Stage-stratified analysis of prognostic significance of tumor size in patients with gastric cancer. PLoS One 2013;8:e54502. [Crossref] [PubMed]
- Li Z, Wu X, Gao X, et al. Development and validation of an artificial neural network prognostic model after gastrectomy for gastric carcinoma: An international multicenter cohort study. Cancer Med 2020;9:6205-15. [Crossref] [PubMed]
- Peeters A, Barendregt JJ, Willekens F, et al. Obesity in adulthood and its consequences for life expectancy: a life-table analysis. Ann Intern Med 2003;138:24-32. [Crossref] [PubMed]
- Tian FX, Cai YQ, Zhuang LP, et al. Clinicopathological features and prognosis of patients with gastric neuroendocrine tumors: A population-based study. Cancer Med 2018;7:5359-69. [Crossref] [PubMed]
- Liu J, Geng Q, Liu Z, et al. Development and external validation of a prognostic nomogram for gastric cancer using the national cancer registry. Oncotarget 2016;7:35853-64. [Crossref] [PubMed]
- Yu C, Zhang Y. Development and validation of prognostic nomogram for young patients with gastric cancer. Ann Transl Med 2019;7:641. [Crossref] [PubMed]
- Wang CY, Yang J, Zi H, et al. Nomogram for predicting the survival of gastric adenocarcinoma patients who receive surgery and chemotherapy. BMC Cancer 2020;20:10. [Crossref] [PubMed]
- Haga Y, Ikejiri K, Wada Y, et al. Preliminary study of surgical audit for overall survival following gastric cancer resection. Gastric Cancer 2015;18:138-46. [Crossref] [PubMed]
- Li J, Lin Y, Wang Y, et al. Prognostic nomogram based on the metastatic lymph node ratio for gastric neuroendocrine tumour: SEER database analysis. ESMO Open 2020;5:e000632. [Crossref] [PubMed]
- Peng W, Ma T, Xu H, et al. Survival benefits of palliative gastrectomy in stage IV gastric cancer: a propensity score matched analysis. J Gastrointest Oncol 2020;11:376-85. [Crossref] [PubMed]
- Weng SF, Reps J, Kai J, et al. Can machine-learning improve cardiovascular risk prediction using routine clinical data? PLoS One 2017;12:e0174944. [Crossref] [PubMed]
- Ji GW, Jiao CY, Xu ZG, et al. Development and validation of a gradient boosting machine to predict prognosis after liver resection for intrahepatic cholangiocarcinoma. BMC Cancer 2022;22:258. [Crossref] [PubMed]
- Bibault JE, Chang DT, Xing L. Development and validation of a model to predict survival in colorectal cancer using a gradient-boosted machine. Gut 2021;70:884-9. [Crossref] [PubMed]
- Ben Azzouz F, Michel B, Lasla H, et al. Development of an absolute assignment predictor for triple-negative breast cancer subtyping using machine learning approaches. Comput Biol Med 2021;129:104171. [Crossref] [PubMed]
- Parikh RB, Manz C, Chivers C, et al. Machine Learning Approaches to Predict 6-Month Mortality Among Patients With Cancer. JAMA Netw Open 2019;2:e1915997. [Crossref] [PubMed]
- D'Ascenzo F, De Filippo O, Gallone G, et al. Machine learning-based prediction of adverse events following an acute coronary syndrome (PRAISE): a modelling study of pooled datasets. Lancet 2021;397:199-207. [Crossref] [PubMed]
- Li W, Liu Y, Liu W, et al. Machine Learning-Based Prediction of Lymph Node Metastasis Among Osteosarcoma Patients. Front Oncol 2022;12:797103. [Crossref] [PubMed]
- Kaida H, Kitajima K, Nakajo M, et al. Predicting tumor response and prognosis to neoadjuvant chemotherapy in esophageal squamous cell carcinoma patients using PERCIST: a multicenter study in Japan. Eur J Nucl Med Mol Imaging 2021;48:3666-82. [Crossref] [PubMed]
- Ayaru L, Ypsilantis PP, Nanapragasam A, et al. Prediction of Outcome in Acute Lower Gastrointestinal Bleeding Using Gradient Boosting. PLoS One 2015;10:e0132485. [Crossref] [PubMed]
- Sawada S, Yamashita N, Suehisa H, et al. Risk factors for recurrence after lung cancer resection as estimated using the survival tree method. Chest 2013;144:1238-44. [Crossref] [PubMed]
- Hines RB, Jiban MJH, Specogna AV, et al. The association between post-treatment surveillance testing and survival in stage II and III colon cancer patients: An observational comparative effectiveness study. BMC Cancer 2019;19:418. [Crossref] [PubMed]
- Liu WC, Li MX, Wu SN, et al. Using Machine Learning Methods to Predict Bone Metastases in Breast Infiltrating Ductal Carcinoma Patients. Front Public Health 2022;10:922510. [Crossref] [PubMed]
- Shimokawa A, Kawasaki Y, Miyaoka E. Comparison of splitting methods on survival tree. Int J Biostat 2015;11:175-88. [Crossref] [PubMed]
- Davis RB, Anderson JR. Exponential survival trees. Stat Med 1989;8:947-61. [Crossref] [PubMed]
- Ishwaran H, Kogalur UB, Blackstone EH, et al. Random survival forests. Ann Appl Stat 2008;2:841-60. [Crossref]
- Natekin A, Knoll A. Gradient boosting machines, a tutorial. Front Neurorobot 2013;7:21. [Crossref] [PubMed]
- Qiu X, Gao J, Yang J, et al. A Comparison Study of Machine Learning (Random Survival Forest) and Classic Statistic (Cox Proportional Hazards) for Predicting Progression in High-Grade Glioma after Proton and Carbon Ion Radiotherapy. Front Oncol 2020;10:551420. [Crossref] [PubMed]
- Lin H, Zeng L, Yang J, et al. A Machine Learning-Based Model to Predict Survival After Transarterial Chemoembolization for BCLC Stage B Hepatocellular Carcinoma. Front Oncol 2021;11:608260. [Crossref] [PubMed]
- Du M, Haag DG, Lynch JW, et al. Comparison of the Tree-Based Machine Learning Algorithms to Cox Regression in Predicting the Survival of Oral and Pharyngeal Cancers: Analyses Based on SEER Database. Cancers (Basel) 2020;12:2802. [Crossref] [PubMed]
- van Zutphen M, van Duijnhoven FJB, Wesselink E, et al. Identification of Lifestyle Behaviors Associated with Recurrence and Survival in Colorectal Cancer Patients Using Random Survival Forests. Cancers (Basel) 2021;13:2442. [Crossref] [PubMed]
- Banerjee M, Reyes-Gastelum D, Haymart MR. Treatment-Free Survival in Patients With Differentiated Thyroid Cancer. J Clin Endocrinol Metab 2018;103:2720-7. [Crossref] [PubMed]
- Banerjee M, Muenz DG, Chang JT, et al. Tree-based model for thyroid cancer prognostication. J Clin Endocrinol Metab 2014;99:3737-45. [Crossref] [PubMed]
- Samara KA, Al Aghbari Z, Abusafia A. GLIMPSE: a glioblastoma prognostication model using ensemble learning-a surveillance, epidemiology, and end results study. Health Inf Sci Syst 2021;9:5. [Crossref] [PubMed]
- Park KB, Jun KH, Song KY, et al. Development of a staging system and survival prediction model for advanced gastric cancer patients without adjuvant treatment after curative gastrectomy: A retrospective multicenter cohort study. Int J Surg 2022;101:106629. [Crossref] [PubMed]
- Gronnier C. Feature Review Papers on Gastroesophageal Junction and Gastric Cancers. Cancers (Basel) 2022;14:3979. [Crossref] [PubMed]
- Hao Y, Liang D, Zhang S, et al. Machine learning for predicting the survival in osteosarcoma patients: Analysis based on American and Hebei Province cohort. Biomol Biomed 2023;23:883-93. [Crossref] [PubMed]
- Mita MT, Marchesi F, Cecchini S, et al. Prognostic assessment of gastric cancer: retrospective analysis of two decades. Acta Biomed 2016;87:205-11. [PubMed]
- Jiao XG, Deng JY, Zhang RP, et al. Prognostic value of number of examined lymph nodes in patients with node-negative gastric cancer. World J Gastroenterol 2014;20:3640-8. [Crossref] [PubMed]
- Nakauchi M, Court CM, Tang LH, et al. Validation of the Memorial Sloan Kettering Gastric Cancer Post-Resection Survival Nomogram: Does It Stand the Test of Time? J Am Coll Surg 2022;235:294-304. [Crossref] [PubMed]
- Puhr HC, Karner A, Taghizadeh H, et al. Clinical characteristics and comparison of the outcome in young versus older patients with upper gastrointestinal carcinoma. J Cancer Res Clin Oncol 2020;146:3313-22. [Crossref] [PubMed]
- Takatsu Y, Hiki N, Nunobe S, et al. Clinicopathological features of gastric cancer in young patients. Gastric Cancer 2016;19:472-8. [Crossref] [PubMed]
- Schildberg CW, Croner R, Schellerer V, et al. Differences in the treatment of young gastric cancer patients: patients under 50 years have better 5-year survival than older patients. Adv Med Sci 2012;57:259-65. [Crossref] [PubMed]
- Ueno D, Matsumoto H, Kubota H, et al. Prognostic factors for gastrectomy in elderly patients with gastric cancer. World J Surg Oncol 2017;15:59. [Crossref] [PubMed]
- Noh SH, Park SR, Yang HK, et al. Adjuvant capecitabine plus oxaliplatin for gastric cancer after D2 gastrectomy (CLASSIC): 5-year follow-up of an open-label, randomised phase 3 trial. Lancet Oncol 2014;15:1389-96. [Crossref] [PubMed]
- Claassen YHM, van Amelsfoort RM, Hartgrink HH, et al. Effect of Hospital Volume With Respect to Performing Gastric Cancer Resection on Recurrence and Survival: Results From the CRITICS Trial. Ann Surg 2019;270:1096-102. [Crossref] [PubMed]
- Ebinger SM, Warschkow R, Tarantino I, et al. Modest overall survival improvements from 1998 to 2009 in metastatic gastric cancer patients: a population-based SEER analysis. Gastric Cancer 2016;19:723-34. [Crossref] [PubMed]
- Adham D, Abbasgholizadeh N, Abazari M. Prognostic Factors for Survival in Patients with Gastric Cancer using a Random Survival Forest. Asian Pac J Cancer Prev 2017;18:129-34. [PubMed]
- He X, Lai S, Su T, et al. Survival benefits of gastrectomy in gastric cancer patients with stage IV: a population-based study. Oncotarget 2017;8:106577-86. [Crossref] [PubMed]
- Li X, Wang W, Ruan C, et al. Age-specific impact on the survival of gastric cancer patients with distant metastasis: an analysis of SEER database. Oncotarget 2017;8:97090-100. [Crossref] [PubMed]
- Karapetyan L, Wang L, Gardiner J, et al. Ethnic, racial and gender differences in presentation and outcomes of gastric cancer in a Michigan cohort. J Clin Oncol 2018;36:e18656. [Crossref]
- Sugawara K, Yamashita H, Urabe M, et al. Combining nutritional status with TNM stage: a physiological update on gastric cancer staging for improving prognostic accuracy in elderly patients. Int J Clin Oncol 2022;27:1849-58. [Crossref] [PubMed]
- Yuan SQ, Nie RC, Chen YM, et al. Glasgow Prognostic Score is superior to ECOG PS as a prognostic factor in patients with gastric cancer with peritoneal seeding. Oncol Lett 2018;15:4193-200. [Crossref] [PubMed]
- Shi S, Miao Z, Zhou Y, et al. Radiomics signature for predicting postoperative disease-free survival of patients with gastric cancer: development and validation of a predictive nomogram. Diagn Interv Radiol 2022;28:441-9. [Crossref] [PubMed]
- Jia Z, Zheng M, Jiang J, et al. Positive H. pylori status predicts better prognosis of non-cardiac gastric cancer patients: results from cohort study and meta-analysis. BMC Cancer 2022;22:155. [Crossref] [PubMed]