Bioinformatics identification of characteristic genes of cervical cancer via an artificial neural network
Highlight box
Key findings
• Artificial neural network (ANN) is a robust neural network model that can be used to potentially predict cervical cancer (CC), which can provide novel insights into the pathogenesis and molecular mechanisms of CC. The present hypothesis-free study sought to use an ANN to identify the characteristic genes of CC.
What is known and what is new?
• There are differences in the immune infiltrating cells between the normal cervical and CC tissues.
• The differentially expressed genes of RNA sequencing profiles were identified between the normal and CC tissues. A neural network model was established using the characteristic genes of CC. A Cox regression analysis was used to analyze the experimental data and to examine the verification accuracy of the model. The differences in the immune infiltrating cells between the normal cervical and CC tissues were compared by CIBERSORT.
What is the implication, and what should change now?
• ANN is generalizable to a particular model and can thus provide accurate responses to a new learning experience, which also provides novel insights into the pathogenesis and molecular mechanisms of CC. More further studies are necessary to explore the roles of tumor-infiltrating immune cells and antigen presenting cells as diagnostic and prognostic biomarkers of CC combine with ANN.
Introduction
Cervical cancer (CC) is the fourth most common type of malignancy among females with a high mortality rate worldwide (1). Approximately 95% of CC is caused by a persistent oncogenic human papillomavirus (HPV) infection (2,3), while there is still debate about the precise molecular pathways between chronic high-risk HPV infection and the CC pathological phase (4).
Artificial intelligence (AI) techniques have been rapidly expanding in various scientific fields, including medical science (5). An artificial neural network (ANN) is a computational model using machine-learning algorithms to understand complex systems. It contains the following three layers: the input layer, output layer and hidden layer. Each layer is made up of a set of neurons that are connected to each other in the three layers (6,7). ANNs have been used to decrease the majority of problems associated with conventional statistical methods. In fact, ANNs do not require the assumption of data normality and can determine a functional relationship in which the relationship between the independent and dependent variables is not necessarily linear (8). Moreover, since ANN has no limitation regarding its formulated function, it is more flexible and has more strength in mimicking complicated patterns than logistic regression. Another advantage of ANNs is that their ability to find patterns despite missing data (9). Thus, a correct answer may still be obtained if certain cells are removed or exhibit a false function within the network (10). However, using the classical models require many assumptions that may not be true in some real applications. Violation of these assumptions may produce error in prediction and hypothesis testing. The inability to capture pattern complexity and inability to capture process dynamic are two major pitfalls of traditional methods (9). Additionally, ANNs are readily generalizable to a particular model and can thus provide accurate responses to a new, untrained learning experience (9,10). ANNs had been reported as an intelligent method with relatively good sensitivity and specificity in identifying the normal and precancerous tissues, which also had been reported as a predictive model in assessing the risk of cancer recurrence (5,11). The ANN represents a highly potent technology, which is a robust neural network model based on the gene score.
Bioinformatics analysis has been a powerful method in the study of disease, which also can provide insights into the pathogenesis and molecular mechanism of cancer (12,13). Growing research has shown multiple genes is convoluted in the pathogenesis of CC (14,15). Recently, a large number of differentially expressed genes (DEGs) have been discovered, which can help identify potential target proteins and agents for the treatment in CC patients (16-18). The available data on some messenger RNAs are dissimilar or even contradictory to date. Nowadays, the immune system plays an essential role in controlling virus infection which provides possible options for the immunotherapy of CC patients. Tumor-infiltrating immune cells (TIICs) are an important determinant of the tumor microenvironment and are associated with cancer progression and treatment response (19). To date, many methods have been used to predict TIICs, including single-sample gene set enrichment analyses, CIBERSORT (20), TIMER2.0 (21), EPIC (22), ABIS-sequencing (23), microarray microdissections with analyses of differences (24), and digital sorting algorithms.
In this study, CIBERSORT was used to identify the immune cells of CC. DEGs were screened from the Gene Expression Omnibus (GEO) database (https://www.ncbi.nlm.nih.gov/geo/). R software was used to identify the DEGs, and Metascape (http://metascape.org/gp/index.html) was used to analyze the functions, pathways, and biological network of the DEGs. The ANN method was applied to establish a neural network model based on the characteristic genes of CC, which was examined by receiver operating characteristic (ROC) analysis. Besides, the enumeration of the TIICs was also analyzed, which can provide potential treatment prescription. A flowchart of the analysis is displayed in Figure 1. Each step is further elaborated on in the following section. We present this article in accordance with the STREGA reporting checklist (available at https://cco.amegroups.com/article/view/10.21037/cco-23-139/rc).
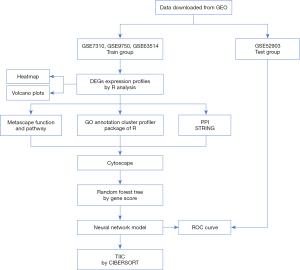
Methods
Data acquisition and preprocessing
The GEO is a publicly available repository for data generated from high-throughput microarray experiments. The gene expression profiles of CC (i.e., the GSE7410, GSE9750, GSE63514 and GSE63514, and GSE52903 datasets) were retrieved from the GEO database. A total of 227 specimens, 156 CC and 71 normal cervical tissues, were included in the study. The gene expression profiles of the GSE7410, GSE9750 and GSE63514 datasets were tested in the train group, and those of the GSE52903 dataset in the test group. The study was conducted in accordance with the Declaration of Helsinki (as revised in 2013).
Identification of DEGs
Expression datasets of the training group were integrated by R software to identify the common DEGs. Normalization and log2 transformation were conducted for each dataset, heatmaps and volcano plots were used to analyze the DEGs. The following filter conditions were set: |log2 fold-change| ≥1 and an adjusted P value <0.05.
Functions, pathways, and biological network of the DEGs
Metascape was used to conduct the function and pathway enrichment analyses of the DEGs. Gene Ontology (GO) and Kyoto Encyclopedia of Genes and Genomes (KEGG) analyses were performed using the clusterProfiler package in R software. The cut-off criterion was an adjusted P value of <0.05. The GO annotation consists of the following 3 subontologies revealing the biological characteristics of the target genes in all organisms: molecular functions (MFs), cellular components (CCs), and biological processes (BPs). Afterwards, a protein-protein interaction (PPI) network of the genes with a score ≥0.4 was built using the online Search Tool to Retrieve Interacting Genes (STRING), and then visualized by Cytoscape (version 3.8.2). In co-representation networks, the maximal clique center (MCC) algorithm is considered the most efficient algorithm to identify the central nodes. The MCC of each node was determined by the CytoHubba, a Cytoscape plug-in. The top 10 genes with the highest MCC values were regarded as the hub genes.
Statistical analysis
Neural network model establishment and verification
An ANN analysis was conducted to obtain the random-forest tree. Gene filtering was conducted, the number of X was calculated and the weighted importance of the genes was examined. A gene importance map was drawn to highlight the characteristic genes of CC and the expression levels of such significant genes. A specific gene neural network model was established based on the input layer characteristic gene score, hidden layer node weight score, and output layer weight genus. The vertical and horizontal axes of a ROC curve define the true- and false-positive rates, respectively. The area under the curve (AUC) of the ROC curve was used to evaluate the accuracy of the model. The ROC curve describes the specificity and sensitivity of the classifier. The closer to 1 the AUC, the higher quality of the classifier. The ideal classifier AUC is 1, and AUC =0.5 means it is random and useless (9,25).
Analysis of the TIICs in CC
To clarify the number of immune cells in each sample, 22 types of TIICs were analyzed by CIBERSORT. The proportion matrix of the TIICs in CC was obtained by the CIBERSORT.R package using the default signature matrix at 100 permutations (23). The CIBERSORT P value was set at <0.05. An immune cell difference analysis was conducted by comparing gene scores and reducing the batch difference between the two groups. The accuracy of the model was predicted, and the correlation between the immune cells and CC was analyzed.
Results
Identification of the DEGs
In this study, expression datasets of 10,360 genes in normal tissues (n=53) and CC tissues (n=105) were selected for the identification of the DEGs. Among them, 107 DEGs were co-expressed, of which 35 were upregulated and 72 were downregulated. The heatmap is shown in Figure 2A. Genes with a fold change of >2 were submitted to further analysis. The corresponding volcano plots are shown in Figure 2B.
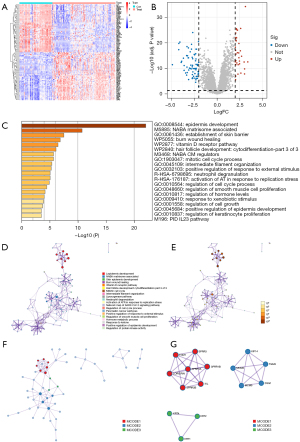
Function and pathway enrichment analyses
The gene set and pathway enrichment analyses were conducted using the following ontology sources: GO BPs, KEGG pathways, Canonical pathways, Reactome gene sets, cell-type signatures, TRRUST, CORUM, PaGenBase, DisGeNET, PANTHER pathways, WikiPathways, COVID, and transcription factor targets. Terms with an enrichment factor, minimum count, and P value of >1.5, 3, and <0.01, respectively, were obtained and grouped in a cluster according to their membership similarity. The q value was determined using the Benjamini-Hochberg method (8), while the P value was determined according to the cumulative hypergeometric distribution (7).
Kappa scores (10) were employed as the similarity metric for the hierarchical clustering of the enriched terms. The sub-trees were classified as a cluster based on a similarity score of >0.3. Each cluster was indicated by its most significantly enriched term. The results are shown in Figure 2C and Table 1. To further assess the correlations among the enriched terms, a group of enriched terms was used to plot a network graph. The terms with a similarity score of >0.3 were linked by an edge. Cytoscape (9) was used to visualize the network. Each node indicated a term, which was colored by its cluster identification number (Figure 2D) and P value (Figure 2E). Functional and pathway enrichment analyses were independently conducted for each MCODE component, and the 3 best scoring terms (as screened by the P value) were used to describe the function of each component (Figure 2F,2G). The pathway and process enrichment analysis results are set out in Tables 2,3.
Table 1
GO | Category | Description | Count | % | Log10(P) | Log10(q) |
---|---|---|---|---|---|---|
GO:0008544 | GO biological processes | Epidermis development | 22 | 20.56 | −22.05 | −17.70 |
M5885 | Canonical pathways | NABA matrisome-associated | 19 | 17.76 | −10.73 | −7.39 |
GO:0061436 | GO biological processes | Establishment of skin barrier | 5 | 4.67 | −7.50 | −4.23 |
WP5055 | WikiPathways | Burn wound healing | 7 | 6.54 | −6.87 | −3.75 |
WP2877 | WikiPathways | Vitamin D receptor pathway | 8 | 7.48 | −6.46 | −3.41 |
WP2840 | WikiPathways | Hair follicle development: cytodifferentiation-part 3 of 3 | 6 | 5.61 | −6.10 | −3.08 |
M3468 | Canonical pathways | NBAA ECM regulators | 8 | 7.48 | −5.66 | −2.69 |
GO:1903047 | GO biological processes | Mitotic cell cycle process | 11 | 10.28 | −5.65 | −2.69 |
GO:0045109 | GO biological processes | Intermediate filament organization | 5 | 4.67 | −5.35 | −2.40 |
GO:0032103 | GO biological processes | Positive regulation of response to external stimulus | 10 | 9.35 | −5.28 | −2.35 |
R-HSA-6798695 | Reactome gene sets | Neutrophil degranulation | 10 | 9.35 | −5.06 | −2.19 |
R-HSA-176187 | Reactome gene sets | Activation of ATR in response to replication stress | 4 | 3.74 | −5.05 | −2.19 |
GO:0010564 | GO biological processes | Regulation of the cell cycle process | 12 | 11.21 | −4.93 | −2.13 |
GO:0048660 | GO biological processes | Regulation of smooth muscle cell proliferation | 6 | 5.61 | −4.47 | −1.79 |
GO:0010817 | GO biological processes | Regulation of hormone levels | 9 | 8.41 | −4.14 | −1.53 |
GO:0009410 | GO biological processes | Response to xenobiotic stimulus | 8 | 7.48 | −4.03 | −1.46 |
GO:0001558 | GO biological processes | Regulation of cell growth | 8 | 7.48 | −3.90 | −1.38 |
GO:0045684 | GO biological processes | Positive regulation of epidermis development | 3 | 2.80 | −3.70 | −1.25 |
GO:0010837 | GO biological processes | Regulation of keratinocyte proliferation | 3 | 2.80 | −3.51 | −1.09 |
M196 | Canonical pathways | PID IL23 pathway | 3 | 2.80 | −3.51 | −1.09 |
“Count” is the number of genes in the user-provided lists with membership in the given ontology term. “%” is the percentage of all of the user-provided genes that are found in the given ontology term (only input genes with at least 1 ontology term annotation are included in the calculation). “Log10(P)” is the P value in log base 10. “Log10(q)” is the multi-test adjusted p value in log base 10. GO, Gene Ontology; ECM, extracellular matrix; ATR, ataxia telangiectasia-mutated and Rad3-related; PID, process ID.
Table 2
GO | Description | Log10(P) |
---|---|---|
GO:0018149 | Peptide cross-linking | −21.6 |
R-HSA-6809371 | Formation of the cornified envelope | −20.6 |
GO:0008544 | Epidermis development | −18.5 |
The three best-scoring terms by P values were retained as the functional description of the corresponding components, shown in the tables underneath the corresponding network plots in Figure 2. GO, Gene Ontology.
Table 3
Color | MCODE | GO | Description | Log10(P) |
---|---|---|---|---|
Red | MCODE_1 | GO:0031424 | Keratinization | −18.0 |
GO:0018149 | Peptide cross-linking | −16.9 | ||
R-HSA-6809371 | Formation of the cornified envelope | −16.6 | ||
Green | MCODE_3 | GO:0045109 | Intermediate filament organization | −8.0 |
GO:0031424 | Keratinization | −7.7 | ||
GO:0045104 | Intermediate filament cytoskeleton organization | −7.6 |
MCODE, Molecular Complex Detection; GO, Gene Ontology.
GO and KEGG analyses
To investigate the mechanisms in the CC microenvironment, we performed GO and KEGG analyses, PPI network analyses and an immune infiltration correlation analysis. The GO analysis results of the DEGs are shown in Figure 3A-3D. The DEGs were most enriched in epidermis and skin development, and epidermal cell and keratinocyte differentiation. The KEGG analysis results of the DEGs are shown in Figure 3E-3H. The DEGs were significantly enriched in multiple pathways, such as the cell cycle (4 proteins), viral protein interaction with cytokine and cytokine receptor (5 proteins), and cytokine-cytokine receptor interaction (7 proteins). The results of the functional analysis of the characteristic CC genes are shown in Table 4.
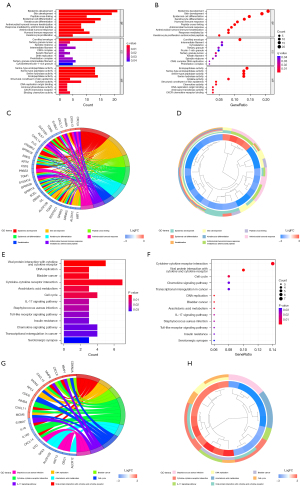
Table 4
Gene | Function |
---|---|
CDKN2A | This gene is frequently mutated or deleted in a wide variety of tumors and is known to be an important tumor suppressor gene |
C1orf112 | This gene has also been shown to have altered levels of expression in some tumors with mutant TP53, which with DNA replication and reveal possible links to DNA damage repair pathways |
HELLS | This gene encodes a lymphoid-specific helicase. Other helicases function in processes involving DNA strand separation, including replication, repair, recombination, and transcription |
MCM5 | The protein encoded by this gene is a member of the MCM family of chromatin-binding proteins and can interact with at least 2 other members of this family. The encoded protein is upregulated in the transition from the G0 to G1/S phase of the cell cycle and may actively participate in cell cycle regulation |
MCM2 | The protein encoded by this gene is one of the highly conserved MCM proteins that are involved in the initiation of eukaryotic genome replication |
KNTC1 | This gene encodes a protein that is one of many involved in mechanisms to ensure proper chromosome segregation during cell division. Experimental evidence indicates that the encoded protein functions in a similar manner to that of the Drosophila rough deal protein |
CRISP3 | This gene encodes a member of the CRISP family within the CRISP, antigen 5 and pathogenesis-related 1 protein superfamily |
PHYHIP | This gene enables protein tyrosine kinase binding activity, is involved in protein localization, and is located in the cytoplasm |
CRNN | This gene encodes a member of the “fused gene” family of proteins, which contain N-terminus EF-hand domains and multiple tandem peptide repeats, which is also known as squamous epithelial heat shock protein 53, and may play a role in the mucosal/epithelial immune response and epidermal differentiation |
MCM, mini-chromosome maintenance; CRISP, cysteine-rich secretory protein; EF, α-helix E and α-helix F.
PPI network analysis
A PPI analysis was performed by STRING (16), BioGrid (17), OmniPath (2), and InWeb_IM databases (3). All the physical interactions with a STRING score >0.132 and BioGrid were considered a reliable subset. The established network comprised a subset of proteins that interact physically with other proteins. Molecular Complex Detection (MCODE) algorithms (19) were used to evaluate the densely connected network components when the networks contained 3–500 proteins. The MCODE networks are shown in Figure 4.
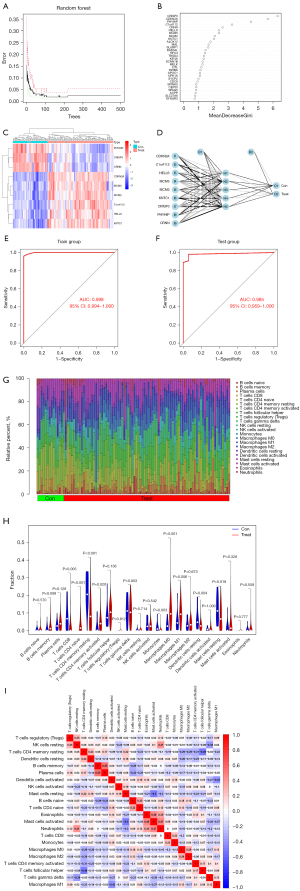
Neural network model of CC
The random-forest tree was obtained by the ANN analysis. The point with the smallest error of two groups is shown in Figure 4A. The disease characteristic genes with an importance score >2 were then selected, including cyclin-dependent kinase inhibitor 2A (CDKN2A), chromosome 1 open reading frame 112 (C1orf112), helicase, lymphoid-specific (HELLS), mini-chromosome maintenance protein 5 (MCM5), mini-chromosome maintenance protein 2 (MCM2), kinetochore associated 1 (KNTC1), cysteine-rich secretory protein 3 (CRISP3), phytanoyl-CoA 2-hydroxylase interacting protein (PHYHIP), and cornulin (CRNN) (Figure 4B). The heatmap showed that PHYHIP, CRISP3, and CRNN were upregulated in the normal tissues, but downregulated in the CC tissues, while CDKN2A, MCM5, MCM2, C1orf112, HELLS, and KNTC1 showed the opposite trends (Figure 4C). A neural network model was constructed to predict the characteristic CC genes (Figure 4D).
Verification of the neural network model
The AUC of the training group was 0.998, while that of the test group was 0.985. The higher the AUC value, the higher the prediction accuracy of the neural network model (Figure 4E,4F).
Enumeration of the TIICs
CIBERSORT was employed to assess the differences in the TIICs between the 71 normal tissues and 156 CC tissues. The proportions of the 22 immune cells are summarized in Figure 4G. The proportions of M0 and M1 macrophages were higher in the CC tissues than the normal tissues. The correlations among the 22 types of TIICs are shown in Figure 4H. Notably, the resting mast cells were negatively correlated with the activated mast cells (r=0.5), while the memory B cells were positively correlated with the plasma cells (r=0.55). There were 9 differentially expressed immune-infiltrating cells between the CC and normal groups (Figure 4I).
Discussion
With more than 265,700 deaths annually, CC is the second leading cause of death among women. More accurate treatments, higher survival rates, and a better quality of life can be achieved with the early detection of CC. At present, high-throughput sequencing and microarray technologies are commonly used to identify DEGs to predict the diagnosis and prognosis of CC patients. However, the reliability of single studies with small sample sizes is limited. Thus, this study was performed to analyze expression datasets of the DEGs in CC using bioinformatics tools and R software.
A series of genetic alterations plays an essential role in transition to malignancy and cancer progression. To determine the genetic alterations occurring during CC progression, gene expression profiles were retrieved from the GSE7410, GSE9750, GSE63514 and GSE52903 datasets. In total, 107 DEGs were identified between the normal and CC tissues, of which 35 were upregulated and 72 were downregulated. The GSE52903 dataset was used as the test group, while the GSE7410, GSE9750 and GSE63514 datasets were used as the training group. The functional analysis demonstrated that these DEGs were consistent with previous findings. Notably, microtubule binding and the cell cycle is associated with CC (26), while the cell cycle regulates the abnormal proliferation of various cancer cells (27,28).
Metascape identified the top 20 GO clusters, of which the top 4 clusters were GO:0008544, M5885, GO:0010564, and GO:1903047, which were mainly involved in epidermis development, the regulation of cell cycle process, NABA matrisome-associated and the mitotic cell cycle process. CDKN2A, C1orf112, HELLS, MCM5, MCM2, KNTC1, CRISP3, PHYHIP and CRNN were the characteristic genes of CC. The CDKN2A gene (also called the P16 gene) encodes multiple tumor suppressor 1 (29). The phosphorylation of the RB protein can be prevented by its kinase activity complex. After the dephosphorylation of the RB protein, the G1/S transition is blocked, and the cells are regulated. The CDKN2A gene, being 8.5 kb in length, located on chromosome 9p21, comprises 3 exons and encodes a protein containing 148 amino acid residues (30). CDKN2A plays a vital role in regulating the prognosis of many cancers. The CDKN2A methylation rate is significantly higher in patients with pancreatic cancer and is associated with patient survival.
C1orf112, HELLS, and MCM2 play crucial roles in the cell cycle, especially in DNA replication. We found that the BPs involved in the cell cycle transition and DNA replication were mainly enriched in the CC tissues. A subset of DENT that controls multiple DNA replication stages were upregulated in the CC tissues to maintain a hyperproliferative state. C1ORF112 has been reported to be differentially expressed in some tumors with the TP53 mutation (31). C1ORF112 is also associated with DNA replication via the regulation of the DNA repair pathways (32).
As a component of the MCM2–7 complex (an MCM complex), MCM2 is largely localized in the nuclei of eukaryotic cells (33). MCM2 overexpression is commonly found in patients with CC, especially those with persistent HPV infection (34). MCM2 promotes tumor proliferation by regulating DNA initiation, replication and elongation (35). In this study, in accordance with previous findings (35,36), MCM2 was observed to be overexpressed in the CC tissues. MCM2 might exert protective effects on CC progression. Another study showed that the cytoplasmic accumulation of MCM2 is associated with the better survival of ovarian clear cell carcinoma patients (37) and DNA damage-induced apoptosis (38). However, further studies need to be conducted to explore the functional role of this pathway in CC.
MCM5 is another member of the MCM family that participates in the initiation of DNA replication (39). MCM5 could serve as a potential biomarker to predict both CC and cervical preinvasive neoplasia (40). Additionally, CRISP3 has been found to be overexpressed in patients with intraductal carcinoma of the prostate and is related to poor outcomes (41). PHYHIP is located on the p-arm of chromosome 8. Reports have shown that PHYHIP is downregulated in breast cancer patients and the corresponding cell lines, which may provide protection against breast cancer (42,43) The Crnn protein, a member of the S100 protein, has a calcium binding site at the N-terminal (44), and can inhibit G1/S transformation in the cell cycle by upregulating the expression of the 2 proteins (45). Another study showed that Crnn is a member of the fusion gene, which may be related to the epidermal differentiation (44).
Co-expressed genes are a subset of genes with similar expression patterns that usually participate in the same BP. From the PPI network, we observed that the network encompassed 3 clusters. SPRR1A, SPRR1B, SPRR2B, SPRR3, IVL, TGM1, and LORICRIN were the MCODE1 which was associated with the formation of the cornified envelope. MCM2, MCM5, DSG1, TGM3, and KIF14 were the MCODE2. KRT1, KRT2, and KRT4 were the MCODE3 and it was associated with the organization of the intermediate filament cytoskeleton.
Immune checkpoint therapy is a kind of treatment method that improves antitumor immune responses by modulating T cell activity (46). The preliminary results for immunotherapy are encouraging, however, the overall response rate is only 17–27%, costs are high, and immune-related toxicity may occur (47). The complexity of TIICs can affect the biological behavior and immune status of the host, thereby regulating the immunotherapy response (48). Thus, research on novel biological markers to predict immunotherapy outcome is of crucial importance.
The tumor microenvironment of HPV-related CC is closely associated with its etiology (49). As a result of persistent viral infection, CC masses are infiltrated by different inflammatory immune cells, thus facilitating self-sustaining carcinogenesis (50). Previous reports have indicated that the proportion of CD4+ T cells is decreased and the CD4/CD8 ratio is reversed in the CC microenvironment suggesting a reduction in antitumor immunity (49,51). Additionally, the infiltration of myeloid cells can induce cancer progression and malignant transformation and it has been detected in high-grade cervical intraepithelial neoplasia (52). Furthermore, CC cells can recruit antigen presenting cells to generate a dysregulated immune milieu, thus facilitating tumor survival (53).
The proportions of M0 and M1 macrophages were higher in the CC tissues than the normal tissues. The results demonstrated that the resting mast cells were negatively correlated with the activated mast cells (r=0.5), while the memory B cells were positively associated with the plasma cells (r=0.55). The results showed that CD4 naive T cells, CD4 memory T cells (resting), CD4 memory T cells (activated), CD8 T cells, M0 macrophages, M1 macrophages, monocytes, mast cells (resting), and dendritic cells (resting) were differentially expressed between the CC and normal tissues. CD4 naive T cells, CD4 memory T cells (activated), M0 macrophages, and M1 macrophages were significantly increased in the CC tissues, while CD4 memory T cells (resting), CD8 T cells, monocytes, mast cells (resting), and dendritic cells (resting) were significantly decreased in the tumor tissues.
Previous research has suggested the occurrence of an immunosuppressive microenvironment in CC (54). However, the prospect of TIICs and the immune-related antigens responsible for the prognosis of CC remain largely unclear. Thus, it is necessary to explore the roles of TIICs and antigen presenting cells as diagnostic and prognostic biomarkers of CC (55).
Conclusions
The current work used a comprehensive bioinformatics analysis to identify the biomarkers of CC by using ANNs. We identified a total of 10,360 DEGs with 35 upregulated and 72 downregulated genes. Metascape was used to conduct the function and pathway enrichment analyses of the DEGs. GO and KEGG revealed the biological characteristics of the target genes. PPI established a network comprising a subset of proteins that interact physically with other proteins. A neural network model was established using the characteristic genes of CC by using ANN, which was used to analyze the experimental data and to examine the verification by Cox regression analysis. The differences in TIICs were compared by CIBERSORT. Epigenetic biomarkers need to be considered within the context of differential diagnostic situations, and this is particularly true for CC biomarkers (56-58).
The relationship between viral immune escape and tumor immune escape, the reasons for further changes in the immune microenvironment in CC, and the specific mechanism of the decline of immune killing are not clear. Therefore, we have meticulously analysed the differences of TIICs in CC microenvironment, aiming to identify the crucial elements necessary to maintain stability in the cervical immune microenvironment. Furthermore, we sought to determine effective methods to regulate the proportion of various immune cell compositions and immune evasion mechanisms, thereby providing innovative insights and guidance for immunotherapeutic interventions. In conclusion, ANN was an intelligent method with relatively good sensitivity and specificity in the diagnosis of CC and therefore explore biomarkers, functions, pathways, regulatory factors, and immunotherapy.
Acknowledgments
We are very grateful to Gene Expression Omnibus (GEO) database for providing access to their platforms which enables us to conduct this research.
Funding: None.
Footnote
Reporting Checklist: The authors have completed the STREGA reporting checklist. Available at https://cco.amegroups.com/article/view/10.21037/cco-23-139/rc
Peer Review File: Available at https://cco.amegroups.com/article/view/10.21037/cco-23-139/prf
Conflicts of Interest: All authors have completed the ICMJE uniform disclosure form (available at https://cco.amegroups.com/article/view/10.21037/cco-23-139/coif). The authors have no conflicts of interest to declare.
Ethical Statement: The authors are accountable for all aspects of the work in ensuring that questions related to the accuracy or integrity of any part of the work are appropriately investigated and resolved. The study was conducted in accordance with the Declaration of Helsinki (as revised in 2013).
Open Access Statement: This is an Open Access article distributed in accordance with the Creative Commons Attribution-NonCommercial-NoDerivs 4.0 International License (CC BY-NC-ND 4.0), which permits the non-commercial replication and distribution of the article with the strict proviso that no changes or edits are made and the original work is properly cited (including links to both the formal publication through the relevant DOI and the license). See: https://creativecommons.org/licenses/by-nc-nd/4.0/.
References
- Arbyn M, Weiderpass E, Bruni L, et al. Estimates of incidence and mortality of cervical cancer in 2018: a worldwide analysis. Lancet Glob Health 2020;8:e191-203. [Crossref] [PubMed]
- Hu Z, Ma D. The precision prevention and therapy of HPV-related cervical cancer: new concepts and clinical implications. Cancer Med 2018;7:5217-36. [Crossref] [PubMed]
- Smola S. Immunopathogenesis of HPV-Associated Cancers and Prospects for Immunotherapy. Viruses 2017;9:254. [Crossref] [PubMed]
- Cancer Genome Atlas Research Network. Integrated genomic and molecular characterization of cervical cancer. Nature 2017;543:378-84. [Crossref] [PubMed]
- Lorenc A, Romaszko-Wojtowicz A, Jaśkiewicz Ł, et al. Exploring the efficacy of artificial neural networks in predicting lung cancer recurrence: a retrospective study based on patient records. Transl Lung Cancer Res 2023;12:2083-97. [Crossref] [PubMed]
- Zhang Z. A gentle introduction to artificial neural networks. Ann Transl Med 2016;4:370. [Crossref] [PubMed]
- Agatonovic-Kustrin S, Beresford R. Basic concepts of artificial neural network (ANN) modeling and its application in pharmaceutical research. J Pharm Biomed Anal 2000;22:717-27. [Crossref] [PubMed]
- Nimon KF. Statistical assumptions of substantive analyses across the general linear model: a mini-review. Front Psychol 2012;3:322. [Crossref] [PubMed]
- Tu JV. Advantages and disadvantages of using artificial neural networks versus logistic regression for predicting medical outcomes. J Clin Epidemiol 1996;49:1225-31. [Crossref] [PubMed]
- Parsaeian M, Mohammad K, Mahmoudi M, et al. Comparison of logistic regression and artificial neural network in low back pain prediction: second national health survey. Iran J Public Health 2012;41:86-92. [PubMed]
- Mei L, Meng J, Wei D, et al. Diagnostic test of bioimpedance-based neural network algorithm in early cervical cancer. Ann Transl Med 2022;10:471. [Crossref] [PubMed]
- Hossen MA, Reza MS, Harun-Or-Roshid M, et al. Identification of Drug Targets and Agents Associated with Hepatocellular Carcinoma through Integrated Bioinformatics Analysis. Curr Cancer Drug Targets 2023;23:547-63. [Crossref] [PubMed]
- Li Y, Chen M, Chen Q, et al. Bioinformatics Identification of Therapeutic Gene Targets for Gastric Cancer. Adv Ther 2023;40:1456-73. [Crossref] [PubMed]
- He H, Liu X, Liu Y, et al. Human Papillomavirus E6/E7 and Long Noncoding RNA TMPOP2 Mutually Upregulated Gene Expression in Cervical Cancer Cells. J Virol 2019;93:e01808-18. [Crossref] [PubMed]
- Reza MS, Hossen MA, Harun-Or-Roshid M, et al. Metadata analysis to explore hub of the hub-genes highlighting their functions, pathways and regulators for cervical cancer diagnosis and therapies. Discov Oncol 2022;13:79. [Crossref] [PubMed]
- den Boon JA, Pyeon D, Wang SS, et al. Molecular transitions from papillomavirus infection to cervical precancer and cancer: Role of stromal estrogen receptor signaling. Proc Natl Acad Sci U S A 2015;112:E3255-64. [Crossref] [PubMed]
- Pappa KI, Polyzos A, Jacob-Hirsch J, et al. Profiling of Discrete Gynecological Cancers Reveals Novel Transcriptional Modules and Common Features Shared by Other Cancer Types and Embryonic Stem Cells. PLoS One 2015;10:e0142229. [Crossref] [PubMed]
- Reza MS, Harun-Or-Roshid M, Islam MA, et al. Bioinformatics Screening of Potential Biomarkers from mRNA Expression Profiles to Discover Drug Targets and Agents for Cervical Cancer. Int J Mol Sci 2022;23:3968. [Crossref] [PubMed]
- Swartz MA, Iida N, Roberts EW, et al. Tumor microenvironment complexity: emerging roles in cancer therapy. Cancer Res 2012;72:2473-80. [Crossref] [PubMed]
- Newman AM, Liu CL, Green MR, et al. Robust enumeration of cell subsets from tissue expression profiles. Nat Methods 2015;12:453-7. [Crossref] [PubMed]
- Li T, Fu J, Zeng Z, et al. TIMER2.0 for analysis of tumor-infiltrating immune cells. Nucleic Acids Res 2020;48:W509-14. [Crossref] [PubMed]
- Racle J, de Jonge K, Baumgaertner P, et al. Simultaneous enumeration of cancer and immune cell types from bulk tumor gene expression data. Elife 2017;6:e26476. [Crossref] [PubMed]
- Monaco G, Lee B, Xu W, et al. RNA-Seq Signatures Normalized by mRNA Abundance Allow Absolute Deconvolution of Human Immune Cell Types. Cell Rep 2019;26:1627-1640.e7. [Crossref] [PubMed]
- Liebner DA, Huang K, Parvin JD. MMAD: microarray microdissection with analysis of differences is a computational tool for deconvoluting cell type-specific contributions from tissue samples. Bioinformatics 2014;30:682-9. [Crossref] [PubMed]
- Mandrekar JN. Receiver operating characteristic curve in diagnostic test assessment. J Thorac Oncol 2010;5:1315-6. [Crossref] [PubMed]
- Su Z, Yang H, Zhao M, et al. MicroRNA-92a Promotes Cell Proliferation in Cervical Cancer via Inhibiting p21 Expression and Promoting Cell Cycle Progression. Oncol Res 2017;25:137-45. [Crossref] [PubMed]
- Roy D, Sheng GY, Herve S, et al. Interplay between cancer cell cycle and metabolism: Challenges, targets and therapeutic opportunities. Biomed Pharmacother 2017;89:288-96. [Crossref] [PubMed]
- Newell M, Baker K, Postovit LM, et al. A Critical Review on the Effect of Docosahexaenoic Acid (DHA) on Cancer Cell Cycle Progression. Int J Mol Sci 2017;18:1784. [Crossref] [PubMed]
- Serrano M, Hannon GJ, Beach D. A new regulatory motif in cell-cycle control causing specific inhibition of cyclin D/CDK4. Nature 1993;366:704-7. [Crossref] [PubMed]
- Serra S, Chetty R. p16. J Clin Pathol 2018;71:853-8. [Crossref] [PubMed]
- Sanchez-Carbayo M, Socci ND, Richstone L, et al. Genomic and proteomic profiles reveal the association of gelsolin to TP53 status and bladder cancer progression. Am J Pathol 2007;171:1650-8. [Crossref] [PubMed]
- An R, Meng S, Qian H. Identification of Key Pathways and Establishment of a Seven-Gene Prognostic Signature in Cervical Cancer. J Oncol 2022;2022:4748796. [Crossref] [PubMed]
- Parker MW, Botchan MR, Berger JM. Mechanisms and regulation of DNA replication initiation in eukaryotes. Crit Rev Biochem Mol Biol 2017;52:107-44. [Crossref] [PubMed]
- Zheng J. Diagnostic value of MCM2 immunocytochemical staining in cervical lesions and its relationship with HPV infection. Int J Clin Exp Pathol 2015;8:875-80. [PubMed]
- Liu D, Zhang XX, Xi BX, et al. Sine oculis homeobox homolog 1 promotes DNA replication and cell proliferation in cervical cancer. Int J Oncol 2014;45:1232-40. [Crossref] [PubMed]
- Kaur G, Balasubramaniam SD, Lee YJ, et al. Minichromosome Maintenance Complex (MCM) Genes Profiling and MCM2 Protein Expression in Cervical Cancer Development. Asian Pac J Cancer Prev 2019;20:3043-9. [Crossref] [PubMed]
- Aihemaiti G, Kurata M, Nogawa D, et al. Subcellular localization of MCM2 correlates with the prognosis of ovarian clear cell carcinoma. Oncotarget 2018;9:28213-25. [Crossref] [PubMed]
- Hasegawa M, Kurata M, Yamamoto K, et al. A novel role for acinus and MCM2 as host-specific signaling enhancers of DNA-damage-induced apoptosis in association with viral protein gp70. Leuk Res 2009;33:1100-7. [Crossref] [PubMed]
- Gou K, Liu J, Feng X, et al. Expression of Minichromosome Maintenance Proteins (MCM) and Cancer Prognosis: A meta-analysis. J Cancer 2018;9:1518-26. [Crossref] [PubMed]
- Murphy N, Ring M, Heffron CC, et al. p16INK4A, CDC6, and MCM5: predictive biomarkers in cervical preinvasive neoplasia and cervical cancer. J Clin Pathol 2005;58:525-34. [Crossref] [PubMed]
- Chua MLK, Lo W, Pintilie M, et al. A Prostate Cancer "Nimbosus": Genomic Instability and SChLAP1 Dysregulation Underpin Aggression of Intraductal and Cribriform Subpathologies. Eur Urol 2017;72:665-74. [Crossref] [PubMed]
- Li J, Pu K, Li C, et al. A Novel Six-Gene-Based Prognostic Model Predicts Survival and Clinical Risk Score for Gastric Cancer. Front Genet 2021;12:615834. [Crossref] [PubMed]
- Yamamoto F, Yamamoto M. Identification of genes that exhibit changes in expression on the 8p chromosomal arm by the Systematic Multiplex RT-PCR (SM RT-PCR) and DNA microarray hybridization methods. Gene Expr 2008;14:217-27. [Crossref] [PubMed]
- Contzler R, Favre B, Huber M, et al. Cornulin, a new member of the "fused gene" family, is expressed during epidermal differentiation. J Invest Dermatol 2005;124:990-7. [Crossref] [PubMed]
- Malumbres M, Barbacid M. To cycle or not to cycle: a critical decision in cancer. Nat Rev Cancer 2001;1:222-31. [Crossref] [PubMed]
- Kim HD, Park SH. Immunological and clinical implications of immune checkpoint blockade in human cancer. Arch Pharm Res 2019;42:567-81. [Crossref] [PubMed]
- Liu Y, Wu L, Tong R, et al. PD-1/PD-L1 Inhibitors in Cervical Cancer. Front Pharmacol 2019;10:65. [Crossref] [PubMed]
- Xiong Y, Wang K, Zhou H, et al. Profiles of immune infiltration in colorectal cancer and their clinical significant: A gene expression-based study. Cancer Med 2018;7:4496-508. [Crossref] [PubMed]
- Shah W, Yan X, Jing L, et al. A reversed CD4/CD8 ratio of tumor-infiltrating lymphocytes and a high percentage of CD4(+)FOXP3(+) regulatory T cells are significantly associated with clinical outcome in squamous cell carcinoma of the cervix. Cell Mol Immunol 2011;8:59-66. [Crossref] [PubMed]
- Walch-Rückheim B, Ströder R, Theobald L, et al. Cervical Cancer-Instructed Stromal Fibroblasts Enhance IL23 Expression in Dendritic Cells to Support Expansion of Th17 Cells. Cancer Res 2019;79:1573-86. [Crossref] [PubMed]
- Sheu BC, Lin RH, Lien HC, et al. Predominant Th2/Tc2 polarity of tumor-infiltrating lymphocytes in human cervical cancer. J Immunol 2001;167:2972-8. [Crossref] [PubMed]
- Schröer N, Pahne J, Walch B, et al. Molecular pathobiology of human cervical high-grade lesions: paracrine STAT3 activation in tumor-instructed myeloid cells drives local MMP-9 expression. Cancer Res 2011;71:87-97. [Crossref] [PubMed]
- Heusinkveld M, de Vos van Steenwijk PJ, Goedemans R, et al. M2 macrophages induced by prostaglandin E2 and IL-6 from cervical carcinoma are switched to activated M1 macrophages by CD4+ Th1 cells. J Immunol 2011;187:1157-65. [Crossref] [PubMed]
- Piersma SJ. Immunosuppressive tumor microenvironment in cervical cancer patients. Cancer Microenviron 2011;4:361-75. [Crossref] [PubMed]
- Tian J, Geng Y, Lv D, et al. Using plasma cell-free DNA to monitor the chemoradiotherapy course of cervical cancer. Int J Cancer 2019;145:2547-57. [Crossref] [PubMed]
- Yu R, Zhang L, Yu Q, et al. Effect of LHX2 gene methylation level and its function on radiotherapy of cervical cancer. Transl Cancer Res 2021;10:2944-61. [Crossref] [PubMed]
- Kong L, Wang L, Wang Z, et al. DNA methylation for cervical cancer screening: a training set in China. Clin Epigenetics 2020;12:91. [Crossref] [PubMed]
- Xu W, Xu M, Wang L, et al. Integrative analysis of DNA methylation and gene expression identified cervical cancer-specific diagnostic biomarkers. Signal Transduct Target Ther 2019;4:55. [Crossref] [PubMed]