A sub-cluster of cancer cells indicates poor prognosis in advanced hepatocellular carcinoma
Highlight box
Key findings
• This study found that the CancerCell 6 cluster were exhibited significant disparities in both overall survival and progression-free survival rates according to the 370 patients with hepatocellular carcinoma (HCC) from The Cancer Genome Atlas database. The CancerCell 6 cluster was associated with adenosine triphosphate (ATP)-related pathways and the high expression of pyruvate kinase M (PKM). It also demonstrated a strong interaction ability with mononuclear phagocytes (MPs).
What is known and what is new?
• It is known that tumor cells exhibited the Warburg effect by breaking down glucose into lactate through glycolysis, and ATP-related metabolism is the key in glycolysis.
• It is unclear that whether ATP-related metabolism (such as PKM) is the crucial target of glycolysis for advanced HCC treatment, especially in a certain sub-group.
What is the implication, and what should change now?
• The CancerCell 6 cluster associated with a poor prognosis and characterized by ATP-related metabolism in patients with advance HCC. This cluster demonstrated interactions with MPs inclined to M2 polarization.
Introduction
Over 50% of patients diagnosed with hepatocellular carcinoma (HCC) are at advanced or terminal stage in most regions or countries (1). According to Barcelona Clinic Liver Cancer (BCLC) 2022, advanced-stage HCC is characterized by vascular invasion and/or extrahepatic spread, an Eastern Cooperative Oncology Group (ECOG) performance score ≤2, and preserved liver function (2). Patients with advanced HCC often undergo systemic therapies, either alone or in combination with locoregional therapies, such as ablation, transarterial chemoembolization (TACE), and transarterial radioembolization. Currently, numerous drugs for systemic therapy exist, primarily categorized as target therapy and immunotherapy (3,4). The optimal treatment for advanced HCC remains uncertain, although combined therapies have shown better performances than monotherapies (5-10). However, the overall response rate (ORR) without locoregional therapy combination is typically no more than 40%, and the ORR with TACE combination is around 60% with overall survival (OS) generally not exceeding 24 months.
One of the primary reasons for the therapeutic ineffectiveness is intratumoral heterogeneity (ITH) (11). While many studies on HCC patients have focused on ITH on in the context of the immune landscape and the relationship between malignant cells (12-15), few have elaborated the heterogeneity of malignant cells themselves and their significance for treatment and prognosis. This is particularly crucial for advanced HCC with limited treatment options and unsatisfactory prognosis. Ma et al. observed both intertumoral and intratumoral transcriptomic heterogeneity in HCC malignant cells (16), noticing that SPP1 expression was linked to tumor cell evolution and microenvironmental reprogramming (17). Losic et al. investigated ITH and clonal evolution in early-stage HCC (18). Sun et al. explored the ecosystem of early-relapse HCC, assessing the contribution of malignant cells (19). Accordingly, it is emergent to explore the ITH in early-stage HCC for timely intervention. However, as tumor progress, interactions with tumor microenvironment lead to the evolution of tumor cells, resulting in increased complexity of ITH in the majority of advanced HCC patients (20,21). Therefore, it is imperative to focus on elucidating different classifications of ITH and understanding the mechanisms of treatment resistance in patients with advance HCC to improve prognosis.
This study sought to classify the cancer cell sub-clusters in patients with advanced HCC and identify the related pathways and microenvironments of the key sub-cluster that associated with prognosis. We present this article in accordance with the REMARK reporting checklist (available at https://cco.amegroups.com/article/view/10.21037/cco-24-58/rc).
Methods
Tissue samples from patients with advanced HCC
Two cases of advanced HCC were randomly selected based on the following criteria: (I) patients with unresectable HCC at an advanced stage scheduled to undergo locoregional and systemic treatments; (II) patients able to tolerate the biopsy procedure; (III) tumor cell suspensions with over 20,000 single cells, exhibiting more than 85% activity and less than 20% red blood cell ratio and impurity. Tumor tissues were procured immediately during the biopsy procedure at The First Affiliated Hospital of Nanjing Medical University. The study received approval from the Institutional Review Board of The First Affiliated Hospital of Nanjing Medical University (No. 2023-SR-926), and informed consent was obtained from all patients. The study was conducted in accordance with the Declaration of Helsinki (as revised in 2013).
Sample processing and data analysis
Fresh tissues, stored in the sCelLiveTM tissue preservation solution (Singleron, Nanjing, China) on ice, were processed within 30 minutes of biopsy. The specimens underwent impurity removal and analysis, as detailed in the Supplementary file (Appendix 1). The clinical information of the samples was listed in Table S1. Raw reads were processed to generate gene expression profiles using CeleScope (v1.14.0, Singleron, Nanjing, China). Quality control, dimensionality reduction, clustering, and differentially expressed genes (DEGs) were performed using Scanpy (v1.8.1) under Python 3.7. Gene Ontology (GO) and Kyoto Encyclopedia of Genes and Genomes (KEGG) analyses were conducted to explore potential functional pathways (22). Cell-type recognition was accomplished using Cell-ID (23). Predicted cell-cell interactions were based on known ligand-receptor pairs. Pseudo-time trajectory analysis was employed to reconstruct the cell differentiation trajectory of cancer cell and mononuclear phagocyte (MP) subtypes. Functional gene module analysis illustrated heterogeneity within cancer cell subpopulations. Gene set scoring was performed to identify M1 polarization or M2 polarization among MP subtypes by using UCell (v2.2.0) (24). The InferCNV package was applied to detect the copy number alterations in cancer cells for distinguishing the degree of malignancy.
Statistical analysis
Two-tailed Wilcoxon rank-sum test was applied to compare the expression level of individual genes involved in the signature scores between cell sub-clusters. Bulk RNA-seq transcriptome data and clinical information from 370 HCC patients were obtained from The Cancer Genome Atlas (TCGA) database. The samples were categorized into two groups (high expression and low expression) based on the median expression value of the target gene to evaluate the relationship with clinical factors. Kaplan-Meier curves were used to compare OS and progression-free survival (PFS) between high and low expression groups, with P values calculated via log-rank test using the Survival package in R (v4.1.2) software. R (v4.1.2) software was used to perform all statistical analyses.
Results
Single-cell transcriptomic profiling from advanced HCC tumors
A total of 26,800 cells, comprising nine distinct types, were obtained from two advanced HCC patients, including cancer cells, endothelial cells (ECs), hepatic stellate cells (HepSCs), B cells, plasma cells, T and NK cells, neutrophils, mast cells, and MPs (Figure 1A,1B). The top three cell types, namely cancer cells, T and NK cells, and MPs, constituted most of these samples (Figure 1C). Heat maps were generated to visualize the top 10 (Figure 1D) and top 3 (Figure 1E) differential genes among the identified cell types.
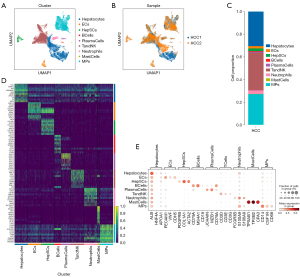
The sub-clusters of cancer cells and prognostic analysis
From a total of 7,829 cancer cells, we identified seven distinct sub-clusters labeled as “CancerCells 1–7” through cell dimension reduction (Figure 2A). CancerCells 1 and 2 were the predominant sub-clusters (Figure 2B). Copy number variation analysis identified that CancerCell 4 had the highest degree of malignancy, while CancerCell 7 had the lowest (Figure S1A,S1B). The top 10 (Figure 2C) and top 3 (Figure 2D) differential genes for each sub-clusters were highlighted in heat maps. Gene set variation analysis (GSVA) revealed that the CancerCell 6 cluster exhibited strong signaling pathways associated with tumor stem score, hypoxia, MHC-I expression, and epithelial-mesenchymal transition (Figure S1C). Additionally, the CancerCell 6 cluster displayed elevated expressions of transcription factors, including XBP1, ATF4, and MXI1 (Figure S1D,S1E).
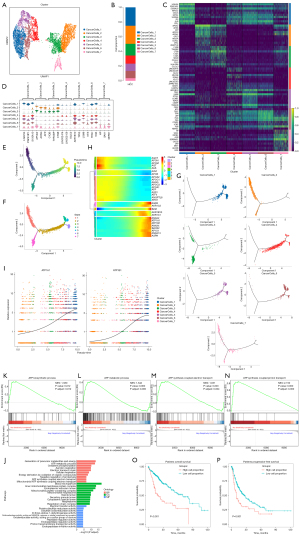
Pseudo-time analysis demonstrated that CancerCell 1, 4, 5, and 6 were situated at the later stages of cell differentiation, while CancerCell 2, 3, and 7 were at the earlier and intermediate differentiation stages (Figure 2E-2G). Beam analysis illustrated the gene expression changes in each sub-cluster (Figure 2H), with ATP1A1 and ATP1B1 showing increased expression with pseudo-time (Figure 2I). GO enrichment analysis highlighted adenosine triphosphate (ATP) metabolic process and ATP synthesis coupled electron transport as enrichment pathways in the CancerCell 6 cluster (Figure 2J). GSEA analysis further revealed significant enrichment of ATP biosynthetic process, ATP metabolic process, ATP synthesis coupled electron transport, and ATP synthesis coupled proton transport in the CancerCell 6 cluster compared to other clusters (Figure 2K-2N).
Kaplan-Meier curve analysis using TCGA database indicated significantly longer OS and PFS in the low cell proportion group of CancerCell 6 compared to the high cell proportion group (Figure 2O,2P). CancerCell 4 and 5 clusters also exhibited significant differences in OS between high and low cell proportion groups (Figure S1F,S1G).
The correlation of the CancerCell 6 cluster with ATP related metabolism
Hotspot analysis divided cancer cells into nine modules (Figure 3A). Jaccard similarity analysis revealed that the CancerCell 6 cluster was most similar to module 8, following by module 9 (Figure 3B). GO and KEGG enrichment analyses indicated enrichment of ATP and glycolysis-related pathways in module 8 (Figure 3C,3D) and endopeptidase-related signaling pathways in module 9 (Figure S2A,S2B). Gene-related prognosis analysis identified high expression of PKM, GAPDH, AKP1C3, ANXA2, CD63, EN01, TP11, SQSTM1, S100A10, and PSMA7 were associated with poor prognosis (Figure 3E). Kaplan-Meier curve analysis confirmed shorter OS in high-level group of PKM (Figure 3F) and GAPDH (Figure 3G). Besides, high gene expression groups had poor OS than the low gene expression groups, including AKR1C3, ANXA2, CD63, ENO1, TPI1, SQSTM1, S100A10 and PSMA7 (Figure S3A-S3H). It is well-established that PKM is associated with glycolysis related pathways. The similarity analysis indicated PKM was inversely correlated with ATP biosynthetic process, ATP metabolic process, ATP synthesis coupled electron transport, and ATP synthesis coupled proton transport (Figure 3H).
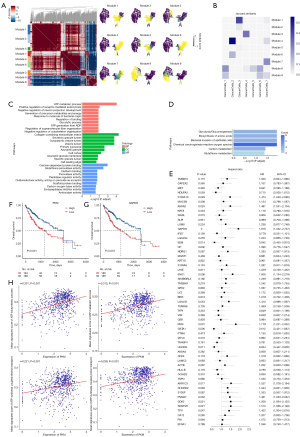
The correlation of the CancerCell 6 cluster with MPs
Cell communication analysis showed that the CancerCell 6 cluster exhibited the strongest interaction with MPs among immune cells (Figure 4A). The interaction between the CancerCell 6 cluster and MPs was associated with high expression of receptor-ligand pairs, including CSF1/CSF1R, TNFSF10/TNFRSF10B and LGALS9/HAVCR2 (Figure 4B,4C).
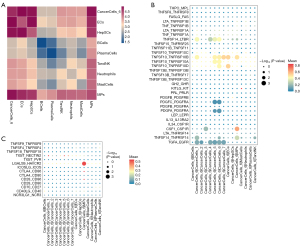
The sub-clusters of MPs and prognostic analysis
From 4,631 cells, we identified five heterogeneous sub-clusters of MPs: MPs_VCAN, MPs_FOLR2, MPs_TREM2, MPs_MMP9 and MPs_CXCL10 (Figure 5A). MPs_VCAN, MPs_FOLR2 and MPs_TREM2 constituted most MPs (Figure 5B). The top 3 differential genes between each sub-cluster of MPs were shown in the violin plots (Figure 5C). Pseudo-time analysis showed MPs_MMP9 was at the front of cell differentiation, but the other 4 sub-clusters were all distributed at every stages (Figure 5D,5E). UCell analysis for M1 and M2 polarizations showed that MPs_MMP9 tended toward M2 polarization, whereas MPs_CXCL10 tended toward M1 polarization (Figure 5F-5H). Prognosis analysis confirmed that a high MPs_MMP9 group had significantly shorter OS than a low MPs_MMP9 group (Figure 5I), a pattern also observed in MPs_TREM2 (Figure S4).
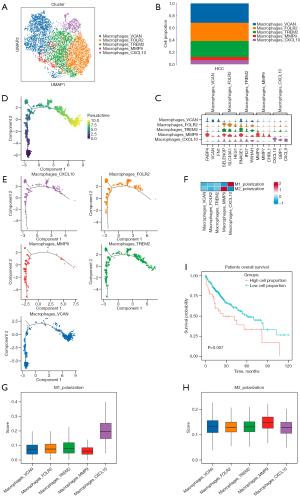
The correlation between the CancerCell 6 cluster and MPs_MMP9
CellChat analysis revealed strong interactions between CancerCell 6 and MPs_MMP9 (Figure 6A,6B), which were related to the EDN and NOTCH signaling pathways (Figure 6C,6D).
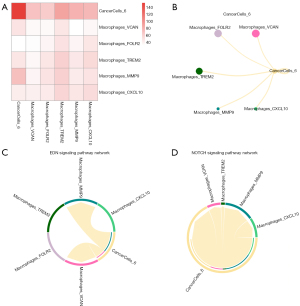
Discussion
In this study, we identified the CancerCell 6 cluster as significantly associated with poor OS and PFS in patients with advanced HCC. This cluster emerged as a key representation of malignant cells in advanced HCC, signifying a poor prognosis. Pseudo-time analysis positioned the CancerCell 6 cluster at the posterior stage of tumor evolution, exhibiting enrichment in ATP-related pathways, indicative of deregulated cellular metabolism (25). Under anaerobic or hypoxic conditions, normal cells undergo the decomposition of glucose or glycogen into lactate and a small amount of ATP. Despite the energy demand for rapid cell multiplication, even under aerobic conditions, tumor cells exhibited the Warburg effect by breaking down glucose into lactate through glycolysis (26). Both PKM (pyruvate kinase M) and GAPDH (glyceraldehyde-3-phosphate dehydrogenase), the key enzymes in glycolysis, were associated with OS of HCC. It suggested that PKM and GAPDH can represent important targets for advanced HCC treatment. This finding is supported by Du et al.’s review of metabolic dysregulation and emerging therapeutic targets for HCC (27). Nevertheless, it remains unknown and requires further studies to determine whether PKM is the most crucial target of glycolysis for advanced HCC treatment, especially in cases with a high cell proportion of CancerCell 6.
We found that CancerCell 6 exhibits a robust interaction capability with MPs due to the high expression of receptor-ligand pairs, notably CSF1/CSF1R, TNFSF10/TNFRSF10B, and LGALS9/HAVCR2. Jia et al. reported that peritumoral CSF-1R was associated with intrahepatic metastasis, tumor recurrence, and survival after hepatectomy (28). Ao et al. demonstrated that CSF-1R blockade could impede HCC tumor growth by shifting the polarization to M1 (29). Therefore, considering the high proportion of CancerCell 6 in advanced HCC, utilizing CSF-1R blockade may enhance treatment effectiveness. TNFSF10/TNFRSF10B, also known as TRAIL/TRAILR2 (DR5), can induce apoptosis in tumor cells (30). Loss of TRAIL receptors is a frequent feature of HCC and an independent predictor of survival in patients after partial hepatectomy (31). Low TRAIL mRNA levels have been associated with HCC development, growth, tumor recurrence, and poorer survival rates (32). Fortunately, sorafenib can reportedly restore TRAIL sensitivity in HCC cells, improving anticancer effects (33). While a phase 2 clinical trial combining tigatuzumab (a DR5 agonist) with sorafenib in adults with advanced HCC did not meet the efficacy endpoints, it showed a good tolerance profile (34). Busche et al. found that the treatment response of HCC to sorafenib-based pro-apoptotic agent combinations (e.g., TRAIL) depends on specific BH3-only proteins (35). Therefore, assessing individual BH3-only protein expression could aid in selecting HCC patients for tyrosine kinase inhibitors (TKIs) and pro-apoptotic agent combinations. LGALS9/HAVCR2, also known as Galectin9/Tim3, is a promising treatment approach in current immunotherapy (36). Li et al. discovered Galectin9/Tim3 signaling pathway mediates T cell senescence in HBV-associated HCC, with high Tim3 expression in T cells correlating with poor survival (37). Yang et al. revealed that Galectin9 is a PD1-binding protein, contributing to the persistence of PD1+Tim3+ T cells and attenuating Galectin9/Tim3-induced cell death (38). In summary, in addition to TKI and PD1/PDL1 inhibitor combinations, agents related to CSF1/CSF1R, TNFSF10/TNFRSF10B and LGALS9/HAVCR2 may enhance the effectiveness of treating advanced HCC with high CancerCell 6 expression.
This study further unveiled a distinct sub-cluster of cancer cells, denoted as CancerCell 6, characterized by poor prognosis and strong interactions with the MPs_MMP9 cluster, which exhibited a tendency toward M2 polarization. Confirmation of the unfavorable prognosis was evident in the high MPs_MMP9 group of HCC patients, with implicating signaling pathways including EDN and NOTCH. The EDN (endothelin) signaling pathway was identified as a regulator of various cellular processes, such as proliferation, apoptosis, migration, epithelial-to-mesenchymal transition, chemoresistance and neovascularization (39,40). Zhang et al. indicated that HCC patients with lower EDN receptor type B expression had a more unfavorable prognosis (41). Furthermore, elevated EDN1 expression in HCC tissues was established, and downregulation of EDN1 demonstrated significant inhibition of cell cycle arrest, and promotion of apoptosis, suggesting a potential therapeutic target for HCC (42). Additionally, a study highlighted the role of SMAD7 in activating the YAP/NOTCH signaling cascade, contributing to cholangiocellular carcinoma and epithelial-mesenchymal transition signatures implicated in liver carcinogenesis (43). NOTCH-active signatures have been identified in approximately 30% of human HCCs, resembling a cholangiocarcinoma-like HCC transcriptionally, associated with poor prognosis, and recognized as a distinct HCC molecular subtype (44). The NOTCH signaling pathway was also associated with M1 and M2 polarization in HCC (45,46). Activating the NOTCH signaling pathway can regulate the differentiation of M1 polarization, promoting inflammation and antitumor activity (46).
There are some limitations in this study. It remains to be explored that whether the CancerCell 6 cluster is universal in advanced HCC with portal invasion or specific to a particular subtype. Identifying a high-risk subset of cancer cells in advanced HCC with portal invasion could facilitate precision therapies. Potential pathways or targets, such as ATP, PKM, CSF1, TNFSF10, LGALS9, EDN, and NOTCH, may enhance the effectiveness of precision therapies. However, the intricate associations between these pathways and targets, as well as the optimal combinations for treatments, remain unclear. Thus, further studies are warranted to explore optimized strategies for different sub-clusters of advanced HCC. Besides, this study had a relatively small sample size, which might limit the robustness of conclusions. For overcoming the small sample limitation, TCGA database with bulk RNA-seq transcriptome data was used to validate the key points, but this study has the limitations without confirmatory experiments. Certainly, the evidences and appropriate controls are needed in further studies. In vitro and in vivo studies would benefit to elucidate the underlying molecular mechanisms. Larger-scale studies are still required to confirm and extend our findings. Prospectively, novel targeted agents are being developed rapidly. By combining with novel agents, treatment strategies are becoming more diverse and personalized, which may promote treatment response and improve the prognosis for patients with advanced HCC.
Conclusions
In conclusion, this study sheds light on the CancerCell 6 cluster, a sub-cluster of cancer cell associated with poor prognosis in advanced HCC, revealing connections to ATP-related metabolism and interactions with MPs favoring to M2 polarization. Our study also identifies pertinent pathways and targets that may enhance treatment effectiveness for advanced HCC.
Acknowledgments
None.
Footnote
Reporting Checklist: The authors have completed the REMARK reporting checklist. Available at https://cco.amegroups.com/article/view/10.21037/cco-24-58/rc
Data Sharing Statement: Available at https://cco.amegroups.com/article/view/10.21037/cco-24-58/dss
Peer Review File: Available at https://cco.amegroups.com/article/view/10.21037/cco-24-58/prf
Funding: This study was supported by
Conflicts of Interest: All authors have completed the ICMJE uniform disclosure form (available at https://cco.amegroups.com/article/view/10.21037/cco-24-58/coif). H.F.Z. reports that this study was supported by the Jiangsu Provincial Innovative and Entrepreneurial Doctor Program (No. 303073543ER21) and the Young Scholars Fostering Fund of The First Affiliated Hospital of Nanjing Medical University (No. PY2023056). H.B.S. reports that this study was supported by the Jiangsu Province Capability Improvement Project through Science, Technology and Education (No. JSDW202243). W.Z.Z. reports that this study was supported by the National Natural Science Foundation of China (No. 81701802). The other authors have no conflicts of interest to declare.
Ethical Statement: The authors are accountable for all aspects of the work in ensuring that questions related to the accuracy or integrity of any part of the work are appropriately investigated and resolved. The study received approval from the Institutional Review Board of The First Affiliated Hospital of Nanjing Medical University (No. 2023-SR-926), and informed consent was obtained from all patients. The study was conducted in accordance with the Declaration of Helsinki (as revised in 2013).
Open Access Statement: This is an Open Access article distributed in accordance with the Creative Commons Attribution-NonCommercial-NoDerivs 4.0 International License (CC BY-NC-ND 4.0), which permits the non-commercial replication and distribution of the article with the strict proviso that no changes or edits are made and the original work is properly cited (including links to both the formal publication through the relevant DOI and the license). See: https://creativecommons.org/licenses/by-nc-nd/4.0/.
References
- Park JW, Chen M, Colombo M, et al. Global patterns of hepatocellular carcinoma management from diagnosis to death: the BRIDGE Study. Liver Int 2015;35:2155-66. [Crossref] [PubMed]
- Reig M, Forner A, Rimola J, et al. BCLC strategy for prognosis prediction and treatment recommendation: The 2022 update. J Hepatol 2022;76:681-93. [Crossref] [PubMed]
- Yang C, Zhang H, Zhang L, et al. Evolving therapeutic landscape of advanced hepatocellular carcinoma. Nat Rev Gastroenterol Hepatol 2023;20:203-22. [Crossref] [PubMed]
- Rizzo A, Ricci AD, Brandi G. Trans-Arterial Chemoembolization Plus Systemic Treatments for Hepatocellular Carcinoma: An Update. J Pers Med 2022;12:1788. [Crossref] [PubMed]
- Zhu HD, Li HL, Huang MS, et al. Transarterial chemoembolization with PD-(L)1 inhibitors plus molecular targeted therapies for hepatocellular carcinoma (CHANCE001). Signal Transduct Target Ther 2023;8:58. [Crossref] [PubMed]
- Jin ZC, Zhong BY, Chen JJ, et al. Real-world efficacy and safety of TACE plus camrelizumab and apatinib in patients with HCC (CHANCE2211): a propensity score matching study. Eur Radiol 2023;33:8669-81. [Crossref] [PubMed]
- Qin S, Chan SL, Gu S, et al. Camrelizumab plus rivoceranib versus sorafenib as first-line therapy for unresectable hepatocellular carcinoma (CARES-310): a randomised, open-label, international phase 3 study. Lancet 2023;402:1133-46. [Crossref] [PubMed]
- Galle PR, Finn RS, Qin S, et al. Patient-reported outcomes with atezolizumab plus bevacizumab versus sorafenib in patients with unresectable hepatocellular carcinoma (IMbrave150): an open-label, randomised, phase 3 trial. Lancet Oncol 2021;22:991-1001. [Crossref] [PubMed]
- Ren Z, Xu J, Bai Y, et al. Sintilimab plus a bevacizumab biosimilar (IBI305) versus sorafenib in unresectable hepatocellular carcinoma (ORIENT-32): a randomised, open-label, phase 2-3 study. Lancet Oncol 2021;22:977-90. [Crossref] [PubMed]
- Peng Z, Fan W, Zhu B, et al. Lenvatinib Combined With Transarterial Chemoembolization as First-Line Treatment for Advanced Hepatocellular Carcinoma: A Phase III, Randomized Clinical Trial (LAUNCH). J Clin Oncol 2023;41:117-27. [Crossref] [PubMed]
- Dagogo-Jack I, Shaw AT. Tumour heterogeneity and resistance to cancer therapies. Nat Rev Clin Oncol 2018;15:81-94. [Crossref] [PubMed]
- Zheng C, Zheng L, Yoo JK, et al. Landscape of Infiltrating T Cells in Liver Cancer Revealed by Single-Cell Sequencing. Cell 2017;169:1342-1356.e16. [Crossref] [PubMed]
- Zhang Q, Lou Y, Yang J, et al. Integrated multiomic analysis reveals comprehensive tumour heterogeneity and novel immunophenotypic classification in hepatocellular carcinomas. Gut 2019;68:2019-31. [Crossref] [PubMed]
- Zhang Q, He Y, Luo N, et al. Landscape and Dynamics of Single Immune Cells in Hepatocellular Carcinoma. Cell 2019;179:829-845.e20. [Crossref] [PubMed]
- Ho DW, Tsui YM, Chan LK, et al. Single-cell RNA sequencing shows the immunosuppressive landscape and tumor heterogeneity of HBV-associated hepatocellular carcinoma. Nat Commun 2021;12:3684. [Crossref] [PubMed]
- Ma L, Hernandez MO, Zhao Y, et al. Tumor Cell Biodiversity Drives Microenvironmental Reprogramming in Liver Cancer. Cancer Cell 2019;36:418-430.e6. [Crossref] [PubMed]
- Ma L, Wang L, Khatib SA, et al. Single-cell atlas of tumor cell evolution in response to therapy in hepatocellular carcinoma and intrahepatic cholangiocarcinoma. J Hepatol 2021;75:1397-408. [Crossref] [PubMed]
- Losic B, Craig AJ, Villacorta-Martin C, et al. Intratumoral heterogeneity and clonal evolution in liver cancer. Nat Commun 2020;11:291. [Crossref] [PubMed]
- Sun Y, Wu L, Zhong Y, et al. Single-cell landscape of the ecosystem in early-relapse hepatocellular carcinoma. Cell 2021;184:404-421.e16. [Crossref] [PubMed]
- Alizadeh AA, Aranda V, Bardelli A, et al. Toward understanding and exploiting tumor heterogeneity. Nat Med 2015;21:846-53. [Crossref] [PubMed]
- Sia D, Villanueva A, Friedman SL, et al. Liver Cancer Cell of Origin, Molecular Class, and Effects on Patient Prognosis. Gastroenterology 2017;152:745-61. [Crossref] [PubMed]
- Wolf FA, Angerer P, Theis FJ. SCANPY: large-scale single-cell gene expression data analysis. Genome Biol 2018;19:15. [Crossref] [PubMed]
- Cortal A, Martignetti L, Six E, et al. Gene signature extraction and cell identity recognition at the single-cell level with Cell-ID. Nat Biotechnol 2021;39:1095-102. [Crossref] [PubMed]
- Andreatta M, Carmona SJ. UCell: Robust and scalable single-cell gene signature scoring. Comput Struct Biotechnol J 2021;19:3796-8. [Crossref] [PubMed]
- Hanahan D, Weinberg RA. Hallmarks of cancer: the next generation. Cell 2011;144:646-74. [Crossref] [PubMed]
- Vander Heiden MG, Cantley LC, Thompson CB. Understanding the Warburg effect: the metabolic requirements of cell proliferation. Science 2009;324:1029-33. [Crossref] [PubMed]
- Du D, Liu C, Qin M, et al. Metabolic dysregulation and emerging therapeutical targets for hepatocellular carcinoma. Acta Pharm Sin B 2022;12:558-80. [Crossref] [PubMed]
- Jia JB, Wang WQ, Sun HC, et al. High expression of macrophage colony-stimulating factor-1 receptor in peritumoral liver tissue is associated with poor outcome in hepatocellular carcinoma after curative resection. Oncologist 2010;15:732-43. [Crossref] [PubMed]
- Ao JY, Zhu XD, Chai ZT, et al. Colony-Stimulating Factor 1 Receptor Blockade Inhibits Tumor Growth by Altering the Polarization of Tumor-Associated Macrophages in Hepatocellular Carcinoma. Mol Cancer Ther 2017;16:1544-54. [Crossref] [PubMed]
- Ware CF. The TNF Superfamily-2008. Cytokine Growth Factor Rev 2008;19:183-6. [Crossref] [PubMed]
- Kriegl L, Jung A, Engel J, et al. Expression, cellular distribution, and prognostic relevance of TRAIL receptors in hepatocellular carcinoma. Clin Cancer Res 2010;16:5529-38. [Crossref] [PubMed]
- Piras-Straub K, Khairzada K, Trippler M, et al. TRAIL expression levels in human hepatocellular carcinoma have implications for tumor growth, recurrence and survival. Int J Cancer 2015;136:E154-60. [Crossref] [PubMed]
- Jiang W, Wu DB, Fu SY, et al. Insight into the role of TRAIL in liver diseases. Biomed Pharmacother 2019;110:641-5. [Crossref] [PubMed]
- Cheng AL, Kang YK, He AR, et al. Safety and efficacy of tigatuzumab plus sorafenib as first-line therapy in subjects with advanced hepatocellular carcinoma: A phase 2 randomized study. J Hepatol 2015;63:896-904. [Crossref] [PubMed]
- Busche S, John K, Wandrer F, et al. BH3-only protein expression determines hepatocellular carcinoma response to sorafenib-based treatment. Cell Death Dis 2021;12:736. [Crossref] [PubMed]
- Zhao L, Cheng S, Fan L, et al. TIM-3: An update on immunotherapy. Int Immunopharmacol 2021;99:107933. [Crossref] [PubMed]
- Li H, Wu K, Tao K, et al. Tim-3/galectin-9 signaling pathway mediates T-cell dysfunction and predicts poor prognosis in patients with hepatitis B virus-associated hepatocellular carcinoma. Hepatology 2012;56:1342-51. [Crossref] [PubMed]
- Yang R, Sun L, Li CF, et al. Galectin-9 interacts with PD-1 and TIM-3 to regulate T cell death and is a target for cancer immunotherapy. Nat Commun 2021;12:832. [Crossref] [PubMed]
- Wang R, Dashwood RH. Endothelins and their receptors in cancer: identification of therapeutic targets. Pharmacol Res 2011;63:519-24. [Crossref] [PubMed]
- Rosanò L, Spinella F, Bagnato A. Endothelin 1 in cancer: biological implications and therapeutic opportunities. Nat Rev Cancer 2013;13:637-51. [Crossref] [PubMed]
- Zhang L, Luo B, Dang YW, et al. The clinical significance of endothelin receptor type B in hepatocellular carcinoma and its potential molecular mechanism. Exp Mol Pathol 2019;107:141-57. [Crossref] [PubMed]
- Shi L, Zhou SS, Chen WB, et al. Functions of endothelin-1 in apoptosis and migration in hepatocellular carcinoma. Exp Ther Med 2017;13:3116-22. [Crossref] [PubMed]
- Wang H, Song X, Liao H, et al. Overexpression of Mothers Against Decapentaplegic Homolog 7 Activates the Yes-Associated Protein/NOTCH Cascade and Promotes Liver Carcinogenesis in Mice and Humans. Hepatology 2021;74:248-63. [Crossref] [PubMed]
- Zhu C, Ho YJ, Salomao MA, et al. Notch activity characterizes a common hepatocellular carcinoma subtype with unique molecular and clinicopathologic features. J Hepatol 2021;74:613-26. [Crossref] [PubMed]
- Sharma A, Seow JJW, Dutertre CA, et al. Onco-fetal Reprogramming of Endothelial Cells Drives Immunosuppressive Macrophages in Hepatocellular Carcinoma. Cell 2020;183:377-394.e21. [Crossref] [PubMed]
- Chen W, Liu Y, Chen J, et al. The Notch signaling pathway regulates macrophage polarization in liver diseases. Int Immunopharmacol 2021;99:107938. [Crossref] [PubMed]