Drug designs fulfilling the requirements of clinical trials aiming at personalizing medicine
Introduction
The development of new therapeutics in oncology typically follows the phase I, phase II, and phase III drug development paradigm. In phase I, the primary goal is to understand the safety profile of a new treatment in a small group of patients (typically including multiple tumor types) for further investigation. In the phase II setting, the primary goal is to better understand the efficacy profile in order to make a determination of whether the treatment is worthy of further investigation with a secondary goal of gaining a better understanding of the treatment’s safety. Traditionally, this has been accomplished through a single arm or a randomized trial in a homogenous group of patients, with the trial size varying from 30 to 100 patients. If the agent is considered safe with a promising efficacy signal, it is then taken forward into a phase III trial, where the primary goal is to compare the new treatment or regimen with the standard of care to demonstrate a clinical benefit, and/or in some cases, cost-effectiveness. Phase III trials are usually large (few hundreds to few thousands of patients), and are done in a homogenous group of patients in a multi-institution setting.
This traditional drug development paradigm is challenged by the fact that cancer is increasingly becoming a “rare” disease with the use of targeted therapeutics and biomarker assessment for medical treatment. Medical treatment for oncology patients is driven by a combination of the expected outcome for the patient (prognosis), and the ability for treatment to improve the expected outcome (prediction). The standard paradigm of drug development is thus called into question in the setting of biomarker based trials. In the context of personalized medicine utilizing biomarkers and targeted therapeutics, a “phase I” study tests the methods of assessment of marker alteration in normal and tumor tissue samples and guides in the determination of cut points, if applicable, for quantitative and meaningful interpretation of results. The feasibility of obtaining the specimens as well as the reliability and reproducibility of the assay is often established at this stage. A “phase II” study is typically a careful retrospective assessment of the marker to establish clinical value, and phase III trials are prospective confirmatory trials that attempt to validate the marker through large randomized controlled trials (RCTs) in a multi-center setting (1).
Biomarkers can be classified into three categories: prognostic biomarkers, predictive biomarkers, and surrogate endpoints, with the recognition that some biomarkers may fall into more than one category (2,3). Prognostic and predictive biomarkers focus on individual patient risk-classification and treatment selection respectively, whereas biomarkers used as surrogate endpoints aid in the evaluation of the efficacy of a new treatment. The ultimate intended usage of a biomarker usually determines its definition and the required validation methods. A prognostic biomarker predicts the natural history of the disease process in a given individual, and thus aids in the decision of whether a patient needs an intensive and possibly toxic treatment as opposed to no treatment or standard therapy. Prognostic marker validation can be established using the marker and outcome data from a cohort of uniformly treated patients with adequate follow-up since a prognostic marker is associated with the disease or the patient, and not a specific therapy. The validation data source may be from a clinical trial (due to availability of follow-up information) with patients treated on the standard of care arm (or placebo if that is the standard of care), but a clinical trial is not necessarily required.
A predictive biomarker predicts whether an individual patient will respond to a particular therapy or not, and hence its clinical utility is in allowing for individualized therapy. Designs for predictive marker validation are more complex and require at a fundamental level data from a randomized study (4). A surrogate endpoint biomarker replaces the ultimate clinical outcome (i.e., usually overall survival) and informs the efficacy of a new treatment with greater cost-effectiveness than the primary clinical outcome at the population level (5,6). We focus our attention on predictive markers in this review article. We start with a discussion of phase I dose-finding trials, followed by designs for initial marker validation in a phase II setting. Trial designs for definitive marker validation have been extensively discussed in the literature (3,4,7-12); here we provide a summary of the key features and requirements of phase III designs for definitive predictive marker validation. Finally, we will provide examples of real clinical trials that have utilized some of the designs discussed in this article, and conclude with our thoughts on the future perspectives of drug designs aimed at personalizing medicine.
Early phase dose finding trials
While assessing the safety profile and establishing the maximum tolerated dose (MTD) remains the primary focus of phase I trials for all agents, establishing a preliminary efficacy signal and/or identification of subsets of patients most likely to benefit from the new treatment are increasingly assessed as part of phase I trials of targeted therapeutics. The choice of endpoints, patient selection, model-based versus rule-based design algorithms and inclusion of expansion cohorts need carefully consideration in this setting. The historical paradigm of the higher the dose, the greater the chance of efficacy is called into question when evaluating molecularly-targeted therapies, vaccines and immunotherapy agents. While a monotonically non-decreasing dose-toxicity curve definitely continues to be appropriate from a biological standpoint for molecularly-targeted therapies, the dose-efficacy curves for these novel therapies may follow a non-monotone pattern such as a quadratic curve or an increasing curve with a plateau, and often times not well understood at the beginning of the trial (13). Dose finding studies for such agents should therefore incorporate a measure of efficacy in addition to assessment of toxicity with the aim to identify the biologically optimal dose (BOD), or the minimum effective dose (MED) instead of just the MTD. While the incorporation of an early efficacy measure seems straightforward, it poses many inherent challenges. Firstly, should the efficacy measure be based on a biomarker response, or a clinical response? If it is a clinical response, can it be assessed easily and quickly, and does early change to the clinical outcome provide evidence of a sustained clinical benefit? Secondly, if a biomarker response is sought, is there sufficient evidence demonstrating that the impact on the biomarker would translate to a meaningful clinical response? What are the characteristics of the assay(s) to assess the biomarker in terms of reproducibility, validity etc.? Thirdly, can the biomarker endpoint be assessed in real time to inform the dose escalation decision process?
Assuming that an efficacy endpoint that is a meaningful “surrogate” for the clinical outcome is available, and one that can be measured reliably and in real time, the question of how best to incorporate this endpoint in the dose escalation/recommended phase II dose determination process needs considerable thought. Phase I trial designs can be broadly categorized into model-based versus rule-based (or algorithm-based) designs (13). In the rule-based designs, small numbers of patients are treated starting at the lowest dose level and the decision to escalate, de-escalate or treat additional patients at the same dose level is based on a pre-specified algorithm related to the occurrence of unacceptable dose-limiting toxicity (DLT). The classical cohorts of 3 design and its many variants (accelerated titration design, two-stage design) are rule-based designs that have been and continue to be widely utilized in oncology (14).
Several ad-hoc rule-based designs have been used in practice in oncology for vaccine based and immunotherapy trials such as: (I) randomize a pre-specified number of patients to all dose levels under investigation (this assumes that there are no safety concerns); or (II) use a rule-based algorithm based on toxicity and efficacy to guide dose escalation (de-escalation), for example, if the observed unacceptable toxicity rate is <33%, and the observed efficacy is below (or above threshold) escalate (or de-escalate/stay at the same level) etc. While these are attractive from an implementation/clinical appeal standpoint, the statistical properties (precision, and sample size) of these designs are often not explored and in many cases can be sub-optimal. On the other hand, model-based designs utilize a statistical model for the dose-toxicity (and dose-efficacy in some cases) relationship to guide the dose-finding process (15-25). Designs that utilize both a safety and efficacy outcome during dose escalation or dose escalation based on safety and inclusion of expansion cohorts to understand efficacy are clearly essential in the context of targeted therapeutics. Model-based designs offer the flexibility to model different possible dose-efficacy curves that can realistically represent the true but unknown underlying dose-efficacy profile of a targeted agent, and allow for flexibility in trial conduct, with improved precision in estimating the dose to take forward for further testing.
Expansion cohorts and patient selection are increasingly being utilized as part of the dose-finding process (either during the dose escalation phase or as part of a dose expansion phase) to try and identify subsets of patient who might benefit most from the treatment (26). Lack of validated assays and/or markers of efficacy, inability to have a real-time assessment of the biomarker, and incomplete understanding of the metabolism of the drug or its pathway often make an enrichment strategy at this stage of agent development non-viable. Notable exceptions include the development of vemurafenib for patients with BRAF mutant melanoma, and crizotinib for ALK positive non-small cell lung cancer (NSCLC) patients, both of which adopted the enrichment strategy from the get go (27,28).
Phase II trials for initial marker validation
Phase II trials have at least three main purposes: assess the regimen/drug under investigation for evidence of efficacy; to evaluate the patient population for a phase III study and to more fully assess the toxicity profile in a larger number of patients than that of a phase I study. A single arm two-stage design could be used to determine if a drug is likely to have a certain level of activity in unselected patients, and if activity is below the level of interest, whether a particular patient selection method can enrich the responding population to meet the targeted level of activity in the selected group (29). Such single-arm designs however conduct comparisons against historical controls, which may be inaccurate given changes in patient population based on biologic sub-setting and/or evolution in imaging technologies (30). McShane et al. (31) show through simulations how misleading the results of a single-arm phase II trial in a selected patient population can be when the benchmark estimate is from prior trials of “unselected” patients and thus inappropriate for the enriched study population. In contrast, a RCT includes a control arm for comparison, thereby assuring that patients who are treated with the agent for whom the marker is purported to be predictive are comparable to those who are not. RCTs are essential for making the distinction between a prognostic and predictive marker, as well as to isolate any causal effect of the marker on therapeutic efficacy from the multitude of other factors that may influence the decision to treat or not to treat a patient (4). In the setting of phase II trials, RCTs also provide the opportunity to simultaneously assess multiple promising therapies (and multiple possible markers) for a given disease.
Several prospective designs for biomarker-directed therapy have been proposed, differing primarily in the study population, randomization scheme, or both (4). Most of these designs were intended primarily in a phase III setting, but have evolved since then to be applicable in an initial marker validation setting, i.e., a phase II setting (32). The design choice is driven by scientific rationale, marker prevalence, strength of preliminary evidence, assay performance, and turn-around times for marker assessment. An all-comers design with retrospective marker evaluation is a reasonable approach when multiple biomarkers are to be assessed and there is insufficient knowledge to use a specific marker to drive design properties. However this is subject to insufficient power determined by the marker prevalence, for example in a study of 100 patients (50/arm), a marker with 10% prevalence would only have approximately five patients per arm on which to base the subgroup evaluation. A slight variation to the all-comers designs is the multiple hypotheses design that specifies prospectively a test for a treatment effect in the overall population and within pre-specified marker subgroups. A biomarker-stratified design specifies accrual target within biomarker-defined subgroups. The fundamental difference between this design and the traditional RCT is that only patients with a valid marker result are eligible and randomized. A separate evaluation of the treatment effect can be tested in the different marker-defined subgroups, or a preliminary test of marker by treatment interaction can be carried out first. Different sequential analysis plans can also be implemented (4,33). Enrichment or targeted designs enroll only patients with a particular marker profile, compared to hybrid designs where only a certain subgroup of patients based on their marker status are randomized between treatments, however patients in the other marker-defined subgroups are assigned the standard of care treatment(s). Finally, recent emphasis has been on outcome adaptive randomization designs that evaluate the success of the drug-biomarker subgroup in an ongoing manner. These designs allow either for the randomization ratio to be altered in order to place more patients on the most promising arm(s), and/or allow for the elimination of the under-performing drugs and/or the biomarker subgroups midway through the trial (34-36). A much simpler alternative to this completely adaptive approach is the direct assignment option design, which allows the option to stop randomization and assign all patients to the experimental arm based on interim analysis results (37). The key considerations for the choice of the phase II design are outlined in Table 1 [adapted from Mandrekar et al. (32)].
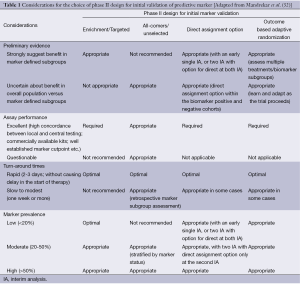
Full table
Definitive phase III trials for marker validation
Frequently, a complete understanding of the drug metabolism pathway or the underlying biology prior to the testing of a therapy (and even approval of the therapy in some cases) is not possible. Similarly, an established cut point to classify patients into different marker subsets is also often not available prior to the start of a phase III trial. Retrospective validation can aid in such situations by bringing forward effective treatments to marker-defined patient subgroups (4). This is likely the only possible solution in cases of where there are approved therapies for an indication, since once a therapy is approved for common use, designs that randomize patients to not use that therapy become exceedingly difficult. The important components of a retrospective validation include availability of clinical and biomarker data from a well-conducted RCT, well established assay characteristics (analytical and clinical validity), availability of samples from a large majority of patients from the RCT to avoid selection bias, and finally and most importantly, well defined prospectively stated hypothesis, sample size and power calculations, analytical techniques, and patient sub-populations to ensure statistical rigor and clinical confidence in the findings. An example of a successful retrospective predictive biomarker validation is the establishment of mutant KRAS status as a predictor of lack of efficacy from panitumumab and cetuximab therapy in advanced colorectal cancer (38-40).
As with initial marker validation in a phase II setting, prospective trials for definitive marker validation also fall under the same classifications, but require greater rigidity than the phase II setting for the statistical parameter standards of type I and type II errors (typically set at 2-sided level of 5% and 10% respectively), and multiplicity adjustments. Specifically, the designs can be classified into enrichment, all-comers (with marker stratified, sequential testing strategy, multiple hypotheses or hybrid designs), or outcome based adaptive randomization designs (3,4). A number of innovative statistical designs using an adaptive strategy for analysis have also been proposed for definitive marker validation. The first is an adaptive accrual design that modifies accrual to two pre-defined marker-defined subgroups based on an interim futility analysis (41). In this design, if the treatment effect in one of the subgroups fails to satisfy a futility boundary, accrual to that subgroup is terminated, and accrual is continued to the other subgroup until the planned total sample size is reached, including accruing subjects that had planned to be included from the terminated subgroup. This design has demonstrated greater power than a non-adaptive trial in simulation settings; however, this strategy might lead to a substantial increase in the accrual duration depending on the prevalence of the marker for the subgroup that continues to full accrual. The second design adaptively modifies accrual, where in the first stage of the trial, only the marker positive group patients are accrued (42). Based on promising interim analysis results, the second stage can continue accrual to the marker positive cohort and also include marker negative patients. If the first stage shows no benefit in the marker positive cohort, then the trial will be closed permanently.
Sequential testing designs are yet another category of phase III designs for validation of markers that control for the type I error rates associated with multiple testing (43-45). These designs are similar to a traditional RCT in that they have a single primary hypothesis, which is either tested in the overall population first and then in a prospectively planned subset if the overall test is not significant, or in the marker-defined subgroup first, and then tested in the entire population if the subgroup analysis is significant (also known as closed testing procedure). The first is recommended in cases where the experimental treatment is hypothesized to be broadly effective, and the subset analysis is ancillary; the second is used when there is strong preliminary data to support that the treatment effect is strongest in the marker-defined subgroup, and that the marker has sufficient prevalence that the power for testing the treatment effect in the subgroup is adequate. A sequential testing strategy can induce potential correlation from testing the overall treatment effect and the treatment effect within the marker-defined subgroup from the same trial population; an approach to appropriately control for such correlations has also been proposed in the literature (45). Freidlin et al. (46) argue that sequential testing designs of the overall/marker-defined subgroup (say, marker-positive) do not appropriately control for the type I error rate for the complementary marker-negative subgroup (i.e., result in higher than acceptable error rate of falsely recommending the new treatment for the marker-negative cohort), when the treatment benefits only the marker-positive cohort. The authors propose instead a strategy called the Marker Sequential Test (MaST) design that allows for sequential testing of the treatment effect in the marker-positive and the marker-negative groups as well as the entire population while appropriately controlling for the type I error rates (46). A more recent class of sequential testing strategy designs specifically applicable to biomarker validation are the adaptive threshold (AT) and the adaptive signature (AS) designs (47-49). AT is useful in cases where a marker is known at the start of the trial, but a cut-point for defining marker positive and marker negative groups is not known. AS is useful when information regarding the marker and the threshold are both unknown; AS design allows for the “discovery and validation” process of the marker within the realm of the single phase III trial, using either a cross validation approach or the split-alpha approach in cases when the treatment is not broadly effective in the overall unselected population (47-49). At least two issues need further consideration with such designs: (I) the added cost of a somewhat larger sample size and/or redundant power dictated by the strategy of partitioning the overall type I error rate; and (II) use of data from the same trial to both define and validate a marker cut-point (3).
Finally, in the current era of stratified medicine, phase II/III designs are gaining popularity as they enable us to use small patient subsets most effectively (50,51). These designs, known as multi-arm multi-stage (MAMS) designs, enable the simultaneous assessment of multiple experimental agents against the standard of care in the phase II portion using an intermediate (or surrogate) endpoint. This eliminates the need to conduct separate (large-scale) phase II trials to evaluate each experimental regimen. The phase III portion will subsequently continue with the promising experimental arms from the phase II portion, comparing them to the standard of care (50,51).
Examples of biomarker-based trials in the phase II and phase III setting
In this section, we provide examples of ongoing, in development or completed cancer clinical trials that utilized a design strategy aiming at personalized medicine.
Outcome based adaptive randomization design
The biomarker-integrated approaches of targeted therapy of lung cancer elimination (BATTLE) trial used an outcome based adaptive randomization design for randomizing patients to treatment choices based on multiple biomarker profiles in NSCLC (9). This trial is completed and accrued ~200 patients who had their tumors tested for 11 different biomarkers (categorized into five biomarker subgroups), and were randomized to one of four treatment choices. The first 97 patients were assigned using a balanced randomization to one of the four treatments equally. All subsequent patients were adaptively randomized, where the randomization rate was proportional to the eight week disease control rate. As hypothesized, the results from the trial showed that each drug works best for patients with a specific molecular profile (52). Two successor trials, BATTLE 2 and BATTLE 3, also following an adaptive design strategy, will attempt to confirm these initial findings.
Multiple hypothesis design
A subgroup-focused, multiple-hypothesis design was utilized in the phase III SWOG S0819 that incorporates co-primary endpoints to assess cetuximab in both the overall study population (all-comers) as well as within a pre-specified biomarker subgroup (epidermal growth factor receptor (EGFR) fluorescent in-situ hybridization (FISH)-positive, FISH+), with the sample size determined based on evaluation in the EGFR FISH(+) group (53). This trial evaluates both the value of cetuximab in this setting (overall general efficacy objective) and EGFR FISH as a predictive biomarker. With progression-free survival as the primary endpoint (targeting an improvement of 20% in the overall and 33% in the subgroup), and allocating 80% of the type I error rate to the subgroup hypothesis (1-sided study wide type I error of 2.5%), the sample size for this trial is 1,420 patients, with 564 in the EGFR FISH+ group (53).
Combination designs
An example of a phase III trial utilizing an enrichment followed by a marker by treatment interaction design to validate the predictive value of the K-ras mutation, EGFR protein expression, and EGFR gene copy number is the Tailor trial in second line NSCLC (54) (Figure 1). The primary hypotheses, based on a 2-sided interaction test with 95% power, is that docetaxel (D) is better than erlotinib (E) in Group A (30% improvement in OS, for a HR of 1.43 in favor of D), and E better than D in Group B (21% improvement in OS, for a HR of 0.79 in favor of E). A limitation of this trial is that the secondary within group comparisons are not adequately powered to detect clinically relevant differences in outcomes. Another example of a phase III trial using a combination design of an enrichment strategy followed by a marker-based strategy design is trial 0601, coordinated by the Spanish Lung Cancer Group, comparing erlotinib with chemotherapy in stage IV NSCLC patients with EGFR mutations (55) (Figure 2).
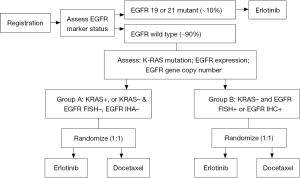
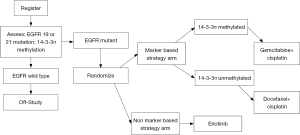
National Cancer Institute (NCI) precision medicine initiative
The NCI’s recent focus is to develop trials where patients are screened for certain molecular characteristics that may predict for response to a targeted therapy, the so-called genotype to phenotype initiative. At least three trials are in development to address this paradigm: the adjuvant lung cancer enrichment marker identification and sequencing trial (ALCHEMIST) (Figure 3), the molecular profiling based assignment of cancer therapeutics (M-PACT) (Figure 4), and the molecular analysis for therapy choice (NCI-MATCH) (Figure 5).
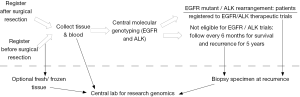
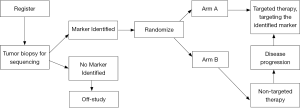
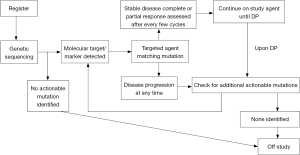
Concluding remarks
Cancer is increasingly becoming a “rare” disease with the use of targeted therapeutics and biomarker assessment for medical treatment. Design of phase I, phase II and phase III trials has thus undergone a rapid evolution in the last decade. The focus has shifted from predictions based on the traditional anatomic staging systems to guide the choice of treatment for an individual patient to an integrated approach using the genetic makeup of the tumor and the genotype of the patient. In the setting of early phase dose-finding trials, identification of the MTD and assessment of the safety profile is no longer the only goal; a preliminary assessment of efficacy has become a necessity in order to identify a so-called MED to take forward into phase II trials. A better understanding of the tumor biology (identifying patient subsets, rare tumor subtypes, etc.), advancement in assay techniques, and availability of commercial kits with rapid turn-around times have popularized enrichment designs in phase II and phase III trials, allowing only patients with a particular molecular profile to be eligible for the trial. Tailored treatments with effective biomarker-driven hypotheses are leading to smaller clinical trials targeting larger treatment effects. Phase II/III designs are gaining popularity as small patient subsets will require us to not ‘waste’ patients. The NCI’s initiative to promote and focus on molecularly driven trials has provided impetus to design trials that match the right patient to the right drug. Finally, advancements in technology such as mobile computing, electronic data capture, and integration of research records with electronic medical records has made real time access to clinical trial and biomarker data a reality, allowing adaptive designs to take on a much greater role in clinical trials.
Acknowledgements
Supported in part by the National Cancer Institute Grant: Mayo Clinic Cancer Center (CA-15083).
Disclosure: The authors declare no conflict of interest.
References
- Hammond ME, Taube SE. Issues and barriers to development of clinically useful tumor markers: a development pathway proposal. Semin Oncol 2002;29:213-21. [PubMed]
- Sargent DJ, Mandrekar SJ. Statistical issues in the validation of prognostic, predictive, and surrogate biomarkers. Clin Trials 2013;10:647-52. [PubMed]
- Mandrekar SJ, Sargent DJ. Clinical Trial Designs for Biomarker Evaluation. Personalized Medicine in Oncology, Vol 1, No 1-Biomarkers, May 2012.
- Mandrekar SJ, Sargent DJ. Clinical trial designs for predictive biomarker validation: theoretical considerations and practical challenges. J Clin Oncol 2009;27:4027-34. [PubMed]
- Prentice RL. Surrogate endpoints in clinical trials: Definition and operational criteria. Stat Med 1989;8:431-40. [PubMed]
- Buyse M, Sargent DJ, Grothey A, et al. Biomarkers and surrogate end points--the challenge of statistical validation. Nat Rev Clin Oncol 2010;7:309-17. [PubMed]
- Simon RM, Paik S, Hayes DF. Use of Archived Specimens in Evaluation of Prognostic and Predictive Biomarkers. J Nat Cancer Inst 2009;101:1446-52. [PubMed]
- Barker AD, Sigman CC, Kelloff GJ, et al. I-SPY 2: an adaptive breast cancer trial design in the setting of neoadjuvant chemotherapy. Clin Pharmacol Ther 2009;86:97-100. [PubMed]
- Zhou X, Liu S, Kim ES. Bayesian adaptive design for targeted therapy development in lung cancer-a step towards personalized medicine. Clinical Trials 2008;5:181-93. [PubMed]
- Simon R, Wang SJ. Use of genomic signatures in therapeutics development in oncology and other diseases. Pharmacogenomics J 2006;6:166-73. [PubMed]
- Jiang W, Freidlin B, Simon R. Biomarker-adaptive threshold design: a procedure for evaluating treatment with possible biomarker-defined subset effect. J Natl Cancer Inst 2007;99:1036-43. [PubMed]
- Freidlin B, Jiang W, Simon R. The Cross-Validated Adaptive Signature Design. Clin Cancer Res 2010;16:691-8. [PubMed]
- Mandrekar SJ, Qin R, Sargent DJ. Model-based phase I designs incorporating toxicity and efficacy for single and dual agent drug combinations: methods and challenges. Stat Med 2010;29:1077-83. [PubMed]
- Storer BE. Choosing a Phase I Design. In Handbook of Statistics in Clinical Oncology. Crowley J, Hoering A. eds. Chapman & Hall/CRC: Boca Raton, FL, 2012:3-20.
- O’Quigley J, Pepe M, Fisher L. Continual reassessment method: A practical design for Phase 1 clinical trials in cancer. Biometrics 1990;46:33-48. [PubMed]
- Braun TM. The bivariate continual reassessment method. extending the CRM to phase I trials of two competing outcomes. Control Clin Trials 2002;23:240-56. [PubMed]
- Thall PF, Cook JD. Dose-finding Based on Efficacy-Toxicity Trade-offs. Biometrics 2004;60:684-93. [PubMed]
- Yin G, Li Y, Ji Y. Bayesian dose-finding in phase I/II clinical trials using toxicity and efficacy odds ratios. Biometrics 2006;62:777-84. [PubMed]
- Bekele BN, Shen Y. A Bayesian Approach to Jointly Modeling Toxicity and Biomarker Expression in a Phase I/II Dose-finding Trial. Biometrics 2005;61:343-54. [PubMed]
- Dragalin V, Fedorov V. Adaptive designs for dose-finding based on efficacy-toxicity response. J Stat Plan Inference 2006;136:1800-23.
- Thall PF, Russell KE. A strategy for dose-finding and safety monitoring based on efficacy and adverse outcomes in phase I/II clinical trials. Biometrics 1998;54:251-64. [PubMed]
- Zhang W, Sargent DJ, Mandrekar SJ. An adaptive dose-finding design incorporating both toxicity and efficacy. Stat Med 2006;25:2365-83. [PubMed]
- Mandrekar SJ, Cui Y, Sargent DJ. An adaptive phase I design for identifying a biologically optimal dose for dual agent drug combinations. Stat Med 2007;26:2317-30. [PubMed]
- Zohar S, Chevret S. Recent developments in adaptive designs for Phase I/II dose-finding studies. J Biopharm Stat 2007;17:1071-83. [PubMed]
- Mandrekar SJ. Dose-finding trial designs for combination therapies in oncology. J Clin Oncol 2014;32:65-7. [PubMed]
- Manji A, Brana I, Amir E, et al. Evolution of clinical trial design in early drug development: systematic review of expansion cohort use in single-agent phase I cancer trials. J Clin Oncol 2013;31:4260-7. [PubMed]
- Chapman PB, Hauschild A, Robert C, et al. Improved Survival with Vemurafenib in Melanoma with BRAF V600E Mutation. N Engl J Med 2011;364:2507-16. [PubMed]
- Shaw AT, Kim DW, Nakagawa K, et al. Crizotinib versus Chemotherapy in Advanced ALK-Positive Lung Cancer. N Engl J Med 2013;368:2385-94. [PubMed]
- Pusztai L, Anderson K, Hess KR. Pharmacogenomic predictor discovery in phase II clinical trials for breast cancer. Clin Cancer Res 2007;13:6080-6. [PubMed]
- Tang H, Foster NR, Grothey A, et al. Comparison of error rates in single-arm versus randomized phase II cancer clinical trials. J Clin Oncol 2010;28:1936-41. [PubMed]
- McShane LM, Hunsberger S, Adjei AA. Effective incorporation of biomarkers into phase II trials. Clin Cancer Res 2009;15:1898-905. [PubMed]
- Mandrekar SJ, An MW, Sargent DJ. A review of phase II trial designs for initial marker validation. Contemp Clin Trials 2013;36:597-604. [PubMed]
- Shi Q, Mandrekar SJ, Sargent DJ. Predictive biomarkers in colorectal cancer: usage, validation, and design in clinical trials. Scand J Gastroenterol 2012;47:356-62. [PubMed]
- Berry DA. Adaptive clinical trials: the promise and the caution. J Clin Oncol 2011;29:606-9. [PubMed]
- Barker AD, Sigman CC, Kelloff GJ, et al. I-SPY 2: an adaptive breast cancer trial design in the setting of neoadjuvant chemotherapy. Clin Pharmacol Ther 2009;86:97-100. [PubMed]
- Zhou X, Liu S, Kim ES. Bayesian adaptive design for targeted therapy development in lung cancer-a step towards personalized medicine. Clinical Trials 2008;5:181-93. [PubMed]
- An MW, Mandrekar SJ, Sargent DJ. A 2-Stage Phase II Design with Direct Assignment Option in Stage II for Initial Marker Validation. Clin Cancer Res 2012;18:4225-33. [PubMed]
- Jonker DJ, O’Callaghan CJ, Karapetis CS, et al. Cetuximab for the treatment of colorectal cancer. N Engl J Med 2007;357:2040-8. [PubMed]
- Karapetis CS, Khambata-Ford S, Jonker DJ, et al. K-ras mutations and benefit from cetuximab in advanced colorectal cancer. N Engl J Med 2008;359:1757-65. [PubMed]
- Van Cutsem E, Köhne CH, Hitre E, et al. Cetuximab and chemotherapy as initial treatment for metastatic colorectal cancer. N Engl J Med 2009;360:1408-17. [PubMed]
- Wang SJ, O’Neill RT, Hung HM. Approaches to evaluation of treatment effect in randomized clinical trials with genomic subset. Pharm Stat 2007;6:227-44. [PubMed]
- Liu A, Liu C, Li Q, et al. A threshold sample-enrichment approach in a clinical trial with heterogeneous subpopulations. Clin Trials 2010;7:537-45. [PubMed]
- Simon R, Wang SJ. Use of genomic signatures in therapeutics development in oncology and other diseases. Pharmacogenomics J 2006;6:166-73. [PubMed]
- Bauer P. Multiple testing in clinical trials. Stat Med 1991;10:871-89; discussion 889-90. [PubMed]
- Song Y, Chi GY. A method for testing a prespecified subgroup in clinical trials. Stat Med 2007;26:3535-49. [PubMed]
- Freidlin B, Korn EL, Gray R. Marker Sequential Test (MaST) design. Clin Trials 2014;11:19-27. [PubMed]
- Jiang W, Freidlin B, Simon R. Biomarker-adaptive threshold design: a procedure for evaluating treatment with possible biomarker-defined subset effect. J Natl Cancer Inst 2007;99:1036-43. [PubMed]
- Freidlin B, Simon R. Adaptive signature design: an adaptive clinical trial design for generating and prospectively testing a gene expression signature for sensitive patients. Clin Cancer Res 2005;11:7872-8. [PubMed]
- Freidlin B, Jiang W, Simon R. The cross-validated adaptive signature design. Clin Cancer Res 2010;16:691-8. [PubMed]
- Hunsberger S, Zhao Y, Simon R. A comparison of phase II study strategies. Clin Cancer Res 2009;15:5950-5. [PubMed]
- Parmar MK, Barthel FM, Sydes M, et al. Speeding up the evaluation of new agents in cancer. J Natl Cancer Inst 2008;100:1204-14. [PubMed]
- Kim ES, Herbst RS, Wistuba II, et al. The BATTLE trial: personalizing therapy for lung cancer. Cancer Discov 2011;1:44-53. [PubMed]
- Redman MW, Crowley JJ, Herbst RS, et al. Design of a phase III clinical trial with prospective biomarker validation: SWOG S0819. Clin Cancer Res 2012;18:4004-12. [PubMed]
- Farina G, Longo F, Martelli O, et al. Rationale for treatment and study design of tailor: a randomized phase III trial of second-line erlotinib versus docetaxel in the treatment of patients affected by advanced non-small-cell lung cancer with the absence of epidermal growth factor receptor mutations. Clin Lung Cancer 2011;12:138-41. [PubMed]
- Rosell R, Taron M, Sanchez JJ, et al. Setting the benchmark for tailoring treatment with EGFR tyrosine kinase inhibitors. Future Oncol 2007;3:277-83. [PubMed]