From genomic data analysis to drug development: a new generation of trials using molecular marker assessment in breast cancer
Introduction
Breast cancer is the leading cause of cancer death for women worldwide (1,2) and breast cancer diversity is a crucial point to consider accurate categorization and treatment of patient subpopulations (3,4). Current breast cancer classification and assessment remain strongly based on clinicopathological criteria (patient age, tumor size, lymph node invasion, histological type, and grade) (5). Besides, histological characterization is driven by development of targeted therapies, including endocrine and anti-HER2 therapies (estrogen and progesterone receptors -ER and PR- and HER2 expressions respectively), and by proliferation index evaluation (Ki67).
In parallel to chemotherapy, personalized treatments were initiated with the first targeted therapy, tamoxifen in the 1970’s. This antiestrogenic treatment was followed later by fulvestrant and aromatase inhibitor commercialization. About 25 years later, the antiHER2-antibody trastuzumab was the next step in sub-dividing large cohorts of patient populations for personalized treatment. The efficiency of trastuzumab was based on the specific amplification of the ERBB2 gene in some breast cancers. Since then, no other targeted drug could add such benefits, using gene mutations or rearrangements specific for breast cancers.
Besides individual mutation analysis, gene expression microarrays have allowed simultaneous expression analysis of thousands of genes in a single experiment in order to create molecular tumor profiles. In 2000, Perou and colleagues published the first classification of breast cancers into four intrinsic subtypes, based on the gene expression profiling from unsupervised hierarchical analysis of complementary DNA (cDNA) microarray data (6,7): luminal A, luminal B, HER2-enriched (HER2-E), and basal-like. New classifications have emerged defining now two additional subtypes: normal breast-like and more recently, the claudin-low or mesenchymal-like subtype (8,9). Although the so-called triple negative tumors are mainly represented among the basal-like subtype, the two subtypes do not completely overlap (10-12). These new intrinsic classifications are in permanent evolution and new signaling pathway identification for each subtype has proven to be useful for drug discovery and for identification of new molecular markers, such as luminal androgen receptor (AR) subtypes (4,12,13).
At primary diagnosis, approximately 60% of patients with invasive breast cancer are node-negative, with 94% of these expected to have no distant metastasis at 10 years if treated by locoregional and adjuvant systemic therapy (www.tumoregister-muenchen.de). The HER2-negative, hormone receptor (HR) positive population can be intrinsically divided in subpopulations with low, average, and high risks of recurrence. Adjuvant therapy, either endocrine or chemotherapy, should be decided accordingly but risk stratification based on only clinicopathological parameters may cause under- or overtreatment. Since 2007, international guidelines [e.g., St Gallen, American Society of Clinical Oncology (ASCO), Arbeitsgemeinschaft Gynäkologische Onkologie (AGO)] have recommended to combine validated protein or gene expression tests reflecting the intrinsic tumor characteristics to improve the clinical risk stratification (14,15). Genomic information is now combined with the clinicopathological characteristics to estimate recurrence risk (prognostic value) and to predict therapy efficacy (predictive value). Identifying high-risk patients for recurrence and administering optimal therapies but avoiding overtreatment are major issues in breast cancer management as therapy resistance and metastasis processes need to be effectively targeted for improvement of survival (16).
Endocrine therapy resistance is a constant threat during patient treatment, as tumor cells may escape the antiestrogenic drugs by adapting the ER-pathway and/or by switching from their usual signaling pathway to an alternate one (17-21). Moreover, the intrinsic phenotypic and genotypic heterogeneities of the primary tumors and of the micrometastases are omitted whenever a single biopsy from the primary tumor is performed to establish the treatment (22-24). Other resistance mechanisms clearly involve miRNA and epigenetic regulations [for review (25-28)]. Recent advances in next-generation sequencing technologies provide the opportunity for new prospective clinical trials that should ideally be affordable as routine tests, using limited amounts of material. This may not be an utopia any longer to allow for each patient, the identification of druggable genes, i.e., targets of efficient drugs or drug combination against resistance mechanisms specifically hormone therapy ones (13,29,30).
We will present in this review a brief history of the genome analysis evolution over the last 15 years, followed by their main applications in breast cancer sub-typing and clinical trials for HR positive breast cancer, and their implications for drug development.
Genomic characterization of breast cancers
The human genome project [for review (31)]
The human genome project was initiated in 1986, with the hypothesis, among others, that some of the cellular genes could drive cancer apparition and progression. The impressive amount of data collected over 13 years, after the project completion in 2003, was only the basement for the elucidation of the numerous genes involved in each individual cancer (32). Within the last 10 years, another revolution happened with the development of the large-scale or high-throughput studies of mutations and other gene alterations, leading far beyond the traditional Sanger sequencing technique.
Next generation sequencing (NGS) and actual high-throughput genomic assays
From 2005, NGS or massive parallel sequencing (MPS) technologies allowed time and money affordable analyses as they are applicable on tumor material to generate information on the whole genome within few days and thousands euros (33-35). They both rely on the sequencing of a sequence library of the targeted nucleic acid, followed by synthesis sequencing. The huge amount of data has then to be processed through powerful and rapid data analysis tools. After the current marker leader, Illumina HiSeq sequencer, three major new sequencing platforms have been released in 2011, Ion Torrent’ PGM, Pacific Biosciences’ RS and Illumina MiSeq (36). The applications of NGS are extending every day not only to DNA, but to RNA and epigenome sequences as presented below, competing with the microarray techniques.
The Whole Genome Sequencing (WGS) developed enough in the recent years to generate data that considerably improve the diagnostic, prognostic and treatments of cancer patients (37). It allows the analysis of genes and regulatory elements with all types of mutations and aberrations from single base point mutations to copy number aberrations, DNA rearrangements or chromosome-scale amplification.
More recently, the amplification and sequencing of each coding exon [Whole Exome Sequencing (WES)] of 18,000 genes clearly evidenced and confirmed some “cancer” genes (e.g., TP53 for breast cancers) and recurrent “driver” mutations to distinguish from “one-off” mutations (38). Although the WES only identifies point mutations and small insertion/deletion in the coding regions of the genes, but not larger scale DNA rearrangements, it may become a routine analysis due to its lower cost and number of data to process and store (just over 1% of the whole genome), compared to WGS. The routine feasibility of WES allowed its integration in numerous large scale analyses of tumors from up to 500 patients, for rational decision making.
The cDNA sequencing or RNA-seq. was then the next approach to explore any alteration from the transcriptome, by sequencing mRNA, mi-RNA and other RNAs. RNA-seq provides first of all gene expression levels, but information on the aberrant splicings, chimeric gene fusion transcripts features, too (39-41). Nonetheless, the reverse transcription step is not reliable enough to allow the characterization of point mutations.
The targeted sequencing of specific genes or regions, known to be highly involved in the disease development or progression may be a more affordable and rapid option than the WGS and WES (42). The development of such disease-targeted sequencing is a promising approach to develop.
The technology for a systematic analysis of chromatin and epigenetic modifications in cancer cells is still in development but encouraging data arose from the ENCODE Project Consortium’s genome-wide (43).
Other “omics” technologies
In parallel to the DNA and RNAs analysis, high-throughput sequencing technologies have been developed for protein comprehensive analysis, such as the Reverse-Phase Protein Array (RPPA) (44). The proteome and the phosphoproteome analysis generate very sensible data for the functional interpretation of the alterations observed in the signaling pathways of tumor cells.
Using these powerful tools, The Cancer Genome Atlas (TCGA) (http://cancergenome.nih.gov), the Cancer Genome Project (http://www.sanger.ac.uk/genetics/CGP), the International Cancer Genome Consortium [(ICGC), (45)] and others initiated the complex comprehensive analysis of the mutations present in various cancers (46,47). By using multiplexed screens, i.e., multiple high throughput genotyping platforms, the data of combined powerful technologies are pooled for an integrated analysis. The open questions for many cancer researchers today may be the criteria to select NGS rather than microarray technology for each definite project or trial to set up. There is no clear answer as both have advantages and limitations. The main advantages of microarrays are still the much lower cost of the analyses, the quicker preparation of the samples and analysis of the data, and the 20-year expertise of many laboratories worldwide. Regarding the NGS, each application has to be considered independently: for RNA-Seq, the main advantage over microarrays may be that it covers all aspects the transcriptome (non-coding RNAs, splice junctions, novel transcripts, etc) without previous definition of specific probes. Similarly, for the methylation projects, the NGS is much more complete but much more expensive too at the moment. Consequently, a good balance may be to prefer the NGS approach for wide screenings and then the microarrays for rapid profiling. Any progression of the NGS technologies towards diagnostic application will need strong validation through clinical trials. We will now present the TCGA breast cancer project analysis that cleverly combined both technologies.
TCGA breast cancer project
The TCGA breast cancer project was conducted to analyze 510 breast cancers on six platforms for DNA sequencing (WES by MPS technology), DNA copy numbers [Affymetrix 6.0 Single Nucleotide Polymorphism (SNP) arrays], DNA methylation (Illumina Infinium DNA methylation chips), mRNA expression (Agilent mRNA expression microarrays), miRNA sequencing, and protein and phosphoprotein expression (RPPA) (30,32,48).
In the initial four breast cancer subtypes defined by Perou in 2000 (6), the luminal breast cancers have been first described as having a gene expression signature that includes estrogen receptor 1 (ESR1), GATA-binding protein 3 (GATA-3), forkhead bow protein A1 (FOXA1), B-cell chronic lymphocytic leukemia (CLL)/lymphoma 2 (BCL2), X-Box binding protein 1 (XPB1) and the myeloblastosis gene (MYB), which are highly characteristic of the luminal epithelial cells in the inner layer of a normal breast duct. Luminal B cells differ from luminal A in their lower levels of these luminal gene expressions, higher level of proliferation genes and a worse clinical outcome (7). The HER2-E breast cancers expressed higher levels of HER2 and of growth factor-bound protein 7 (GRB7), but not all HER2-E cells are HER2 amplified and they can belong to the luminal or basal-like subtypes in some cases.
The TCGA innovative large scale screening demonstrated 30,626 mutations in the 510 analyzed tumors. The four main subtypes were confirmed by presenting striking differences in their mutation spectra. The luminal breast cancers exhibited a lower mutation rate than the two other subtypes but the most diverse and recurrent Significantly Mutated Genes (SMG), i.e., genes mutated more frequently than the background mutation rate, suggesting their causative role in the specific initiation and development of the luminal cancers. Luminal A breast cancers were characterized by a high frequency of mutations in the phosphatidylinositol-4,5-biphosphonate 3-kinase, catalytic subunit alpha (PIK3CA) gene (45%), a low frequency of mutation of TP53 (12%) and multiple SMGs such as mitogen-activated protein kinase kinase kinase 1 (MAP3K1), GATA3, cadherin 1 (CDH1), and mitogen-activated protein kinase kinase 4 (MAP2K4). MAP2K4 mutation was observed almost exclusively in this subtype.
Compared to the luminal A subtype, luminal B breast cancers exhibited a higher rate of TP53 mutation (29%) and a lower rate of PIK3CA mutation (29%). The TP53 antagonist Murine Double Minute 2, MDM2, exhibits a gene amplification associated to the luminal B status (30%).
Regarding both the luminal A and B subtypes, Cyclin D1 is amplified in 40% of them (29% in A and 58% in B), the fibroblastic growth factor receptor 1 (FGFR1) gene is frequently amplified and the histone trimethyltransferase, mixed lineage leukemia gene 3 (MML3), is found mutated too (8% in A and 6% in B). Unique to luminal subtypes are the mutations of GATA3 (14% in A and 15% in B) and to a lower extent the forkhead bOX protein A1 (FOXA1) and of the RUNt-related transcription factor 1 (RUNX1), the three of them being important for the genomic activity of ER (48). Similarly, it was confirmed that the serine threonine kinase MAP3K1 that regulates the extracellular signal-regulated kinase (ERK) and c-JuN amino-terminal kinase (JNK) kinase pathways and the nuclear-factor-kappa-B (NFκB) signaling, is mutated almost exclusively in the luminal subtypes (13% in A and 5% in B), with a mutual exclusivity with MAP2K4 mutations, the serine threonine kinase downstream of MAP3K1 that activates JNK. Both kinases appear then as driver events of this subtype, although MAP3K1 mutations are associated to a lower tumor grade and proliferation index.
As expected, the HER2-E subtype was characterized by 80% of HER2 amplification, plus highly mutated TP53 (72%) and PIK3CA (39%) genes, and a much lower frequency of the other SMGs.
In comparison, the basal-like subtype carried the highest rate of TP53 mutation (80%), without SMGs except PIK3CA (9%).
It is essential to distinguish the driver mutations from passenger one (29,48); the driver mutations being recurrent mutations observed at higher frequency than expected from background mutation across tumors. They may be the first one to target in drug development. To date, we know that breast cancer genes make up to 25 to 30% of the heritability, including BRCA1, BRCA2, CHEK2, ATM, PALB2, BRIP1, TP53, PTEN, CDH1 and STK11 (49). Genome-Wide Association Studies (GWAS) and international consortia confirmed common polymorphisms individually associated with breast cancer risk, adding a further 14% (49-51). SNPs complete this percentage to 50%, leaving around 50% of the breast cancer without any heritability (48).
The druggable genes against endocrine resistance
As cancer genome sequencing consortia are providing thousands of somatic mutations from the analysis of hundreds of patients, new specific powerful tools are developed in parallel to integrate all the data and rank the priorities of the various candidate druggable genes (52-54). For example, dGene is an annotation tool specific for cancer genome sequencing data, designed to allow any cancer researcher to rapidly identify genes belonging to 1 of 10 druggable classes frequently targeted in drug development, without any biostatistician support (55). The seven druggable classes that have been extracted from the analysis of 77 breast cancer tumors from the TCGA breast cancer project (56) are the G-protein coupled receptors, PI3K receptors, protease inhibitors, proteases, phosphotyrosine phosphatases, serine/threonine kinases and tyrosine kinases.
Introduction of molecular subtypes opened new ways for clinicians to classify, diagnose and treat breast cancers (8,13,57) and endocrine therapy resistance is now clearly defined through guidelines (15). Nonetheless, the diversity and complexity of the biological pathways involved in the endocrine resistance mechanisms are very high limitations. Moreover, besides ERα signaling pathway, other nuclear receptor, essentially PR, ERβ and the AR may be involved in ERα activity modulation (58-63).
Concentrating on the HR positive/luminal tumors, the TCGA data demonstrated that the PIK3CA gene is the most mutated gene in these tumors. The PI3K/AKT/mTOR pathway is involved in essential cell functions including cell growth, proliferation, survival, migration and angiogenesis. As the PIK3CA mutations trigger a gain of function, PIK3CA is a druggable gene. Indeed, the most recent new drug available to overcome endocrine resistance is the rapamycin analog everolimus, a mTOR inhibitor (64), mTOR being a pathway component downstream of PIK3CA. Nonetheless, when everolimus was combined with the aromatase inhibitor exemestane in endocrine resistant advanced breast cancers, PIK3CA mutation status failed to predict benefit outlining the complexity of the interpretation and validation of the genomic data generated (30,65).
Many clinical trials are evaluating PI3K pathway inhibitors, because the direct targeting of PIK3CA seems to be the most promising approach and may lead to molecules with a better tolerability than the rapamycin analogues [for review (30)].
Apart from the PI3K pathway mutations, most of the other gene mutations trigger a loss of function, with predominantly tumor suppressor genes such as the most frequent apoptotic TP53. Although mutations of TP53 cannot be targeted, because they are significantly enriched in the luminal B subtypes (29% compared to 19% in the luminal A) and in tumors with a higher histological grade, a potential role as prognostic marker is suggested (30,32,56,66-69). Moreover, the TP53 antagonist MDM2 exhibits a gene amplification associated to the luminal B status and to endocrine therapy resistance. This was the basis for MDM2 inhibitor development, now in phase I [NCT01462175 (52)].
GATA3 mutations are then the third most common mutations in luminal tumors, following PIK3CA and TP53. These inactivating mutations do not appear to change the proliferation level except in response to neoadjuvant aromatase inhibitor therapy, suggesting that GATA3 mutation could serve as predictor of aromatase inhibitor sensitive disease (56).
The cyclin dependant kinases 4 and 6 (CDK4/6) normally bind to Cyclin D1 to allow pRb phosphorylation and G1/S cell cycle progression. As Cyclin D1 is amplified in 40% of the luminal cancers, especially in luminal B, inhibitors of CDK4/6 have successfully been tested in combination with the aromatase inhibitor letrozole in a completed phase II clinical trial with advanced or metastatic breast cancers patients (30,32,56,66-69).
Clinical trials combining entinostat (a histone desacetylase inhibitor and epigenetic modulator) with the aromatase inhibitor exemestane proved to be active on HR positive advanced breast cancer patients too (30). This outlines the importance of target genes involved in epigenetic regulation, such as the histone trimethyltransferase MML3 mutated in luminal cancers (30,32,56,66-69).
Amplification of the FGFR1 gene is another relatively frequent event in luminal cancers and both antibodies and small inhibitors are developed at the moment (52).
Because multiple inhibitors of specific pathways are proposed (e.g., PI3K/PKB/mTOR), a clear ranking of the specific class and inhibitor needed for each tumor is needed (25,29), remembering that low frequency mutations may be very relevant to target. Other necessary approaches are the functional validation of the relevance of the candidate gene, but cell lines, primary or xenograft cell lines are useful but still limited models. The only ultimate demonstration of the relevance of a definite targeted treatment, in agreement with the related genotype of a subpopulation of patients, will be prospective clinical trials that allow the adaptation of the treatment according to the tumor genotype evolution.
The new generation of prospective clinical trials
In parallel to the breast cancer sub-typing by gene expression profiling, multigene assays have been developed based on a specific prognosis and/or predictive signature [for review (70)]. Among the most widely used platforms are MammaPrint® (microarray) and Oncotype DX® (qRT-PCR), but several other assays are either marketed or in development. Based on numerous retrospective studies, the most advanced tests are now in prospective clinical trials in order to reach a high level of evidence (3,4,71). The development of comprehensive affordable high-throughput genomic platforms was the prerequisite to conduct prospective clinical marker trials in molecularly defined patient populations, and these technologies are now ready to follow the same path as the multigene assays to eventually become decisive treatment decision tools.
Few prospective clinical therapy trials using molecular markers for patient stratification have already been completed (MINDACT for validation of the Mammaprint® assay and TAILORx and WSG Plan B trials for further validation of Oncotype DX®) and results are still expected (72-74). Besides ongoing trials are the RxPONDER/SWOG S1007 (Oncotype DX® in 9,400 node-positive disease) and WSG-Adjuvant Dynamic marker-Adjusted Personalized Therapy (ADAPT) trials (Oncotype DX® in 4,936 invasive early breast cancers from pre-/post-menopausal women with node-negative and -positive disease). The WSG-ADAPT trial (ADAPT trial optimizing risk assessment and therapy response prediction in early breast cancers) is set up as a prospective, multi-center, controlled, non-blinded, randomized phase II/III trial, launched in May 2012 by the WSG, (www.wsg-online.com) (75) (Figure 1). ADAPT is one of the first new generation adjuvant trials expected to establish early predictive molecular surrogate markers for outcome by assessing response to a short 3-week induction treatment, using a baseline diagnostic core biopsy and a second biopsy or surgical tissue sample after induction treatment. ADAPT combines static assessment of prognosis of patients by Oncotype DX® recurrence score in HR positive HER2-negative disease and conventional prognostic markers (nodal status) with dynamic measurement of proliferation/apoptosis changes during the short course of preoperative therapy.
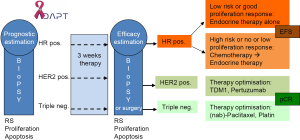
Investigation of Serial studies to Predict Your Therapeutic Response with Imaging And moLecular analysis (I-SPY 1 TRIAL) was a multicenter breast cancer study integrating clinical, imaging and genomic data to evaluate pathological response, recurrence free survival (RFS), and their relationship and predictability based on tumor biomarkers (76-78). The 221 included patients with tumors >3 cm received neoadjuvant anthracycline-based chemotherapy, followed by taxanes. The various molecular classifiers tested were highly correlated. In multivariate analysis, the molecular signatures that added to the ability of HR and HER2 receptors, clinical stage, and pathological complete response (pCR) in predicting RFS included 70-gene signature, wound healing signature, p53 mutation signature, and PAM50 risk of recurrence (78). The I-SPY 1 TRIAL demonstrated the increase of the ability of pCR to predict outcome when analyzed within tumor subtypes. Indeed, pCR is the primary endpoint of the next generation study, the I-SPY 2 TRIAL, which is designed to identify agents early in the drug development cycle that improve the rates of pCR (www.ispy2.org). Patients that are included in the I-SPY 2 TRIAL have newly diagnosed locally advanced breast cancers, with high risk of recurrence. Since the first approval in 2009, five investigational drug combinations have been already tested and should be extended to 12 when the trial will be completed (with more than 1,000 patients) (79,80). This trial is based on adaptive designs that rely on information, including from patients who have not achieved the trial’s primary endpoint.
Such innovant trial designs should allow parallel translational research, by performing adequate NGSs on the tumor samples, before and after the endocrine induction treatment. Moreover, they represent a therapy concept for studying drugs which are supposed to overcome endocrine resistance under routine conditions in early breast cancer.
Such MPS has already been performed on 77 samples from HR-positive tumors from breast cancer patients previously included in two independent trials (56). In both trials, patients received neoadjuvant aromatase inhibitor and tumor materials were collected before treatment. WGS was performed on 46 samples and WES on the 31 others, followed by extensive analysis of somatic alterations and their association with aromatase inhibitors. 18 SMGs were identified among them genes previously identified as mutated in breast cancers (PIK3CA, TP53, GATA3, CDH1, RB1, MLL3, MAP3K1 and CDKN1B) as well as genes not previously observed in clinical breast cancer samples (RUNX1, TBX3, LDLRAP1, STNM2, MYH9, AGTR2, STMN2, SF3B1 and CBFB). Mutated MAP3K1 was associated with the luminal A subtype, low grade histology and low proliferation rate whereas p53 was associated to the opposite pattern. As already cited above, mutant GATA3 was correlated with suppression of proliferation upon aromatase inhibitor treatment. Pathway analysis demonstrated mutations in the MAP3K1 substrate, MAP2K4, inducing similar alteration as direct MAP3K1 loss. Besides, many SMGs appear with low frequencies, making the correlative data analysis more complex. Nonetheless, the authors suggest that for patients with MAP3K1 mutated tumors, luminal A subtype, neoadjuvant aromatase inhibitors are a favorable option. On the contrary, for patients with TP53 mutated tumors, resistance to aromatase inhibitor is expected and an alternate treatment could be selected. PIK3CA was the most common mutation in the luminal subtypes (41.3%) but was neither associated to clinical nor KI67 response. Nonetheless, a positive association was observed between MAP3K1/MAP2K4 mutations and PIK3CA mutation, suggesting an in vivo crosstalk. In any cases, the initial tumor heterogeneity, its evolution along the disease progression and after the pressure of the successive treatments is a key element to consider when analyzing such huge amounts of data.
Another innovative study was the prospective trial SAFIRO/UNICANCER (NCT01414933), conducted with 423 metastatic breast cancer patients enrolled within 11 months in 18 centres in France (81). The aim was to perform molecular screenings to identify abnormalities in individual patients, with the aim of providing targeted therapy matched to individual genomic alterations. Comparative genomic hybridization (CGH) array and Sanger sequencing on PIK3CA (exon 10 and 21) and on AKT1 (exon 4) were used to assess the biopsy samples. Therapy target was then decided accordingly. A targetable genomic alteration was observed in 46% of the patients, and the most frequent one were PIK3CA (25%), CCND1 (19%) and FGFR1 (13%). Mutations and amplifications described above and others (AKT1, EGFR, MDM2, FGFR2, AKT2, IGFR1 and MET amplification), were observed but with a low frequency (<5%). Therapy could be personalized and assessed in 43 patients, with four objective responses (9%) and nine stable diseases for more than 16 weeks (21%). Antitumor activity was observed in patients with PIK3CA, FGFR1, IGF1R, FGF3, AKT1, AKT2 and EGFR gene alterations, although the mutations of the last three genes were located in two rare gene segments that are not conventional driver mutations to focus on.
This challenging clinical trial definitely proved the relevance of such large scale genomic analysis, where ideally, no theoretical or practical restriction should limit the range of mutations to identify in order to adapt the treatment to each patient and limit the resistance mechanisms.
Expected clinical impact
As technologies for DNA/RNA sequencing are improving so quickly to the accuracy, acceptable cost and technical feasibility needed, they reach the standards to be implemented in clinic for the identification of driver mutations. Then, a strict definition of the targeted drug combination to use will be possible, to block the tumor cells of using new signaling pathways that drive endocrine therapy resistances.
All the large-scale genomic analysis already introduced a wide spectrum of candidate genes but reality will force us to focus on a small definite number. Of course, the SMGs and driver mutations are the favorite candidates to target, but we are aware that the low frequency mutated genes and passenger mutations may be relevant too. Because breast cancers are so common, even a low percentage of patients that would benefit from a targeted therapy could represent a significant number of patients worldwide. As not each mutated gene possesses a related drug, then, extensive/exhaustive genomic analysis may not be the most adequate strategy for rational decision-making that could be directed according to available targeted drugs.
So far, clinical trials using NGS for clinical identification of druggable targets in advanced breast cancer have shown that this concept is feasible in clinical routine but requires large patient numbers and sufficient downstream trials with specific inhibitors to make this effort worthwhile for patients, investigators, and industry sponsors. Such a concept for rapid in vivo testing of novel drugs will need a tailored approach by all stakeholders in drug development to be successful in the future. Yet, another alternative to move new compounds rapidly into the early breast cancer setting provided that sufficient safety information is available, is the umbrella trial concept as already successfully established by the I-SPY consortium (77,78,82) or WSG ADAPT (83).
Conclusions
As more genomic data and information on mutations are added today, prospective trials should open wide screening tests. Then, patients can expect to benefit from the identification of the specific driver mutations of their tumor and the related drug combination. No general consensus for targeted drug prescription will be then possible as individual tumor molecular specificities and the tumor heterogeneity will drive the therapeutic choice. Aside from clinical practice, large scale molecular testing will also aid drug development but requires coherent concepts for testing and downstream trials, which can only be performed by close national and international collaboration between all stakeholders. Moreover, novel trial concepts such trials including several tumor entities or umbrella trials with several biology-based sub-protocols supported by national and international research consortia will also speed up progress in this area.
Acknowledgements
Disclosure: NH has received honoraria for lectures from Genomic Health and obtained research funding from Genomic Health and NanoString. SDS declares that she has no interest to disclose related to the publication of this manuscript.
References
- Levi F, Bosetti C, Lucchini F, et al. Monitoring the decrease in breast cancer mortality in Europe. Eur J Cancer Prev 2005;14:497-502. [PubMed]
- Jemal A, Thun MJ, Ries LA, et al. Annual report to the nation on the status of cancer, 1975-2005, featuring trends in lung cancer, tobacco use, and tobacco control. J Natl Cancer Inst 2008;100:1672-94. [PubMed]
- Eroles P, Bosch A, Perez-Fidalgo JA, et al. Molecular biology in breast cancer: intrinsic subtypes and signaling pathways. Cancer Treat Rev 2012;38:698-707. [PubMed]
- Dawson SJ, Rueda OM, Aparicio S, et al. A new genome-driven integrated classification of breast cancer and its implications. EMBO J 2013;32:617-28. [PubMed]
- Ellis IO, Galea M, Broughton N, et al. Pathological prognostic factors in breast cancer. II. Histological type. Relationship with survival in a large study with long-term follow-up. Histopathology 1992;20:479-89. [PubMed]
- Perou CM, Sorlie T, Eisen MB, et al. Molecular portraits of human breast tumours. Nature 2000;406:747-52. [PubMed]
- Sorlie T, Tibshirani R, Parker J, et al. Repeated observation of breast tumor subtypes in independent gene expression data sets. Proc Natl Acad Sci U S A 2003;100:8418-23. [PubMed]
- Prat A, Parker JS, Karginova O, et al. Phenotypic and molecular characterization of the claudin-low intrinsic subtype of breast cancer. Breast Cancer Res 2010;12:R68. [PubMed]
- Perou CM, Parker JS, Prat A, et al. Clinical implementation of the intrinsic subtypes of breast cancer. Lancet Oncol 2010;11:718-9; author reply 20-1. [PubMed]
- Prat A, Perou CM. Deconstructing the molecular portraits of breast cancer. Mol Oncol 2011;5:5-23. [PubMed]
- Prat A, Adamo B, Cheang MC, et al. Molecular characterization of basal-like and non-basal-like triple-negative breast cancer. Oncologist 2013;18:123-33. [PubMed]
- Lehmann BD, Bauer JA, Chen X, et al. Identification of human triple-negative breast cancer subtypes and preclinical models for selection of targeted therapies. J Clin Invest 2011;121:2750-67. [PubMed]
- Harbeck N, Rody A. Lost in translation? Estrogen receptor status and endocrine responsiveness in breast cancer. J Clin Oncol 2012;30:686-9. [PubMed]
- Harris L, Fritsche H, Mennel R, et al. American Society of Clinical Oncology 2007 update of recommendations for the use of tumor markers in breast cancer. J Clin Oncol 2007;25:5287-312. [PubMed]
- Goldhirsch A, Wood WC, Coates AS, et al. Strategies for subtypes--dealing with the diversity of breast cancer: highlights of the St. Gallen International Expert Consensus on the Primary Therapy of Early Breast Cancer 2011. Ann Oncol 2011;22:1736-47. [PubMed]
- Rodenhiser DI, Andrews JD, Vandenberg TA, et al. Gene signatures of breast cancer progression and metastasis. Breast Cancer Res 2011;13:201. [PubMed]
- Hamilton-Burke W, Coleman L, Cummings M, et al. Phosphorylation of estrogen receptor beta at serine 105 is associated with good prognosis in breast cancer. Am J Pathol 2010;177:1079-86. [PubMed]
- O’Hara J, Vareslija D, McBryan J, et al. AIB1:ERalpha transcriptional activity is selectively enhanced in aromatase inhibitor-resistant breast cancer cells. Clin Cancer Res 2012;18:3305-15. [PubMed]
- Santen RJ, Fan P, Zhang Z, et al. Estrogen signals via an extra-nuclear pathway involving IGF-1R and EGFR in tamoxifen-sensitive and -resistant breast cancer cells. Steroids 2009;74:586-94. [PubMed]
- Ross-Innes CS, Stark R, Teschendorff AE, et al. Differential oestrogen receptor binding is associated with clinical outcome in breast cancer. Nature 2012;481:389-93. [PubMed]
- Martin HL, Smith L, Tomlinson DC. Multidrug-resistant breast cancer: current perspectives. Breast Cancer 2014;6:1-13. [PubMed]
- Geyer FC, Weigelt B, Natrajan R, et al. Molecular analysis reveals a genetic basis for the phenotypic diversity of metaplastic breast carcinomas. J Pathol 2010;220:562-73. [PubMed]
- Navin N, Krasnitz A, Rodgers L, et al. Inferring tumor progression from genomic heterogeneity. Genome Res 2010;20:68-80. [PubMed]
- Navin N, Kendall J, Troge J, et al. Tumour evolution inferred by single-cell sequencing. Nature 2011;472:90-4. [PubMed]
- Eccles SA, Aboagye EO, Ali S, et al. Critical research gaps and translational priorities for the successful prevention and treatment of breast cancer. Breast Cancer Res 2013;15:R92. [PubMed]
- Viedma-Rodríguez R, Baiza-Gutman L, Salamanca-Gómez F, et al. Mechanisms associated with resistance to tamoxifen in estrogen receptor-positive breast cancer Oncol Rep 2014. [Epub ahead of print]. [PubMed]
- Shah NR, Chen H. MicroRNAs in pathogenesis of breast cancer: Implications in diagnosis and treatment. World J Clin Oncol 2014;5:48-60. [PubMed]
- Byler S, Goldgar S, Heerboth S, et al. Genetic and epigenetic aspects of breast cancer progression and therapy. Anticancer Res 2014;34:1071-7. [PubMed]
- Ellis MJ. Mutational analysis of breast cancer: guiding personalized treatments. Breast 2013;22 Suppl 2:S19-21. [PubMed]
- Ellis MJ, Perou CM. The genomic landscape of breast cancer as a therapeutic roadmap. Cancer Discov 2013;3:27-34. [PubMed]
- Wheeler DA, Wang L. From human genome to cancer genome: the first decade. Genome Res 2013;23:1054-62. [PubMed]
- Cancer Genome Atlas Network. Comprehensive molecular portraits of human breast tumours. Nature 2012;490:61-70. [PubMed]
- Stephens PJ, McBride DJ, Lin ML, et al. Complex landscapes of somatic rearrangement in human breast cancer genomes. Nature 2009;462:1005-10. [PubMed]
- Pleasance ED, Cheetham RK, Stephens PJ, et al. A comprehensive catalogue of somatic mutations from a human cancer genome. Nature 2010;463:191-6. [PubMed]
- Chin L, Hahn WC, Getz G, et al. Making sense of cancer genomic data. Genes Dev 2011;25:534-55. [PubMed]
- Quail MA, Smith M, Coupland P, et al. A tale of three next generation sequencing platforms: comparison of Ion Torrent, Pacific Biosciences and Illumina MiSeq sequencers. BMC Genomics 2012;13:341. [PubMed]
- Khurana E, Fu Y, Colonna V, et al. Integrative annotation of variants from 1092 humans: application to cancer genomics. Science 2013;342:1235587. [PubMed]
- Wood LD, Parsons DW, Jones S, et al. The genomic landscapes of human breast and colorectal cancers. Science 2007;318:1108-13. [PubMed]
- Maher CA, Kumar-Sinha C, Cao X, et al. Transcriptome sequencing to detect gene fusions in cancer. Nature 2009;458:97-101. [PubMed]
- Shah SP, Morin RD, Khattra J, et al. Mutational evolution in a lobular breast tumour profiled at single nucleotide resolution. Nature 2009;461:809-13. [PubMed]
- Tuch BB, Laborde RR, Xu X, et al. Tumor transcriptome sequencing reveals allelic expression imbalances associated with copy number alterations. PLoS One 2010;5:e9317. [PubMed]
- Rehm HL. Disease-targeted sequencing: a cornerstone in the clinic. Nat Rev Genet 2013;14:295-300. [PubMed]
- Bernstein BE, Birney E, Dunham I, et al. An integrated encyclopedia of DNA elements in the human genome. Nature 2012;489:57-74. [PubMed]
- Malinowsky K, Wolff C, Ergin B, et al. Deciphering signaling pathways in clinical tissues for personalized medicine using protein microarrays. J Cell Physiol 2010;225:364-70. [PubMed]
- International Cancer Genome Consortium, Hudson TJ, Anderson W, et al. International network of cancer genome projects. Nature 2010;464:993-8. [PubMed]
- Lawrence MS, Stojanov P, Polak P, et al. Mutational heterogeneity in cancer and the search for new cancer-associated genes. Nature 2013;499:214-8. [PubMed]
- Garraway LA, Lander ES. Lessons from the cancer genome. Cell 2013;153:17-37. [PubMed]
- Ma CX, Ellis MJ. The Cancer Genome Atlas: clinical applications for breast cancer. Oncology (Williston Park) 2013;27:1263-9, 1274-9.
- Melchor L, Benitez J. The complex genetic landscape of familial breast cancer. Hum Genet 2013;132:845-63. [PubMed]
- Michailidou K, Hall P, Gonzalez-Neira A, et al. Large-scale genotyping identifies 41 new loci associated with breast cancer risk. Nat Genet 2013;45:353-61, 361e1-2.
- Sakoda LC, Jorgenson E, Witte JS. Turning of COGS moves forward findings for hormonally mediated cancers. Nat Genet. 2013;45:345-8. [PubMed]
- Tabchy A, Ma CX, Bose R, et al. Incorporating genomics into breast cancer clinical trials and care. Clin Cancer Res 2013;19:6371-9. [PubMed]
- Ciruelos Gil EM. Targeting the PI3K/AKT/mTOR pathway in estrogen receptor-positive breast cancer. Cancer Treat Rev 2014. [Epub ahead of print]. [PubMed]
- Ferrario C, Batist G. Advances in the approach to novel drug clinical development for breast cancer. Expert Opin Drug Discov 2014;9:647-68. [PubMed]
- Kumar RD, Chang LW, Ellis MJ, et al. Prioritizing Potentially Druggable Mutations with dGene: An Annotation Tool for Cancer Genome Sequencing Data. PLoS One 2013;8:e67980. [PubMed]
- Ellis MJ, Ding L, Shen D, et al. Whole-genome analysis informs breast cancer response to aromatase inhibition. Nature 2012;486:353-60. [PubMed]
- Herschkowitz JI, Simin K, Weigman VJ, et al. Identification of conserved gene expression features between murine mammary carcinoma models and human breast tumors. Genome Biol 2007;8:R76. [PubMed]
- Bartlett JM, Brookes CL, Robson T, et al. Estrogen receptor and progesterone receptor as predictive biomarkers of response to endocrine therapy: a prospectively powered pathology study in the Tamoxifen and Exemestane Adjuvant Multinational trial. J Clin Oncol 2011;29:1531-8. [PubMed]
- Honma N, Horii R, Iwase T, et al. Clinical importance of estrogen receptor-beta evaluation in breast cancer patients treated with adjuvant tamoxifen therapy. J Clin Oncol 2008;26:3727-34. [PubMed]
- Yan Y, Li X, Blanchard A, et al. Expression of both estrogen receptor-beta 1 (ER-beta1) and its co-regulator steroid receptor RNA activator protein (SRAP) are predictive for benefit from tamoxifen therapy in patients with estrogen receptor-alpha (ER-alpha)-negative early breast cancer (EBC). Ann Oncol 2013;24:1986-93. [PubMed]
- De Amicis F, Thirugnansampanthan J, Cui Y, et al. Androgen receptor overexpression induces tamoxifen resistance in human breast cancer cells. Breast Cancer Res Treat 2010;121:1-11. [PubMed]
- Garay JP, Park BH. Androgen receptor as a targeted therapy for breast cancer. Am J Cancer Res 2012;2:434-45. [PubMed]
- Hickey TE, Robinson JL, Carroll JS, et al. Minireview: The androgen receptor in breast tissues: growth inhibitor, tumor suppressor, oncogene? Mol Endocrinol 2012;26:1252-67. [PubMed]
- Gnant M. Overcoming endocrine resistance in breast cancer: importance of mTOR inhibition. Expert Rev Anticancer Ther 2012;12:1579-89. [PubMed]
- Baselga J, Campone M, Piccart M, et al. Everolimus in postmenopausal hormone-receptor-positive advanced breast cancer. N Engl J Med 2012;366:520-9. [PubMed]
- Banerji S, Cibulskis K, Rangel-Escareno C, et al. Sequence analysis of mutations and translocations across breast cancer subtypes. Nature 2012;486:405-9. [PubMed]
- Stephens PJ, Tarpey PS, Davies H, et al. The landscape of cancer genes and mutational processes in breast cancer. Nature 2012;486:400-4. [PubMed]
- Curtis C, Shah SP, Chin SF, et al. The genomic and transcriptomic architecture of 2,000 breast tumours reveals novel subgroups. Nature 2012;486:346-52. [PubMed]
- Shah SP, Roth A, Goya R, et al. The clonal and mutational evolution spectrum of primary triple-negative breast cancers. Nature 2012;486:395-9. [PubMed]
- Harbeck N, Sotlar K, Wuerstlein R, et al. Molecular and protein markers for clinical decision making in breast cancer: today and tomorrow. Cancer Treat Rev 2014;40:434-44. [PubMed]
- Gökmen-Polar Y, Badve S. Molecular profiling assays in breast cancer: are we ready for prime time? Oncology (Williston Park) 2012;26:350-7, 361.
- Zujewski JA, Kamin L. Trial assessing individualized options for treatment for breast cancer: the TAILORx trial. Future Oncol 2008;4:603-10. [PubMed]
- Sparano JA. TAILORx: trial assigning individualized options for treatment (Rx). Clin Breast Cancer 2006;7:347-50. [PubMed]
- Gluz, Kreipe H, Dehenhardt T, et al. Prospective Comparison of Risk Assessment Tools in Early Breast Cancer (Recurrence Score®, uPA/PAI-1, Central Grade, and Luminal Subtypes): Final Correlation Analysis from the Phase III WSG planB Trial. San Antonio Breast Cancer symposium 2011:Abstract S4-3.
- Gluz O, Hofmann D, Kates RE, et al. ADAPT - Adjuvant Dynamic marker-Adjusted Personalized Therapy trial optimizing risk assessment and therapy response prediction in early breast cancer. Cancer Res 2012;72:Abstract nr OT3-3-02.
- Berry DA. Adaptive clinical trials in oncology. Nat Rev Clin Oncol 2011;9:199-207. [PubMed]
- Esserman LJ, Berry DA, DeMichele A, et al. Pathologic complete response predicts recurrence-free survival more effectively by cancer subset: results from the I-SPY 1 TRIAL--CALGB 150007/150012, ACRIN 6657. J Clin Oncol 2012;30:3242-9. [PubMed]
- Esserman LJ, Berry DA, Cheang MC, et al. Chemotherapy response and recurrence-free survival in neoadjuvant breast cancer depends on biomarker profiles: results from the I-SPY 1 TRIAL (CALGB 150007/150012; ACRIN 6657). Breast Cancer Res Treat 2012;132:1049-62. [PubMed]
- Barker AD, Sigman CC, Kelloff GJ, et al. I-SPY 2: an adaptive breast cancer trial design in the setting of neoadjuvant chemotherapy. Clin Pharmacol Ther 2009;86:97-100. [PubMed]
- Printz C. I-SPY 2 may change how clinical trials are conducted: researchers aim to accelerate approvals of cancer drugs. Cancer 2013;119:1925-7. [PubMed]
- André F, Bachelot T, Commo F, et al. Comparative genomic hybridisation array and DNA sequencing to direct treatment of metastatic breast cancer: a multicentre, prospective trial (SAFIR01/UNICANCER). Lancet Oncol 2014;15:267-74. [PubMed]
- Lin C, Buxton MB, Moore D, et al. Locally advanced breast cancers are more likely to present as Interval Cancers: results from the I-SPY 1 TRIAL (CALGB 150007/150012, ACRIN 6657, InterSPORE Trial). Breast Cancer Res Treat 2012;132:871-9. [PubMed]
- Hofmann D, Nitz U, Gluz O, et al. WSG ADAPT - adjuvant dynamic marker-adjusted personalized therapy trial optimizing risk assessment and therapy response prediction in early breast cancer: study protocol for a prospective, multi-center, controlled, non-blinded, randomized, investigator initiated phase II/III trial. Trials 2013;14:261. [PubMed]