Statistical principles for omics-based clinical trials
Introduction
Omics technologies that generate a large amount of molecular data about biospecimens have the potential to provide accurate predictions of a patient’s prognosis and predictions of their response to a specific treatment regime. The idea of omics-based tests is that distinct subgroups of patients can be identified using multi-dimensional molecular data and therefore treatment decisions can be personalized to that subgroup. An omics-based test can guide the decisions to treat or not to treat and help identify the particular therapy most likely to work. The challenge is to identify and demonstrate definitively that the use of an omics-based test improves clinical outcomes in a patient population.
An omics-based test can be used to predict a patient’s prognosis, which is their expected clinical outcome. A test that provides accurate predictions of prognosis, regardless of treatment, is referred to as prognostic. A predictive omics-based test is one that accurately predicts disease outcomes with the application of specific interventions. Predictive markers are therefore useful for the selection among two or more treatment options. Statistically, a prognostic omics-based test is strongly associated with clinical outcome and a predictive omics-based test modifies the association between treatment and clinical outcome (interaction). High dimensional omics data can be used to identify specific molecular targets as potential mechanisms for drug development; however the use of omics technologies for drug development is beyond the scope of this review.
The path from development to definitively evaluating an omics-based test for prognosis or prediction of treatment response is long and arduous. Often, the end goal is to develop a test suitable for use in a clinical trial for guiding treatment. The oncology literature is full of reports that develop and/or evaluate omics-based tools for prognosis and prediction. Developing a simple test based on high-dimensional omics data can be complex and requires careful application and interpretation of statistical methods. Definitive evaluation of a prognostic or predictive omics-based test is costly and rife with methodological pitfalls. We aim to review the relevant issues, providing the resources to ask the right questions when critically weighing the evidence presented in a report of an omics-based study. Figure 1 gives an overview of the omics test development process. Ultimately, for a practicing oncologist the question is: “Is this omics-based test something I want to use to improve outcomes of my patients?”
The long road to implementing a test in a practice starts with analytical validation of the assay involved, that is, demonstrating that the omics-based assay accurately and reproducibly measures the molecular quantities. After the assay performance is established, development of the test and preliminary evaluation are necessary. Those involve reducing the high-dimensional data into a one-dimensional quantity that will be used to make a decision. This one-dimensional quantity is often a risk score: an estimate of the probability of a specific clinical outcome. It is necessary to establish the clinical validity of this risk score, that is, to demonstrate that the risk score is independently and strongly associated with clinical outcome. Care must be taken to completely separate the development of the risk score from the evaluation, otherwise estimates can be optimistically biased. Finally, the risk score must be translated into a binary decision, often using a threshold. It remains to demonstrate that the use of the test to make this decision improves patient outcomes. Figure 2 illustrates the types of studies that are involved in the omics test development and evaluation process.
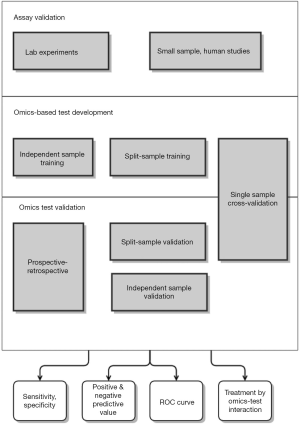
The following sections specify questions to be considered while reading a report of an omics-based clinical study. We review the importance of such questions, and common pitfalls to watch for. In the planning or reporting of an omics-based trial, answers to these questions should be made clear to the reader. Formal efforts to guide reporting have been developed, such as the REMARK checklist (1), the GRIPS statement (2), and an omics checklist (3).
Terminology
An omics-based test, or simply an omics test, is a mapping from the set of features on the omics assay to a single number. This number can be a binary value, such as good or poor prognosis, or it can provide a continuous scale, such as a risk score. It must be feasible to perform the test on an individual patient basis, by measuring the omics assay on the individual’s tissue. The assay generates a multitude of measurements, which we will refer to as features, and then fixed mathematical calculations are done to transform the many features into the single test value. Examples of such features are gene expression values, protein expression measurements, or genetic mutations. We use the term specimens to refer to individual patient tissues or fluids on which the assay would be run. We use the term sample in the statistical sense, meaning a group of individuals randomly selected from a population.
Investigators determine the way that the mathematical calculations are done in the development phase. Often, there is a complete sample which is randomly allocated into development and validation sub-samples. These are also sometimes referred to as training and test sets of samples. At the end of the development phase, the model for the mathematical calculations is fixed and the algorithm is locked down.
That model is evaluated definitively in the validation phase in a completely independent sample. In order for the validation to be unbiased and definitive, it is imperative that no information from the validation sample leaks into the development phase. The validation should mimic realistic clinical use as much as possible, and that means that no further refinement to the test is allowed based on the observed results.
A given study may cover only one of the many steps and the entire process may be reported across multiple peer-reviewed publications. For example, at least four key publications were devoted to the development and validation of Oncotype DX, which is a commercially available omics-based prognostic test used in breast cancer (4-7).
What is the intended clinical use?
As with all clinical studies, the end goal is to improve patient care. Omics studies are no different, and a clear statement of the intended clinical use of the omics-test should be prominent. Carefully describing the context for the use of the assay determines the type of study needed to develop and validate it. The intended use of the assay also provides an overarching context in which to interpret the population under study, the assay measurements, and the statistical methods.
Omics-based tests in oncology generally are used for one of two clinical purposes: prognosis or prediction of treatment response. A prognostic test is used to predict the likely clinical outcome of a patient. Often a prognosis is used to guide management of the disease. Patients with a very good prognosis may opt not to receive any treatment, while patients with a poor prognosis may opt for more aggressive treatment. An omics-based prognostic test that is currently used in practice is EndoPredict, which is used to predict the risk of recurrence in ER-positive, HER2-negative breast cancer (8). For patients with a low risk of recurrence, it has been demonstrated that the risks of chemotherapy do not outweigh the benefits. Prognostic tests are clinically useful for guiding general disease management.
Predictive tests are most useful for selecting patient populations for treatment with specific targeted therapies. This presumes the existence of a particular molecular targeted therapy. The predictive test is used to identify patients who will benefit from the targeted therapy. Predictive tests are generally based on only one or a few molecular characteristics that the therapy targets. For example, HER-2 is a gene that is associated with a more aggressive form of breast cancer. Trastuzumab is a drug that specifically targets HER-2 and has been shown to be effective in HER-2 positive breast cancer (9). While targeted therapies generally target only one molecular characteristic, omics assays can be used to identify molecular targets for less well-understood drugs. However, most successful targeted therapies have associated predictive tests that were developed based on the underlying biology rather than a broad search over a large number of molecular features (10).
What is the patient population of interest?
Along with the intended clinical use, a report should have a clear statement of the intended population in which the test is being evaluated. This could be broad or quite specific. For the omics test to be useful, it must provide sufficient information above and beyond the standard of care in the target patient population. The distribution of the omics test and the expected benefit in the population should be clearly specified in advance.
The expected benefit of a new omics-based test could differ greatly by patient population. For instance, a prognostic test has more potential for benefit in stage 2 breast cancer than it does in stage 1 breast cancer, as the prognosis for stage 1 is already very good. Evaluating an omics-based test in a broad population that encompasses multiple stages or multiple disease types can be difficult, as the test must provide more information beyond that provided by standard clinical and pathological factors.
Are the assay methods and laboratory procedures valid?
Analytical validation of an assay involves evaluating the performance of the measurement in terms of accuracy, bias, and precision under a variety of conditions. Conditions refer to pre-analytic factors such as specimen quality, specimen collection, storage, and processing procedures, and technical aspects such as laboratory technician and batch effects from reagent lots or other assay materials. The high-dimensional nature of omics data makes it very difficult to assess each of the hundreds or thousands of outputs from a single assay. In developing an omics-based signature that only uses a subset of the components of a high-dimensional assay, one can analytically validate the final signature alone. However, prior to developing the signature, one must develop detailed standard operating procedures for specimen handling and processing to ensure a baseline level of validity.
Study reports must state what type of specimens are used and whether the test is applied to formalin-fixed paraffin embedded (FFPE) or only fresh-frozen tissue. Most omics-based assays require a minimum percentage of tumors to be successful. A report should clearly state what criteria were used to screen tissue specimens prior to running the assay. Generally this involves criteria for the rejection of poor-quality specimens on the basis of percent tumor, percent necrosis, or some other marker of tissue quality.
Molecular assays can successfully be run on decades of old FFPE tissue (11). However, factors involved in the tissue processing and storage can impact the analyte extraction and quality (12-14). Relatively little attention has been given to studying the downstream effects of pre-analytic factors on the individual omics features. In one study, the authors observe that older FFPE specimens tended to have lower expression levels and that this effect was different for different genes. The investigators modified their assay to account for this differential effect (15). Due to the high dimensionality of omics assays, a small amount of bias on each feature can translate into large errors when incorporating data from hundreds or thousands of features into a single continuous measurement. Therefore it is important to assess the impact of processing on the individual features in addition to the overall test.
In addition to processing and storage, technical aspects of an assay can impact the final results in a predictable way (16,17). There could be technical effects, differences due to reagent lots, and other batch effects. Such batch effects are commonly recognized yet often ignored in high-dimensional assays (18). Efforts should be made to measure the impact of these technical aspects and minimize them to the greatest extent possible. The way in which specimens are assayed should be randomized to prevent confounding batch effects with the clinical outcome. Development and validation samples are sometimes run in the same batch or with the same lot of technical aspects. This does minimize batch effects; however, it can provide an overly optimistic assessment of the test, because in clinical use, running specimens all in the same batch is not always an option.
Similar to developing criteria for rejection of tissue specimens, in omics settings, criteria should be developed for the rejection of individual features (e.g., genes, proteins) prior to the development of the test, if problems cannot be resolved through improved assay procedures. Features that do not pass the pre-specified quality metrics should be removed from consideration from the final test. Note that this feature processing step does not involve any clinical outcome measurements. As a concrete example, in the development of the gene expression based test EndoPredict, investigators chose to exclude probe locations that have a dynamic range less than 2, probes for which fewer than 1% of the specimens had calls, and probes whose 90th percentile was less than 350 units (8). Quality control steps of this nature can ensure a more robust and reproducible development of the test.
Even with careful quality control and a locked down standard operating procedure, it is difficult to completely eliminate the effects of technical factors on assay results. Therefore, when designing the development phase, the investigator must be mindful not to confound technical factors with the clinical outcomes. The problem of batch effects is widespread in omics research and can lead to spurious or irreproducible results (18,19). As an extreme example, consider developing an omics-based test to predict a binary clinical response. In the development phase, all of the assays for the clinical responders were run using reagent A, while all of the assays for the clinical non-responders were run using reagent B. If it were the case that the reagent has a significant effect on the assays, then the development phase would then lead to what seems like an excellent predictor, except it is predicting the batch effect rather than the clinical outcome.
Are the statistical methods for test development appropriate?
Once the analytical validity of the omics assay is established, the features are translated into a binary classification, a multi-category classification, or a continuous risk score. The methods used to perform this translation must be carefully evaluated to ensure that the features of the omics assay have been properly translated into a clinically meaningful quantity.
Unfortunately, a common approach to developing prediction models is to use cluster analysis of omics features, ignoring the clinical outcome among the development samples. Cluster analysis is a class of methods that is used to partition individuals into groups based on the similarities or differences among the omics features (20). The number of groups or clusters is not known in advance, but rather it is data dependent. Clustering is unsupervised in the sense that discovery of the groups is done without regard to the clinical outcome. The resulting clusters are not designed to provide valid information regarding a prognosis or prediction of response to therapy (21). A common argument in favor of clustering is that it identifies biologically distinct groups. However, the groups are identified using a statistical algorithm and the biological relevance is only considered post hoc. For developing omics-based prognostic or predictive tests, it is better to use supervised statistical methods which are designed to address those aims, outlined below.
Often, there are more features measured than there are patients in the sample. In such high-dimensional settings, it is required to identify a subset of the features that will be used in the final multivariable mathematical model. There are two broad statistical approaches to this problem: filtering and regularization.
Filtering is a statistical approach where univariate methods are applied to each of the many omics features in turn. Typically, the univariate method involves estimating the association of the feature with the clinical outcome. Then, a criterion, chosen in advance or selected using cross-validation, is applied to the statistic to select a subset of features. For example, suppose an investigator is interested in developing a gene expression based test to predict clinical response to a new therapy. For each of the 1,000 gene expression features that are available, one could compute a t-statistic comparing the expression levels for responders versus non-responders. Genes with t-test P values greater than 0.0001 could be filtered out, and the remaining ones used in a multivariable logistic regression model to predict response (22) describes a novel approach to filtering that is applied successfully to predict B-cell lymphoma subtypes using gene expression microarrays.
Regularization is an approach in which all of the features in consideration are entered into a special multivariable statistical model for prediction of the clinical outcome, even if there are more features than study participants. The special model includes a penalty component which encourages the model to remove completely or downplay the impact of features that are not relevant. There are various types of penalty functions each with different properties, such as the lasso (23), the ridge penalty (24), the elastic net (25), and others (20). Each type of penalty term contains at least one tuning parameter, which may be pre-specified or selected using cross-validation.
Each type of approach has its merits, and within each class there are a variety of specific models to choose from. It is difficult to determine what method will work best in advance. Instead of selecting a single model to use, multiple models can be averaged to improve prediction (26). This approach, called Bayesian model averaging, has proven successful in different applications, including prediction of cancer subtypes (27). It is more common, however, to try several different methods then select the one that performs the best on a small subset of the development sample. This is appropriate as long as the model selection is done entirely separately from the final validation sample. Leaking of information from the validation data into the model selection process can cause bias in insidious ways.
In many oncology settings, such as pediatric cancers, patients and specimens may be very sparse. It may be difficult to enroll sufficient subjects to develop an omics test and then perform preliminary validation on an independent sample. In that case, cross-validation can provide an unbiased estimate of prediction error, if done properly (20). Cross-validation refers to the idea that a model can be evaluated in the same sample in which it is developed. Similar to a split sample approach, in cross-validation only a small portion of the sample is left out at a time. The model is estimated on the remaining samples, and the performance is evaluated on the left out independent portion. This process is repeated many times to get a more precise estimate of the performance (28) describe a cross validated trial design tailored for sparse data settings.
In doing cross-validation, it is important to validate the entire model estimation process, not only part of it. For that reason it is often best to avoid complex test development procedures involving multiple, data-driven selection steps and/or tuning parameters so as not to mistakenly leak information from the validation data (29). More complex procedures can also lead to overfitting, in which the model identifies random noise in the data, rather than a true signal of clinical use.
How is the validation study designed?
Once the mathematical model is estimated and completely locked down based on the development sample, a study to definitively evaluate the locked-down test should be designed to address the clinical use in the population of interest. The key characteristic of the evaluation study or sample is that it is completely independent of the sample on which the test was developed. Once the test is defined and locked down, no information from the evaluation sample can be used to change the features of the test. The evaluation sample could be a randomly selected subgroup from the same parent study as the development set, or it could be from a separate study altogether conducted in the same population. As long as the population and the intended clinical use are clearly defined, the evaluation can be done definitively.
A definitive evaluation can be done retrospectively, meaning that stored specimens are selected from a study that has completed. The omics assay is then run on the archived specimens and the locked down test is associated with the clinical outcomes, which have already been observed at the time of the assay measurement. This retrospective design can yield high quality evidence of the test’s characteristics, if it is done carefully. It is imperative to develop a protocol for the study in which the omics test is clearly and completely defined, the main hypotheses are specified, and the assay standard operating procedures are detailed. The archived specimens need to come from a study or trial with a well-defined population under study, not a convenience sample. Sample size and power calculations should be done with the same rigor as they are in a clinical trial. Such a study, called “prospective-retrospective”, can yield a high degree of evidence in the evaluation of an omics-test, and with great efficiency (30).
Alternatively, prospective studies can be used to evaluate an omics-based test by performing the assay at the start of the study and then following patients for clinical outcomes. Again, all of the key details need to be specified up front in the protocol. The details of the study design should be tailored to appropriately answer the clinical question definitively. Several review articles are available that describe the potential study designs for the evaluation of prognostic and predictive tests (31-34). Details of specific designs and statistical approaches are available for Bayesian approaches (35,36), adaptive or sequential approaches (37,38), and standard frequentist approaches (39-41). This has been and continues to be an active research area in statistics, which means that designs are continually evolving to appropriately address the clinical question in the population of interest.
In the design, careful consideration should be given to the study power and sample size. A prospective study in which patients may be undergoing painful biopsies or unnecessary treatment should not be done unless there is a high probability of definitively answering the scientific question. Likewise, precious archived specimens should not be wasted on a retrospective study that is under-powered. Most standard statistical tools for power analysis apply to prognostic tests, however predictive or therapy-guiding omics-based tests require a different approach. Many protocols for predictive tests are powered to detect the interaction effect between the treatment and the test (42). The existence of a treatment-by-test interaction is necessary but not sufficient for the test to be useful in guiding therapy (43). Tools for power and sample size analysis have been designed to specifically address the question of a qualitative interaction (44-46).
Are the development and validation samples strictly separated?
This issue has been discussed in previous sections, yet this error occurs so frequently that it requires an in-depth discussion. The evaluation sample for the assessment of a prognostic or predictive test needs to be completely independent from the development sample. This is especially true for omics-based tests, whose development is often complex. Any information from the evaluation sample that leaks into the development sample can bias the results, making tests appear better than they truly are.
Leaking information between samples can happen in subtle ways. Sometimes, part of the model development process is repeated on the validation data. This is called partial resubstitution (21). For example, a common model development approach is to first filter a subset of 50 genes from a larger set of 450,000 based on their observed association with the outcome. Then, the 50 genes are put into a regression model to develop a single risk score. Occasionally, investigators will perform the filtering on the development sample and then re-estimate the regression model using the combined development and validation samples. This gives overly optimistic estimates of the performance of the algorithm. Partial resubstitution can be difficult to detect when the model development is more complex, and if cross-validation is used to estimate the performance.
In settings where relatively few samples are available, cross-validation is an efficient and valid approach to estimating performance (47). The key point whether using the split sample approach or cross validation is that the entire model building process must be validated. Even informal checks of the model on the validation sample, such as viewing survival curve plots, prior to locking down the model can unknowingly cause bias.
Are the statistical methods appropriate for test validation?
To assess the value of an omics-based test for prognosis or prediction we need to estimate the association between the test and the clinical outcome on an independent sample (the validation sample). Appropriate statistics are essential to measure this association. Often, investigators will report only the odds ratio (in the case of a binary clinical outcome) or the hazard ratio (for a time-to-event clinical outcome) for the omics test. The odds ratio or hazard ratio is insufficient to determine the clinical utility of an omics-based test (48). Ideally, a statistical method or set of statistical measures should be chosen to address the intended clinical use of the test.
For a prognostic test, how often does the test correctly predict recurrence (true positives) and how often does it correctly predict non-recurrence (true negatives)? It is imperative to report both of these measures, also known as the sensitivity and specificity; because one can correctly predict all true positives simply by predicting that all cases are positive. Is the performance good enough to change clinical practice? Patients want to know the likelihood of recurrence given their test results; this is called the positive predictive value. If the likelihood of recurrence is very low overall in the population, as it is in stage 1 breast cancer, then a new test must be highly informative for it to be practice changing. For continuous-valued tests, extensions to these measures exist and can be visualized with the receiver operating characteristic (ROC) curve. Furthermore, extensions also exist for time-to-event clinical outcomes such as overall or progression free survival (49) provides an excellent reference for statistical measures for the evaluation of diagnostic and prognostic tests.
In recent years, a number of potentially misleading statistical methods have crept into common usage. The net reclassification index (NRI) and its sibling, the integrated discrimination improvement (IDI), were designed to assess the added value of a new test to existing criteria: the incremental value. For example, it is often of interest to determine whether a novel omics-based test adds value to standard clinical and pathological features. The NRI and IDI specifically evaluate whether the novel component enhances the differentiation of patients into risk groups. This does not address the question whether the novel component correctly classifies patients (50,51). Others have noted additional problems with the statistical operating characteristics of the method, most importantly, that it is not a valid measure (52-54). More fundamentally, it is not clear what clinical question this measure addresses; does it matter if patients are classified differently if we don’t know whether they are classified correctly?
A proper evaluation of an omics-based test takes a comprehensive and pre-specified approach to address the intended clinical use. For predictive omics-based tests to guide therapy, a rigorous approach to evaluation has been described, along with statistical software for general use (55). This continues to be an active area of biostatistical research.
Concluding remarks
The use of omics-based tests for prognosis, predicting, and therapy selection is steadily increasing in oncology. Careful evaluation of the quality of studies by consumers of the clinical oncology literature is imperative to provide a high level of patient care. Formal sets of reporting criteria exist for the producers of such literature (1-3,56) and these are also useful for readers to be aware of. We hope that the discussion here has brought attention to the issues from the readers’ perspective and will help promote critical evaluation of the relevant literature.
Acknowledgements
None.
Footnote
Conflicts of Interest: The author has no conflicts of interest to declare.
References
- Altman DG, McShane LM, Sauerbrei W, et al. Reporting recommendations for tumor marker prognostic studies (REMARK): explanation and elaboration. BMC Med 2012;10:51. [PubMed]
- Janssens AC, Ioannidis JP, Bedrosian S, et al. Strengthening the reporting of genetic risk prediction studies (GRIPS): explanation and elaboration. Eur J Clin Invest 2011;41:1010-35. [PubMed]
- McShane LM, Cavenagh MM, Lively TG, et al. Criteria for the use of omics-based predictors in clinical trials: explanation and elaboration. BMC Med 2013;11:220. [PubMed]
- Paik S, Shak S, Tang G, et al. A multigene assay to predict recurrence of tamoxifen-treated, node-negative breast cancer. N Engl J Med 2004;351:2817-26. [PubMed]
- Habel LA, Shak S, Jacobs MK, et al. A population-based study of tumor gene expression and risk of breast cancer death among lymph node-negative patients. Breast Cancer Res 2006;8:R25. [PubMed]
- Cronin M, Sangli C, Liu ML, et al. Analytical validation of the Oncotype DX genomic diagnostic test for recurrence prognosis and therapeutic response prediction in node-negative, estrogen receptor-positive breast cancer. Clin Chem 2007;53:1084-91. [PubMed]
- Solin LJ, Gray R, Baehner FL, et al. A multigene expression assay to predict local recurrence risk for ductal carcinoma in situ of the breast. J Natl Cancer Inst 2013;105:701-10. [PubMed]
- Filipits M, Rudas M, Jakesz R, et al. A new molecular predictor of distant recurrence in ER-positive, HER2-negative breast cancer adds independent information to conventional clinical risk factors. Clin Cancer Res 2011;17:6012-20. [PubMed]
- Fleeman N, Bagust A, Beale S, et al. Pertuzumab in combination with trastuzumab and docetaxel for the treatment of hER2 positive metastatic or locally recurrent unresectable breast Cancer. PharmacoEconomics 2015;33:13-23. [PubMed]
- Sawyers CL. The cancer biomarker problem. Nature 2008;452:548-52. [PubMed]
- Iwamoto KS, Mizuno T, Ito T, et al. Feasibility of using decades-old archival tissues in molecular oncology/epidemiology. Am J Pathol 1996;149:399-406. [PubMed]
- Srinivasan M, Sedmak D, Jewell S. Effect of fixatives and tissue processing on the content and integrity of nucleic acids. Am J Pathol 2002;161:1961-71. [PubMed]
- van Maldegem F, de Wit M, Morsink F, et al. Effects of processing delay, formalin fixation, and immunohistochemistry on RNA Recovery From Formalin-fixed Paraffin-embedded Tissue Sections. Diagn Mol Pathol 2008;17:51-8. [PubMed]
- Specht K, Richter T, Müller U, et al. Quantitative gene expression analysis in microdissected archival formalin-fixed and paraffin-embedded tumor tissue. Am J Pathol 2001;158:419-29. [PubMed]
- Cronin M, Pho M, Dutta D, et al. Measurement of gene expression in archival paraffin-embedded tissues: development and performance of a 92-gene reverse transcriptase-polymerase chain reaction assay. Am J Pathol 2004;164:35-42. [PubMed]
- Pennello GA. Analytical and clinical evaluation of biomarkers assays: when are biomarkers ready for prime time? Clin Trials 2013;10:666-76. [PubMed]
- Isler JA, Vesterqvist OE, Burczynski ME. Analytical validation of genotyping assays in the biomarker laboratory. Pharmacogenomics 2007;8:353-68. [PubMed]
- Leek JT, Scharpf RB, Bravo HC, et al. Tackling the widespread and critical impact of batch effects in high-throughput data. Nat Rev Genet 2010;11:733-9. [PubMed]
- Soneson C, Gerster S, Delorenzi M. Batch effect confounding leads to strong bias in performance estimates obtained by cross-validation. PLoS One 2014;9:e100335. [PubMed]
- Hastie T, Tibshirani R, Friedman J. eds. The Elements of Statistical Learning: Data Mining, Inference, and Prediction. Second Edition. New York: Springer, 2009.
- Simon R, Radmacher MD, Dobbin K, et al. Pitfalls in the use of DNA microarray data for diagnostic and prognostic classification. J Natl Cancer Inst 2003;95:14-8. [PubMed]
- Bair E, Tibshirani R. Semi-supervised methods to predict patient survival from gene expression data. PLoS Biol 2004;2:E108. [PubMed]
- Tibshirani R. Regression shrinkage and selection via the lasso. J Roy Stat Soc B 1996;58:267-88.
- Hoerl AE, Kennard RW. Ridge Regression: Biased Estimation for Nonorthogonal Problems. Technometrics 1970;12:55-67.
- Zou H, Hastie T. Regularization and variable selection via the elastic net. J Roy Stat Soc B 2005;67:301-20.
- Hoeting JA, Madigan D, Adrian E, et al. Bayesian Model Averaging: A Tutorial. Statistical science 1999;14:382-417.
- Yeung KY, Bumgarner RE, Raftery AE. Bayesian model averaging: development of an improved multi-class, gene selection and classification tool for microarray data. Bioinformatics 2005;21:2394-402. [PubMed]
- Freidlin B, Jiang W, Simon R. The cross-validated adaptive signature design. Clin Cancer Res 2010;16:691-8. [PubMed]
- McShane LM, Polley MY. Development of omics-based clinical tests for prognosis and therapy selection: the challenge of achieving statistical robustness and clinical utility. Clin Trials 2013;10:653-65. [PubMed]
- Simon RM, Paik S, Hayes DF. Use of archived specimens in evaluation of prognostic and predictive biomarkers. J Natl Cancer Inst 2009;101:1446-52. [PubMed]
- Sargent DJ, Conley BA, Allegra C, et al. Clinical trial designs for predictive marker validation in cancer treatment trials. J Clin Oncol 2005;23:2020-7. [PubMed]
- Baker SG, Kramer BS, Sargent DJ, et al. Biomarkers, subgroup evaluation, and clinical trial design. Discov Med 2012;13:187-92. [PubMed]
- Freidlin B, Korn EL. Biomarker enrichment strategies: matching trial design to biomarker credentials. Nat Rev Clin Oncol 2014;11:81-90. [PubMed]
- Mandrekar SJ, Sargent DJ. Clinical trial designs for predictive biomarker validation: theoretical considerations and practical challenges. J Clin Oncol 2009;27:4027-34. [PubMed]
- Brannath W, Zuber E, Branson M, et al. Confirmatory adaptive designs with Bayesian decision tools for a targeted therapy in oncology. Stat Med 2009;28:1445-63. [PubMed]
- Morita S, Yamamoto H, Sugitani Y. Biomarker-based Bayesian randomized phase II clinical trial design to identify a sensitive patient subpopulation. Stat Med 2014;33:4008-16. [PubMed]
- Freidlin B, Korn EL, Gray R. Marker Sequential Test (MaST) design. Clin Trials 2014;11:19-27. [PubMed]
- Jiang W, Freidlin B, Simon R. Biomarker-adaptive threshold design: a procedure for evaluating treatment with possible biomarker-defined subset effect. J Natl Cancer Inst 2007;99:1036-43. [PubMed]
- Denne JS, Pennello G, Zhao L, et al. Identifying a subpopulation for a tailored therapy: Bridging clinical efficacy from a laboratory-developed assay to a validated in vitro diagnostic test kit. Stat Biopharm Res 2014;6:78-88.
- Eng KH. Randomized reverse marker strategy design for prospective biomarker validation. Stat Med 2014;33:3089-99. [PubMed]
- Freidlin B, McShane LM, Polley MY, et al. Randomized phase II trial designs with biomarkers. J Clin Oncol 2012;30:3304-9. [PubMed]
- Peterson B, George SL. Sample size requirements and length of study for testing interaction in a 2 x k factorial design when time-to-failure is the outcome Control Clin Trials 1993;14:511-22. [corrected]. [PubMed]
- Polley MY, Freidlin B, Korn EL, et al. Statistical and practical considerations for clinical evaluation of predictive biomarkers. J Natl Cancer Inst 2013;105:1677-83. [PubMed]
- Gail M, Simon R. Testing for qualitative interactions between treatment effects and patient subsets. Biometrics 1985;41:361-72. [PubMed]
- Bayman EO, Chaloner K, Cowles MK. Detecting qualitative interaction: a Bayesian approach. Stat Med 2010;29:455-63. [PubMed]
- Mackey HM, Bengtsson T. Sample size and threshold estimation for clinical trials with predictive biomarkers. Contemp Clin Trials 2013;36:664-72. [PubMed]
- Lee S. Mistakes in validating the accuracy of a prediction classifier in high-dimensional but small-sample microarray data. Stat Methods Med Res 2008;17:635-42. [PubMed]
- Pepe MS, Janes H, Longton G, et al. Limitations of the odds ratio in gauging the performance of a diagnostic, prognostic, or screening marker. Am J Epidemiol 2004;159:882-90. [PubMed]
- Pepe MS, editor. The statistical evaluation of medical tests for classification and prediction. New York, NY: Oxford University Press, 2003.
- Hilden J, Gerds TA. A note on the evaluation of novel biomarkers: do not rely on integrated discrimination improvement and net reclassification index. Stat Med 2014;33:3405-14. [PubMed]
- Pepe MS. Problems with risk reclassification methods for evaluating prediction models. Am J Epidemiol 2011;173:1327-35. [PubMed]
- Pepe MS, Janes H, Li CI. Net risk reclassification p values: valid or misleading? J Natl Cancer Inst 2014;106:dju041. [PubMed]
- Kerr KF, Wang Z, Janes H, et al. Net reclassification indices for evaluating risk prediction instruments: a critical review. Epidemiology 2014;25:114-21. [PubMed]
- Hilden J. Commentary: On NRI, IDI, and "good-looking" statistics with nothing underneath. Epidemiology 2014;25:265-7. [PubMed]
- Janes H, Brown MD, Huang Y, et al. An approach to evaluating and comparing biomarkers for patient treatment selection. Int J Biostat 2014;10:99-121. [PubMed]
- Bouwmeester W, Zuithoff NP, Mallett S, et al. Reporting and methods in clinical prediction research: a systematic review. PLoS Med 2012;9:1-12. [PubMed]