Design and statistical principles of the SHIVA trial
Introduction
During the last two decades, most new agents have been designed to target molecular alterations involved in carcinogenesis. For instance, trastuzumab, a monoclonal antibody targeting HER2, has been approved for HER2-overexpressing breast cancer treatment in 1998 (1) and vemurafenib, a BRAF kinase inhibitor, has been approved in melanoma patients harboring the V600E BRAF mutation (2) to cite only two agents among numerous striking examples. Most of these agents are expected to produce anti-tumor activity only in the presence of the matching molecular alteration or companion biomarker. Even though the complete characterization of the target is an area of research for many agents such as mTOR inhibitors and antiangiogenic agents, the objective of treating patients based on the molecular profile of their tumor is claimed by most of the sponsors and investigators. Nevertheless, molecularly targeted agents (MTAs) have been assessed to date according to tumor location and histology. Investigations in other tumor types are then pursued on a case by case basis. For instance, trastuzumab eventually also demonstrated anti-tumor activity in advanced/metastatic stomach cancers overexpressing HER2 (3). However, this approach is rapidly limited by the sample sizes required for clinical trials: the combination of the (low) prevalence of some alterations as well as some specific tumor types transforms several subgroups into rare diseases. The sequential development of a MTA in multiple tumor types with the same molecular abnormality in most cases is thus unrealistic.
Recent advances in high-throughput technologies allow for the screening of a large panel of molecular alterations in a reasonable timeframe for clinical practice, which opens the possibility to select and personalize treatment based on the molecular profile of the tumors. The National Cancer Institute (USA) has recently defined personalized medicine as “a form of medicine that uses information about a person’s genes, proteins, and environment to prevent, diagnose, and treat disease” (4). The question of whether personalized medicine based on the molecular profiling of the tumor of cancer patients improves their outcome has arisen. In a prospective cohort study, von Hoff and colleagues investigated the benefit of selecting treatment for refractory cancer based solely on the tumor biology (5). They found that 27% of the patients had a progression-free survival (PFS) increased by 30% as compared to the time to progression (TTP) obtained with the previous line of treatment, assessed retrospectively. In a comparative non randomized trial, Tsimberidou and colleagues reported that in patients with at least one druggable molecular alteration identified in their tumor, matched MTA compared with treatment without matching was associated with a higher objective response rate, longer PFS, and longer survival (6). However, the lack of randomization vs. standard of care in these studies did not allow for drawing robust conclusions (7).
Genesis of the SHIVA trial
The SHIVA trial was designed within the Institut Curie to evaluate whether tumor biology is a more important driver for treating cancer patients than tumor location and histology. For ethical reasons, only patients with cancers refractory to approved treatments for their disease were selected, similar to the two above mentioned studies (8). Furthermore, the concept appeared particularly attractive for less common or rare tumor types for which dedicated randomized trials of MTAs are usually not carried out. This supported the idea to include all solid tumor types that can be evaluated for efficacy using the same criteria. A very large set of MTAs is under development, with various levels of evidence of activity depending on the stage of development. We decided to use only approved drugs in order to control for this source of heterogeneity. Use of combinations that have often strong biological rationale was limited by safety issues as few phase I trials combining two approved MTAs have been published.
We initiated the SHIVA trial (NCT01771458), a randomized proof-of-concept phase II trial comparing molecularly targeted therapy approved at the time of the trial (outside of their approved indications) based on metastasis molecular profiling vs. conventional chemotherapy (or best supportive care) in patients with any kind of cancer refractory to standard of care. The intervention evaluated in this trial can be described as a complex algorithm that determines the association of a treatment with a putative adequate target.
We introduce here the rationale for the design, the choice of endpoints, the type of conclusions we can expect and specificities due to this type of clinical question. We emphasize the necessity of randomized trials, and explore the power of the trial in case only part of the algorithm would be efficient, that is if only some MTAs actually work in the presence of the selected target while others do not.
Design of the SHIVA trial
The primary objective of the SHIVA trial is to compare the efficacy in terms of PFS of molecularly targeted therapy based on molecular profiling versus conventional therapy in patients with solid tumors refractory to standard treatments. PFS is defined as the delay between randomization and progression according to RECIST 1.1 (9) or death, whatever the cause. Secondary efficacy objectives are to investigate the tumor growth according to the treatment arm, to explore the possible variation in treatment effect according to the altered pathway (interaction test), and to compare the tumor growth obtained with the MTA and the standard treatments for patients who cross over. Tumor growth is defined quantitatively as the sum of the size of the targeted lesions identified using RECIST 1.1 standardized by the delay between measurements. In this secondary analysis, patients with clinical progression and no evidence of radiological progression and patients with new lesions will be analyzed based on the radiological measurements only. Additional analyses including clinical progression and the occurrence of new lesions will be included in a sensitivity investigation.
The flowchart of the study is provided in Figure 1. The SHIVA trial includes an observation cohort study as well as a randomized trial. In brief, the molecular profile of a patient tumor is performed on a mandatory biopsy/resection of a metastasis and analyzed by a molecular biology board made of biologists, physicians and bioinformaticians. If no molecular alteration for which an approved matched MTA exists in the frame of the SHIVA trial was identified, the patient is not eligible for the randomization and is entered into a prospective observational cohort. If one or several molecular alterations are identified, the molecular biology board applies a pre-defined algorithm to select the best MTA (see Table 1). Patients are then randomized between receiving the selected MTA or receiving a conventional treatment according to the investigators’ choice (that is based on tumor type, histopathological characteristics etc.). The investigator and the patient are blinded to the molecular profile. More details can be found in the paper by Le Tourneau and colleagues (10). The protocol for the research project has been approved by an Ethics Committee and the trial conforms to the provisions of the Declaration of Helsinki. The remainder of this communication focuses on the randomized trial of the SHIVA program.
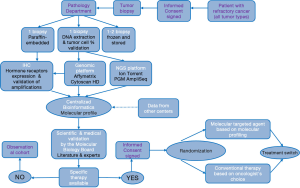
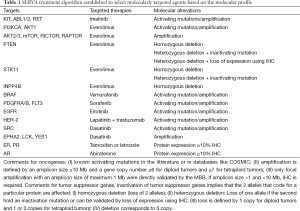
Full table
It is well known that prognosis differs depending on the tumor type, although patients with the same cancer in terms of location and histology might also display different prognosis (11). In order to control for patient heterogeneity from differences in prognosis, randomization is stratified according to the signaling pathway relevant for the choice of the MTA and the patient prognosis based on the two categories of the Royal Marsden Hospital (RMH) score for oncology phase I trials (12). Although molecular alterations may be prognostic for PFS, it was not possible to stratify the design for all possible molecular alterations. Three main signaling pathways have been arbitrarily identified: (I) the hormone receptors pathway; (II) the PI3K/AKT/mTOR pathway; and (III) the MAP kinase pathway (see Table 1). Therefore, combining the two levels of prognosis from the RMH score with the three molecular pathways, the randomization and the planned primary analysis are stratified on six strata.
A cross-over is allowed at disease progression for patients in both treatment arms (patients who received conventional chemotherapy were proposed the MTA and vice-versa). Last, quotas were introduced so that no more than 20% of the randomized patients had the same tumor type.
A feasibility evaluation was planned after the first 100 patients to check the availability of the molecular profile within four weeks after the biopsy, to review the different steps to draw the molecular profile and the algorithm to guide treatment’s selection (10). No modification of the process and the algorithm was required after this interim feasibility analysis.
The population of interest included various tumor types and various number of previous lines of treatment, similar to the population enrolled in phase I trials. The expected PFS of this population in the control arm could be derived from the one reported in phase I clinical trials of cytotoxic agents that have been eventually approved: 6-month PFS in this patient population was around 15% (13). Under the hypothesis that doubling the 6-month PFS rate from 15% to 30% was clinically relevant (i.e., HR =0.63), a total of 142 events is required to detect a statistically significant difference in PFS between the randomized arms with a type I error of 5% and a power of 80% in a bilateral setting. To observe these events after an accrual time of 18 months and a minimum individual follow-up of six months, about 200 patients would need to be randomized onto this trial.
Design specificities relating to the use of high throughput technologies
The evaluation of a complex intervention such as the SHIVA algorithm to select the MTA raises specific issues that not only impact the design, but also the statistical analysis and the final interpretation. First, this complex intervention combines two aspects: the treatment effect and the choice of the putative matching target. Therefore, the resulting efficacy can be related to either of the two and the final interpretation is the evaluation of the whole strategy compared to another strategy (physician choice) which uses different treatments and a different modality to select the treatment. Second, several sources of variability related to the complexity of the intervention may contribute to the final results of the experiment. Eleven different targeted treatments have been administered based on 22 targets characterized by several dozen molecular alterations (see Table 1). A fundamental assumption behind the design is that the intervention has similar effects (or absence of effects) in all six strata, whatever the allocated treatment and whatever the molecular alteration used to select the treatment. This is the homogeneity assumption. In case the algorithm is only partly efficient, the power of the study is impacted. The magnitude of the impact is investigated in the following section. Third, as in any scientific experiment, the algorithm to select patients must be duly described, reproducible and applicable to all participants. Defining the treatment algorithm was challenging as the knowledge regarding the biology of the tumors and the high-throughput platforms evolve quickly with time and initial biological assumptions might become outdated.
Strengths of the selected design
Randomization
A randomized clinical trial is mandatory to evaluate the added value of omic-based classifiers to guide patient’s treatment compared to standard approaches (14,15). Although the tumor biology, the mechanisms of drug resistance, and the role of the tumor environment are known to be crucial to accurately predict patient outcomes, they remain largely unknown, making it necessary to have a comparator. Furthermore, the prognosis of the highly selected patients (those whose tumors have a set of pre-defined molecular alterations) enrolled in such trials is not well-known, and only randomized experiments can disentangle the benefit of the intervention from the benefit obtained with supportive care or conventional chemotherapy outside of standard of care. Randomization is the only way to control for known and unknown confounding factors and to evaluate the causality in such a complex intervention. The more complex the intervention, the more numerous the unknown confounding factors. Likewise, only an intent-to-treat analysis that makes full use of the randomization is appropriate. However, as shown in the next section, this is necessary but this may not be sufficient to provide a clear picture of the benefit of the complex intervention.
Blinded design
Blinding to the molecular profile is a crucial component to evaluate the benefit of the intervention (16). The expectations of the physicians and of the patients in omic-based algorithms to select MTAs are high, and there is a risk of bias in the interpretation of treatment efficacy that would favor the intervention arm. Ideally a double blind trial should be designed; however this was impossible in the SHIVA trial due to the numerous treatments administered to the patients in both arms with various formulations (oral or intravenous).
Algorithm reproducibility
The treatment algorithm to select the best MTA based on a molecular profile was defined by the biologists and the physicians. It includes molecular alterations (in particular oncogene activations and gene suppressor inactivation) that had been demonstrated to have a predictive value of the effect of some treatments in the clinic, such as HER2 amplification and BRAF mutations. Others were based on a strong biological rationale that had not been validated in the clinic, such as PIK3CA mutations. The complete treatment algorithm was defined and secured before initiating the trial. Based on the knowledge of targetable signaling pathways, it makes explicit the definition of what should be considered a druggable molecular alteration (activating and inactivating mutations, focal amplifications, heterozygous and homozygous deletions, etc.), the thresholds for quantitatively measured molecular alterations (fold change and maximal size of focal DNA amplification for instance), validation of some protein expression measures using IHC, the priorization between molecular alterations when several of them were relevant and the correspondence between molecular alterations and MTAs. Each of these aspects is a potential source of variability. Extensive theoretical work has been performed in the SHIVA trial to enable strong control of the underlying heterogeneity, in line with the recommendations of McShane and colleagues (17). Amplifications, gene losses and deletions were clearly defined as a function of copy number alterations corrected to the tumor cell content and the size of the amplification. Similarly, for mutations analyses, thresholds for variant calling were set according to the frequency, strand ratio and reads’ coverage (10). The molecular alterations included in algorithm are precisely documented in terms of techniques used to assess these alterations (18). Furthermore, the molecular technologies are evolving rapidly and in the SHIVA trial two different sequencing panels (Ion Ampliseq Panel version 1 and version 2) were used. Therefore, before updating the sequencing protocol several samples were analyzed in parallel with both panels to ensure the reproducibility and the homogeneity of the results. In the same way, all bioinformatics analyses defined during the feasibility part of the project were centralized and applied to all patients regardless of recruitment center. No modification of the bioinformatics workflows were accepted after the feasibility part of the project. Finally, all patients enrolled in the trial are analyzed in the same way. This is crucial as any research must be self-explanatory and reproducible. A treatment algorithm that relies only on understated experts’ opinion would not be applicable outside of the center and conclusions would not be applicable and generalizable to other samples.
Cross over
Cross-over is allowed in the SHIVA trial to patients at disease progression. Patients initially randomized in the intervention group may then receive conventional chemotherapy based on their tumor type, and patients in the control arm may receive the MTA matching the molecular alteration identified on the biopsy performed at inclusion, provided all eligibility criteria are still fulfilled at the time of progression. The analysis plan included a comparative analysis of the TTP after each of the two treatments using the patient as his (her) own control for the subset of patients who could receive second treatment. The randomization between the two arms of treatment can also be seen as a randomization between the two sequences of treatment, fulfilling one requirement of cross-over designs. The statistical power of this analysis might theoretically be higher than the one comparing the treatment efficacy between the two groups as it enables control for the various sources of patients-related heterogeneity such as the natural history of the disease (the tumor location and histology), the history of previous treatments etc. if TTP for the two lines of treatment are correlated (14). Furthermore, in this planned cross-over, all tumor evaluations are performed using the same criteria, the same set of target lesions identified prospectively. This gives a better and more robust assessment of the two consecutive TTP compared to retrospective assessment. However, cross-over was not mandatory and in the likely case that a large fraction of patients cannot receive both arms (i.e., no crossover) due to clinical deterioration for instance, the power would be lower and the conclusions may be biased. Accordingly, the primary analysis relied on the first period only.
Tumor diversity
Quotas for tumor types were set up in the protocol to avoid over-representation of more frequent tumor types such as breast, lung or colorectal cancers. No more than 20% of the randomized patients are allowed to be enrolled for a given tumor type. A wide diversity of tumors has been enrolled. Differences between the treatment arms would then be unlikely related to a given tumor type. This would reinforce the interest of developing new treatments based on biology first, possibly across multiple diseases.
Biopsy of a metastatic site
In the SHIVA study all patients must undergo a biopsy of a metastatic site before being treated, so that we are sure that the molecular profile established reflects what will be treated as controversial results have been reported on the agreement between molecular profiles measured on the metastasis and on the primary (19,20). However, patients were allowed to receive chemotherapy (but no MTA or hormone therapy) between the time of biopsy and randomization. Establishing a molecular profile on the primary tumor may not accurately reflect the molecular profile of the tumor at the time of treatment, especially if patients have been previously treated with MTAs that can act as a selection pressure in some malignancies, driving clonal evolution and selecting for certain resistant subclones or developed de novo on treatment (21).
In summary, the randomized design for the SHIVA trial allows for comparing two complex strategies on a valid endpoint, while controlling for numerous confounding factors. A statistically significant difference between the two arms would be appropriately interpreted as the superiority of treating patients with MTAs based on molecular alterations and a pre-defined treatment algorithm compared to the conventional approach based on tumor location and histology. In other words, do we perform better than what we usually do for these patients?
Limitations of the selected design
Interpretation
An important question that will not be addressed in the SHIVA trial is the independent effect of the treatment algorithm. The design will not enable the disentanglement of the treatment effect from the algorithm effect. If a given MTA is active irrespective of the measure of the target (that is of the algorithm), we would draw the same conclusions as if the treatment worked thanks to the adequate selection of the patients. The US NCI sponsored M-PACT trial (NCT01827384) presented in the same issue of the journal has been designed to specifically address the question of the added value of the algorithm. Conversely, the control arm used in the M-PACT trial does not correspond to any standard of care and the trial will not be able to conclude whether the global strategy is superior to the usual practice. Both trials are therefore quite complementary.
Population heterogeneity
If randomization guarantees that the two groups of patients have comparable characteristics and the same overall prognosis, heterogeneity may dilute the expected benefit. Heterogeneity impacts any clinical research, but several sources of potential heterogeneity across patients are specific for (or more likely with) this kind of trial: the location and histology of the tumor, the molecular alterations, the assays used to identify the molecular alterations, and the diversity of treatments under study. Stratification of the randomization and of the analysis on the RMH prognostic score and on the signaling pathway is an efficient mean to control part of this heterogeneity, assuming no interaction between the strata and the treatment effect. It was impossible to stratify on the numerous tumor types. On the contrary, as noted in the previous section we tried to increase the diversity of the tumor types to be able to draw conclusions that would be broadly applicable. This source of heterogeneity is intrinsic to the question addressed by the SHIVA trial and we tried to build on it, while controlling for the other identified sources.
Homogeneity and power
Beyond the expected heterogeneity in the population’ prognosis, there is a risk of heterogeneity in the effect of the MTA selected based on the molecular alteration. Statistically, this would mean an interaction between the MTA effect and patient’s characteristics. In other words, the algorithm to select the right treatment would be efficient for some molecular alterations (or equivalently for some treatments) and not for others. For instance, suppose that the treatment selected in case of an alteration on the PI3K/AKT/mTOR pathway is not active in this subset of patients, this would reduce the power of the primary analysis. Our ability to detect a 50%-reduction in the rate of progression or death at six months would be lower than the planned 80%. This is illustrated by the forest plots in Figure 2. Each line represents the MTA effect in a different stratum. In panel A, we have homogeneity of the treatment effect across all strata: whatever the signaling pathway and the prognostic group, the PFS rate is increased by 50%. Conversely, in panel B, no treatment effect is observed in one of the strata and the overall power of the primary analysis is reduced from 80% to 66%. The magnitude of the power loss depends on the number of strata where the MTA is not active, as shown in Table 2. The size of each stratum is also directly related to the power (results not shown). Homogeneity tests (or interaction tests) are part of the statistical analysis plan in order to detect this pattern of results. However, interaction tests are notoriously underpowered as shown in Table 2 and a strong heterogeneity may remain statistically undetected at the 5% level.
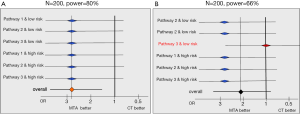
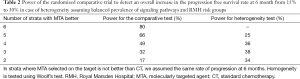
Full table
Endpoints
The primary endpoint for SHIVA is PFS, which is used in many clinical trials to evaluate treatment benefit in advanced disease. As secondary endpoint, the quantitative measure of the tumor growth is analyzed (22). This endpoint has been increasingly investigated in recent years due to the potential increased information carried in continuous outcomes (23). In particular, an improved ability to detect interactions between the treatment effect and baseline characteristics such as the signaling pathway is expected. However, recent works have demonstrated that none of the endpoints based on the tumor growth proposed to date were a good surrogate of the patient’s survival (24), and this is not clear whether a treatment effect measured on the tumor growth would be strongly predictive of a treatment effect on the PFS; furthermore, the best way to combine information from tumor growth and the occurrence of new lesions or clinical symptoms is still an area of research. In the SHIVA trial, this endpoint may help to provide a better understanding of the data, but it could not be used as a primary endpoint instead of PFS.
Perspectives
More than 900 MTAs are under development (25). However the large majority (95%) are tyrosine kinase inhibitors, 4% target the cell cycle, while less than 1% target alternative pathways, which limits the range of eligible targets. The prevalence of molecular alterations varies strongly according to the tumor type (26), also by the stage of the tumor (27), and the exposure to previous MTAs. Many subgroups represent less than 15% of the cancer patients with a tumor type. The SHIVA randomized trial has been set up to investigate which of tumor biology or tumor location and histology is the most important to select treatment in patients with cancer refractory to the standard of care. Interpretation of the results of such trials are complicated by the complexity of the algorithm, but only randomized trials can disentangle the consequence of prognostic factors in these highly selected patients from the intervention effect and enable to control for confounding factors to allow reliable conclusions (28).
The statistical principles for the SHIVA trial integrate various aspects to reduce the variability related to the potential heterogeneity of the population. This heterogeneity will be balanced between the two treatment arms and thus should not induce spurious association, but it may dilute the effect of the intervention. Standardization of the process to identify druggable molecular alterations and the matching MTA, as well as the blinding of the results are key elements in such trials. The same principles as those applied for the development of diagnostic tools should be implemented (29).
There is clearly a need for more sensible endpoints to evaluate such complex interventions. PFS is mildly sensitive to treatment variations and interaction tests to identify differential effects according to the matching between treatment and target are not powerful with 200 patients. Pharmacodynamic endpoints such as functional imaging or biomarkers are promising to detect early treatment failure but have none yet validated.
Overall, cancer biology is at the heart of this type of histology-agnostic trial. Current knowledge of tumor biology does not enable us to systematically predict the final outcome as shown by the disappointing efficacy obtained with vemurafenib in BRAF mutated colon cancer (30), or those obtained with crizotinib in neuroblastoma with ALK-translocation (31). Taking into account the presence or the absence of several molecular alterations might improve the accuracy of the treatment algorithms using systems biology approaches. However, any treatment algorithm should be clearly defined and rigorously evaluated in randomized trials. In addition, the tumor environment is likely an important factor of success of a therapeutic approach, as illustrated with the recent approval of immunotherapeutics. Nevertheless, the question of what is the strongest predictor of the treatment effect and whether matched MTA to molecular profile compared to conventional chemotherapy is more effective for cancer patients is crucial for the scientific community as well as for the patients. A total of 741 patients have been enrolled in 18 months and 197 have been randomized to date. Final efficacy results of the SHIVA trial are expected in 2015.
Acknowledgements
The SHIVA team is indebted to all patients who participated in this trial. More than 100 persons have been working on this trial; their essential contribution is duly acknowledged. The SHIVA trial is supported by the grant ANR-10-EQPX-03 from the Agence Nationale de le Recherche (Investissements d’avenir) and SiRIC (Site de Recherche Intégré contre le Cancer).
Footnote
Conflicts of Interest: The authors have no conflicts of interest to declare.
References
- Pegram MD, Lipton A, Hayes DF, et al. Phase II study of receptor-enhanced chemosensitivity using recombinant humanized anti-p185HER2/neu monoclonal antibody plus cisplatin in patients with HER2/neu-overexpressing metastatic breast cancer refractory to chemotherapy treatment. J Clin Oncol 1998;16:2659-71. [PubMed]
- Chapman PB, Hauschild A, Robert C, et al. Improved survival with vemurafenib in melanoma with BRAF V600E mutation. N Engl J Med 2011;364:2507-16. [PubMed]
- Bang YJ, Van Cutsem E, Feyereislova A, et al. Trastuzumab in combination with chemotherapy versus chemotherapy alone for treatment of HER2-positive advanced gastric or gastro-oesophageal junction cancer (ToGA): a phase 3, open-label, randomised controlled trial. Lancet 2010;376:687-97. [PubMed]
- NCI Dictionary of Cancer Terms. Available online: http://www.cancer.gov/dictionary?CdrID=561717. Last Access April 3, 2015.
- Von Hoff DD, Stephenson JJ Jr, Rosen P, et al. Pilot study using molecular profiling of patients' tumors to find potential targets and select treatments for their refractory cancers. J Clin Oncol 2010;28:4877-83. [PubMed]
- Tsimberidou AM, Wen S, Hong DS, et al. Personalized medicine for patients with advanced cancer in the phase I program at MD Anderson: validation and landmark analyses. Clin Cancer Res 2014;20:4827-36. [PubMed]
- Doroshow JH. Selecting systemic cancer therapy one patient at a time: is there a role for molecular profiling of individual patients with advanced solid tumors? J Clin Oncol 2010;28:4869-71. [PubMed]
- Le Tourneau C, Kamal M, Trédan O, et al. Designs and challenges for personalized medicine studies in oncology: focus on the SHIVA trial. Target Oncol 2012;7:253-65. [PubMed]
- Eisenhauer EA, Therasse P, Bogaerts J, et al. New response evaluation criteria in solid tumours: revised RECIST guideline (version 1.1). Eur J Cancer 2009;45:228-47. [PubMed]
- Le Tourneau C, Paoletti X, Servant N, et al. Randomised proof-of-concept phase II trial comparing targeted therapy based on tumour molecular profiling vs conventional therapy in patients with refractory cancer: results of the feasibility part of the SHIVA trial. Br J Cancer 2014;111:17-24. [PubMed]
- Le Tourneau C, Paoletti X, Coquan E, et al. Critical evaluation of disease stabilization as a measure of activity of systemic therapy: lessons from trials with arms in which patients do not receive active treatment. J Clin Oncol 2014;32:260-3. [PubMed]
- Arkenau HT, Barriuso J, Olmos D, et al. Prospective validation of a prognostic score to improve patient selection for oncology phase I trials. J Clin Oncol 2009;27:2692-6. [PubMed]
- Horstmann E, McCabe MS, Grochow L, et al. Risks and benefits of phase 1 oncology trials, 1991 through 2002. N Engl J Med 2005;352:895-904. [PubMed]
- Buyse M, Quinaux E, Hendlisz A, et al. Progression-free survival ratio as end point for phase II trials in advanced solid tumors. J Clin Oncol 2011;29:e451-2; author reply e453.
- Mandrekar SJ, Sargent DJ. Drug designs fulfilling the requirements of clinical trials aiming at personalizing medicine. Chin Clin Oncol 2014;3:14. [PubMed]
- Boutron I, Estellat C, Guittet L, et al. Methods of blinding in reports of randomized controlled trials assessing pharmacologic treatments: a systematic review. PLoS Med 2006;3:e425. [PubMed]
- McShane LM, Cavenagh MM, Lively TG, et al. Criteria for the use of omics-based predictors in clinical trials. Nature 2013;502:317-20. [PubMed]
- Servant N, Roméjon J, Gestraud P, et al. Bioinformatics for precision medicine in oncology: principles and application to the SHIVA clinical trial. Front Genet 2014;5:152. [PubMed]
- Vakiani E, Janakiraman M, Shen R, et al. Comparative genomic analysis of primary versus metastatic colorectal carcinomas. J Clin Oncol 2012;30:2956-62. [PubMed]
- Vignot S, Frampton GM, Soria JC, et al. Next-generation sequencing reveals high concordance of recurrent somatic alterations between primary tumor and metastases from patients with non-small-cell lung cancer. J Clin Oncol 2013;31:2167-72. [PubMed]
- Hiley C, de Bruin EC, McGranahan N, et al. Deciphering intratumor heterogeneity and temporal acquisition of driver events to refine precision medicine. Genome Biol 2014;15:453. [PubMed]
- Le Tourneau C, Servois V, Diéras V, et al. Tumour growth kinetics assessment: added value to RECIST in cancer patients treated with molecularly targeted agents. Br J Cancer 2012;106:854-7. [PubMed]
- Litière S, de Vries EG, Seymour L, et al. The components of progression as explanatory variables for overall survival in the Response Evaluation Criteria in Solid Tumours 1.1 database. Eur J Cancer 2014;50:1847-53. [PubMed]
- An MW, Mandrekar SJ, Sargent DJ. Application of tumor measurement-based metrics in the real world. J Clin Oncol 2013;31:4374. [PubMed]
- PhRMA. Medicines in Development for Cancer. 2012. Available online: http://www.phrma.org/sites/default/files/pdf/phrmamedicinesindevelopmentcancer2012.pdf. Last Access in April 3, 2015.
- Kandoth C, McLellan MD, Vandin F, et al. Mutational landscape and significance across 12 major cancer types. Nature 2013;502:333-9. [PubMed]
- Neuzillet Y, Paoletti X, Ouerhani S, et al. A meta-analysis of the relationship between FGFR3 and TP53 mutations in bladder cancer. PLoS One 2012;7:e48993. [PubMed]
- Buyse M, Michiels S. Omics-based clinical trial designs. Curr Opin Oncol 2013;25:289-95. [PubMed]
- Rennie D. Improving reports of studies of diagnostic tests: the STARD initiative. JAMA 2003;289:89-90. [PubMed]
- Prahallad A, Sun C, Huang S, et al. Unresponsiveness of colon cancer to BRAF(V600E) inhibition through feedback activation of EGFR. Nature 2012;483:100-3. [PubMed]
- Mossé YP, Lim MS, Voss SD, et al. Safety and activity of crizotinib for paediatric patients with refractory solid tumours or anaplastic large-cell lymphoma: a Children’s Oncology Group phase 1 consortium study. Lancet Oncol 2013;14:472-80. [PubMed]