Molecular and clinical implementations of ovarian cancer mouse avatar models
Introduction
Ovarian cancer (OC) accounts for the most lethal gynecological malignancy, ranking fifth in cancer deaths amongst women. On an annual rate, nearly 220,000 women are diagnosed with epithelial ovarian cancer (EOC) worldwide. The American Cancer Society estimates about 21,980 women will be diagnosed with OC in 2014 in the United States, 14,270 of which are expected to die (1). With its late presentation, most cases suffer wide metastasis within the abdomen by time of presentation, explaining the high death rate (2).
Chemo-resistance and long-term survival for patients with advanced disease are still a major challenge, with only 5% of the anti-cancer agents gaining FDA approval after pre-clinical testing (3). Such impedance in cancer-related therapeutic advances causes a major economic burden. By the year 2020, the direct/indirect cost of cancer is estimated to be $300 billion dollars in the US alone. With these burdens in mind, several in vitro and in vivo strategies are being developed in an attempt to enhance our ability to combat cancer.
One of the major barriers to improvement in this disease is the heterogeneous nature of OC, which encompasses a collection of varying histologic types including serous papillary, endometrial, clear cell, mucinous and other rare subtypes, all with characteristic differences. Even amongst the most common subtype, serous, there is differential response to therapy and predictive markers of response to chemotherapeutic agents are lacking. An additional barrier to improving outcomes in OC is the lack of models that are predictive in response to chemotherapy.
For over 4 decades, patients with high-grade EOC have been all treated with a platinum-based treatment regimen irrespective of histologic subtype, known mutational status (e.g., BRCA1 or BRCA2) or biomarkers of response that could better inform treatment (4). Lack of pre-clinical models that can accommodate the heterogeneity of EOC and represent the donor microenvironment is a major reason of the failure of novel therapies to influence clinical outcomes for patients with OC (4).
Cell lines-derived xenograft models
Classic cell line-derived xenografts have facilitated the development of more insightful approaches towards key signaling pathways of OC oncogenesis and chemoresistance. In a lab-based environment, these cancer cells are propagated in vitro or in vivo and allowed to grow on artificial support. However, mere growth in such an environment does not simulate the natural pathophysiology associated with cell growth in human homeostasis. For example, cells’ genetic information, growth patterns, invasion behaviors, and accompanying cellular population all acquire various alterations in order to adapt to such new environment (5). However, it is to be noted that genetic alterations in tumor cells caused by maintaining cancer cell lines are completely distinctive from genetic alterations caused by stressful mutagenesis from cancer itself.
It has also been demonstrated by The Cancer Genome Atlas (TCGA) ovarian dataset that phenotypic and genotypic discrepancy occurs between OC and cancer cell lines. Such lines also lack the stromal tissue, which represents not only the main bulk of EOC, but also the platform for insulin-like growth factor (IGF) and cMET/hepatocyte growth factor (HGF). Cell lines and xenografts originating from OC cells do not accurately recapitulate the patients’ tumor microenvironment. As such, while existing models are useful in mechanistic work at the protein and pathway level, they fall short of being able to translate novel therapies or understand determinants of sensitivity to current or new therapies for the treatment of OC.
OC patient-derived xenografts (PDX) models
Because of the limitations associated with cell line derived xenografts, interest has developed towards PDX as well as genetically engineered mouse models (GEMMs). These in vivo types of models can better represent the heterogeneous nature of human tumors, which have made them the preferred replicas for drug development and screening research. Many authors have described materials and methods essential to generate OC PDX models (6-8). The idea is to collect fresh primary or secondary tumor samples from patients either during diagnostic biopsy or debulking surgery. It has also been reported that fluid collected from malignant ascites is a valid source for tumor samples. Then, these tumor samples are minced and tissues are transplanted into immunocompromised mice either orthotopically or non-orthotopically.
Orthotopic OC PDX models
Orthotopic OC mouse model accounts for transplantation of tumor cells either into ovarian bursa (intrabursally, IB) (8), or into mouse peritoneal space (intraperitoneally, IP). Consequently, generating the Avatar, an orthotopic PDX with successful engraftment of parenchymal and stromal tumor components, can facilitate further study of cellular interactions, namely between tumor cells, lymphocytes, and fibroblasts (6-8).
One of the rate limiting factors controlling immune response towards cancer cells is presence of tumor stromal components (9,10), specifically T-lymphocytes (11). It has also been shown that fibroblasts can promote growth of invasive cancer breast tissue in PDX models (12). Tumor-associated fibroblasts have been shown by others to enhance or suppress T cell function (13,14) and subsets of tumor-associated T cells are known to interact with tumor cells and reciprocally modulate each other (15). It is not evident yet whether there is any augmenting or suppressing effect of fibroblasts and leukocytes over tumor cells growing in human environment (16,17).
Non orthotopic OC PDX models
Different microenvironment surrounding non-orthotopic tumor grafts can lead to different transplantation outcome or phenotype. Examples of non-orthotopic locations for OC include mammary fat pad, subrenal capsule, and subcutaneous (SC) space.
Although xenografts generated by SC transplantation of tumor cells into NSG mice yielded typical tumor cellular architecture, leukocytic traffic, and fibroblasts surviving for extended durations; they are only capable of spreading locally and limitedly. Such altered growth patterns do not represent growth and metastatic behavior observed in cancer patients, which thereby hinders their applicability for discovering potential growth factors and testing immunomodulatory agents (18).
On the other hand, subrenal capsule transplantation of tumor cells in Severe Combined Immunodeficiency (SCID) mice has demonstrated >95% engraftment rate, preserving histopathologic and immunohistochemical characters of the original parent tumor. This PDX enabled further investigation in many OC subtypes, including benign, borderline, malignant surface, and granulosa cell tumors. High preservation of immune phenotypes in xenografts was reported in these models with significant yet small increase in MIB-1 proliferation index (19). Subrenal engraftment was also studied by Press et al. who generated serially transplanted xenografts of 4 primary tumors in order to evaluate their genotypic and phenotypic stability. Although these grafts did not respond to a poly (ADP-ribose) polymerase-1 inhibitor; they showed favorable response to carboplatin/paclitaxel in the form of tumor volume and proliferation reduction. They also showed concordant proliferation between primary tumor and xenograft using MIB-1 (20).
Paramount hallmarks of OC Avatar
Histological and molecular correlation between patient’s tumor and their Avatars
Histopathologic and molecular similarities were observed in grafted tumors and original patient cancers. Among 168 models generated by Weroha and colleagues, high statistically significant microscopic fidelity between tumors and respective grafts was observed, with correlation of 0.74, taking into consideration comparing stromal mimicry as well. However, when patient and tumor graft tissues were evaluated for expression of human vimentin using non-mouse matching antibody, patient stroma stained strongly while tumor graft stroma did not, indicating that the stroma is murine. They also discovered comparable genomic aberrations in low passage grafts matching with corresponding primary tumors obtained from primary surgical specimen. Nevertheless, tumor-associated lymphocytes (TAL) especially CD45-positive cells did not frequently coheterotransplant with epithelial tumors. Array CGH was performed on two tumor graft models from different patient tumors. The results show marked overlap in genetic gains and losses between the patient tumor and corresponding tumor graft (6). In agreement with this, Topp et al. generated both intrabursal (IB) and SC routes of transplantation in High Grade Serous Ovarian Cancer (HG-SOC). They reported that histologic appearance of H + E stained sections of SC versus IB (orthotopic) PDXs was similar, with serous papillary structures present in PDXs from both sites (8).
Correlation between patients and their Avatars response to platinum therapy
Anticipation of sensitivity and recognizing elective treatments early have a major clinical impact on patient prognosis. Two recent reports have displayed that HGSC Avatar exhibit a similar correlative response to platinum when matched to patient response: Topp and associates described platinum response in SC HGSC Avatar, with three of four platinum sensitive HG-SOC Avatar containing DNA repair gene mutations, and the fourth being methylated for BRCA1, though conversely, each of the three platinum refractory models overexpressed dominant oncogenes (CCNE1, LIN28B and/or BCL2) (8). Similarly, Weroha and colleagues utilized nine HGSC intra-peritoneal Avatar models, treated with four rounds of carboplatin/paclitaxel. Nine out of nine tumorgrafts showed in vivo platinum response complying with patient’s clinical response (6). Patient Avatar models can be important instruments in foreseeing which patients may benefit from the utilization of platinum-based therapies, and highlighting those for whom platinum may have limited value, who would require different treatments in the short-term.
Implementations of Avatar in OC research
Studying mechanisms of development, progression, invasion and metastasis of EOC
There is an expanding understanding that nonmalignant stromal cells including fibroblasts, epithelial cells and other leukocytes collaborate with and have an effect upon tumor development and metastases (21). This highlights the significance of models that preserve tumor microenvironment in studying penumbras related to tumor evolution.
EOC is characterized by exclusive pathogenesis that has been described elsewhere (22). One of the characteristics of shed EOC cells is their ability to coast freely and maintain their viability within peritoneal fluid. This is regulated in cancer cells by interaction of several adhesion molecules, for example, E-cadherin, CD44/hyaluronan, CA125/mesothelin, CXCR4/CXCL12, and b1 integrins (23,24). Such interactions are the main factor supporting their survival and further growth. This happens through activation of signaling molecules such as PI3K, and MAPK, which induce chemotherapeutic resistance and allow further metastatic potential of these cells (25).
All these factors consolidate the mirroring capability of Avatar models to the human physiologic environment, subject to the limitation that differences between species can complicate studying cellular interaction with peritoneal mesothelium. Likewise, it has been reported that genetic polymorphism in host mice impact the rate of metastasis in both human and mouse xenograft models (26). Until recently, molecular properties of tumor(s) were only investigated in patients by single views without real-time analysis, which has provided only a limited understanding to how these tumors spread or resist chemotherapy (27). “Avatars” have allowed us to transplant tumor tissue into multiple mice then harvest them at different time points; allowing a more complete understanding of these mechanisms (28).
Pre-clinical evaluation of chemotherapeutic strategies efficacy
Because of the low success rates facing chemotherapeutic agents (29), and the advancement of many of them towards costly phase III trials which wind up failing because of their limited efficacy (30), Avatar models may offer potential for evaluating novel therapies. With simple noninvasive investigation, Avatar tumors can be followed over time under treatment with various chemotherapeutic agents to analyze growth, vascularity, and metabolism, aided by available small animal imaging systems such as ultrasound, positron-emission tomography, computed tomography, and magnetic resonance imaging (6,31).
Outcomes to be measured during these studies can differ depending on the mechanism of anti-cancerous agent tested. Namely, if cytotoxic drugs are under trial, the end point of comparison should be percentage of tumor regression. If drugs against stem cells are discussed, an outcome of growth latency should be measured (32). Examples of further potential outcomes include tumor regrowth, regression, growth rate, and overall survival. Notably, the effect on growth rate has been described as more predictive of clinical response than tumor regression (33).
Understanding the mechanism of therapeutic response and resistance
Simulation of drug resistance can be demonstrated using Avatar models in OC. When tumors in a platinum-sensitive model receive prolonged exposure to cisplatin, development of resistance takes place (34). This can be useful to explore new medications that need to be tested in platinum-resistant strains, such as DNA minor groove binder lurbinectedin (35). It is also crucial to note that ovarian orthotopic xenografts, whether cisplatin sensitive or resistant, can reflect the same histopathologic regression under treatment encountered in human tumor responses (6,36).
Using Avatars to direct patient’s therapy
Cases of HG-SOC receive combination therapy of platinum and taxane as a first line of treatment. Despite the response most patients experience, a large proportion of patients relapse and develop platinum-resistant tumors (4). For these and similar scenarios, Avatar models for ovarian tumors could potentially be used to screen for chemotherapeutic resistance potential to tailor patients’ clinical management plan upon recurrence (Figure 1). This is in addition to valuable findings pertinent to drug-response and populations more likely to be sensitive to experimental therapies. While OC may serve as a model for such an approach, it is conceivable that engraftment, expansion and treatment experiments could be conducted in time for clinical need for other tumor types as well.
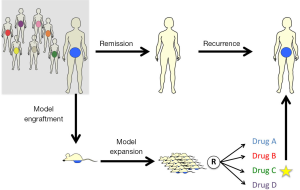
Avatar models can be valuable tools to guide patients’ therapy by testing one experimental agent (EXP) in order to evaluate drug response and resistance. They may also be used to compare response towards multiple chemotherapeutic agents. Thereby, the drug which provides the best outcome in the mouse model is considered the winning agent that should be prescribed to the patient, especially in cases of recurrent tumor.
Design principles for Avatar guided therapy
Clinical trial designs for initial validation of predictive biomarkers can be classified into enrichment, all-comers, adaptive, and the direct assignment option design categories (29,37-39). For a multi marker multi treatment setting, the likely trial design would be the marker strategy design. Figure 2 is a schematic representation of this design in the setting of a randomized, two-arm (marker-directed vs. empiric treatment assignment) design (40).
In the setting of Avatars, this is akin to randomizing patients to treatment based on their Avatar predictions versus treatment independent of Avatar predictions (based on physician choice, for example). While this design can theoretically address if the Avatar has predictive potential and has been historically referenced as the “gold standard”, it would require a large number of patients, and yet, still not be able to cleanly evaluate the predictive marker elements (41-43). Such a strategy can quickly become cost-prohibitive in the Avatar setting once the costs for the laboratory, technician, and treatments are included. The question of how then to “validate” the predictive or prognostic potential of the Avatars is an interesting exercise from a statistical design standpoint. We articulate some of the challenges and opportunities in this regard below.
The first step is to study the associations between Avatar-predictions and observed patient outcomes. For example, an Avatar-based design could be where the patient is offered the treatment choice based on physician choice, but at the same time, Avatars from that patient are exposed to all possible treatment options. Then data from this trial based on the observed patient outcome to the treatment received and the Avatar predicted outcomes (to all possible treatment choices) could be used to understand the predictive or prognostic potential of the Avatars (Figure 3).
At least two issues need to be addressed in the statistical analyses of data obtained from the Avatar design (Figure 3). Specifically, (I) are the associations between Avatar-predictions and observed patient outcomes driven by the tumor’s inherent sensitivity (sensitive or resistant to all treatments) or by the Avatar’s predictive ability (whereby a patient is sensitive to some treatments and resistant to others); and (II) are the Avatars prone to any misclassification errors with respect to patient’s sensitivity to a given treatment. For the latter, it is likely that there is in fact misclassification from the physiological differences between the Avatar and the patient, or due to other factors such as the choice of endpoint (assessment of response in the Avatar as compared to a patient), time point of assessment of endpoint (day 28 in mice versus 3 or 6 months in patient). The proposed design strategy (Figure 3) also lacks the ability to assess each treatment individually. Instead, it serves to inform the prognostic/predictive promise of the Avatar as a marker, and thus is most useful as a “treatment strategy” in an initial validation setting. This design also rests on the assumption that methods for Avatar assessment (if we consider the Avatar to be similar to an assay) are well established, and that the Avatars have good clinical and statistical properties in terms of reproducibility and validity. To establish the latter, the optimal number of animals per Avatar model, as well as the appropriate endpoint and timing of endpoint assessment in the Avatar experiments must have been well-studied.
Avatar-directed chemotherapy offers the potential to improve the poor outcomes from salvage chemotherapy by assigning patients to the most effective regimen in their own, personal Avatar. Figure 4 provides a schematic of the hypotheses regarding the prognostic and predictive properties of the Avatar, for two treatments. Clearly Avatars can be used to guide choice of “multiple” therapies, but for ease of representation, we considered two treatment options.
Looking forward, if the Avatars can truly be considered a “replica” of the patient, then the Avatars can first be exposed to the experimental treatment, and then based on the Avatar responses that can be used as a marker to predict benefit from the EXP, a marker stratified design (stratified by Avatar responses to the experimental treatment) can be utilized to randomize patients to the EXP or standard of care (SOC) (Figure 5).
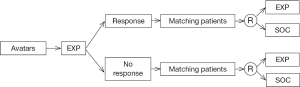
Challenges and limitations of PDX
While PDX models provide opportunities for improvement in cancer research in testing diseases’ pathomechanisms and treatment, there are important limitations that come with this tool that need more analysis and investigation in order to validate the potential translational uses of these models.
Time and cost
Developing PDX models requires expensive maintenance of tumor banks, animal facilities, laboratory consumables, and experienced personnel to ensure the immediate availability of mice on demand and histopathological cores incurring high cost (30). Moreover, the process of harvesting, transplanting, propagating, then drug testing of one mouse model consumes extended periods of time, which may extend beyond the life expectancy of a patient. This is because after patients undergo debulking surgery and their tumor samples get implanted into mice, drug testing is not performed on these mice but rather on the later generations of these founding generations, given the need for expanding the initial tumor into a sufficient number of mice. Moreover, the use of personalized medicine approaches requires genetic, protein and metabolic analysis for every patient, thus increasing the cost of diagnosis and treatment significantly. Other confounding factors are the difficulty to obtain new drug entities from pharmaceutical companies, Material Transfer Agreements (MTAs) and pending patents on these new drug compounds all challenge the availability of therapeutic agents.
Engraftment failure
When the tumor is transplanted into the mouse model, it is largely dependent on the type of tumor whether it will engraft and propagate or not. For example, the engraftment rate of the HG-SOC is one of the highest while mucinous OC has the lowest (6).
As engraftment failures can skew results by focusing on survival in patients whose Avatars did or did not engraft, it should be obvious that PDX models must be chosen to be proven to have high engraftment rate (6). A general sense in this regard is that the more aggressive the tumor, the high engraftment rate it typically demonstrates.
Alteration of microenvironment of the human tumor with murine stroma
Many studies have shown that human stroma surrounding transplanted tumors becomes rapidly replaced across generations by murine stroma (44). Such background constitutes many of the tumor targets for response and resistance, such as fibroblasts, blood vessels, and inflammatory and immune mediating cells. This surrounding framework could alter the paracrine control, response, and interstitial pressure around the tumor, with a resultant suboptimal model for chemotherapeutic trials related to host responses. Likewise, the effect of chemotherapeutic agents themselves might become affected by the different foreign microenvironment. This can be evident when observing a response caused by unusually high dose in the mouse model, while in reality this is pure over-prediction of human dose response, and vice versa (45,46).
Evolving nature of human tumors
Since cancer is essentially a continuous dynamic disease process and not a one-time event of pathologic incident, we need to be cautious regarding the evolution of tumor in human tissue versus the static nature of its graft implanted into the mouse model tissues. It is well established there is a genetic evolution in cancer as the tumor progresses. Swanton and colleagues have examined the intra-tumor heterogeneity of primary renal carcinomas and associated metastatic sites. Phylogenetic reconstruction revealed branched evolutionary tumor growth, with 63-69% of all somatic mutations not detectable across every tumor region (47). Similarly, Iacobuzio-Donahue and colleagues evaluated the clonal relationships among seven primary pancreatic cancer metastases. They found that clonal populations that give rise to distant metastases are represented within the primary carcinoma, but these clones are genetically evolved from the original parental, non-metastatic clone (48). Because of the time elapsing between harvesting the tumor from a patient and initiation of treatment after obtaining results from the mouse model; there is some likelihood that the tumor has changed both genetically and phenotypically in its patient (47).
Standardized reporting methods
Despite concordance of most groups with uniform protocols of PDX generation, each group has developed their unique experience and approaches, but few groups have published these newly developed methods or materials. Issues such as time spent on processing the specimen, best preservation media, the need to add other components, such as Matrigel or mesenchymal cells, tumor injection techniques, site of implantation (SC, orthotopic, or renal cell capsule) for best results are currently unknown. In order to apply comparative and uniform standards for PDX models evaluation, it is necessary for every institute to publish more details regarding genotypes, histotypes, molecular phenotypes, chemotherapeutic naivety, stability over generations, and whether any new materials were implemented during the process of generating their own PDX models.
Lymphomagenesis
Lymphoma development in xenografted solid tumors has been reported by many groups (6,8,49). The pathogenesis of this phenomenon is not well established. Ghanekar and colleagues has shown that the lymphoid neoplasms were Epstein-Barr virus (EBV)-associated human B-cell lymphomas. It was hypothesized that the human lymphomas have developed from reactivation of latent EBV residing in donor B lymphocytes following their xenotransplantation into immunodeficient recipient mice (49). Certain mouse strains, like SCID mice are more prone to spontaneously develop lymphomas. Bosma and colleagues have detected spontaneous T-Cell Lymphoma formation in 41 of 269 SCID mice, none was found to express B-cell markers (50).
Social and personal obstacles
Patient enrollment in personalized medicine clinical trials is a critical factor which determines the success of a clinical trial. Adequate enrollment secures a base for proposed participant retention. In order to obtain final conclusive data as regards the efficacy of a new therapeutic strategy, sufficient participant retention is required, underpowered clinical data is doubtful to provide any decisive evidence. Many factors step back patients from participating in clinical trials such as, but not limited to; Lack of knowledge of ongoing clinical trials, public mistaken belief of clinical trials “Fear of being a guinea pig”, hesitancy of under-represented populations, administrative procedures associated with enrollment in clinical trials, lack of available and appropriate protocols. Concerns about cost are also a major limiting factor. Lack or fear of being denied of insurance coverage, confusion over what is covered by Medicare, costs of being away from work and family are restraints for some patients. Results from a National Cancer Institute study showed that patients with fee-for-service coverage were more than twice as likely as HMO/PPO/Medicare patients to be enrolled in a clinical trial (51,52).
Personalized immune Avatar models
Currently available Avatar models have shown to be useful tool in preclinical settings. However, they impede studying interactions between immune and cancer cells. An ideal model to study tumor progression and response to therapy will allow preclinical testing of new therapeutics in immune-competent, spontaneous tumor scenery to define efficacy and ascertain predictive biomarkers of response. The lack of immune response can considerably impact tumor formation as well as therapeutic efficacy.
Genetic-chimera models are genetically engineered mice in which human genes/chromosomes are introduced into the mouse genome. Kymouse and VelocImmune mice represent this model type, which carry human immunoglobulin genes (53,54). These mice has been used in clinical trials for agents such as Nesvacumab, which binds Angiopoietin-2, and Enoticumab, which binds Dll4 in where they yield entirely humanized antibodies (54,55). MITRG and MISTRG are two mouse strains, in which human versions of four genes encoding cytokines vital for innate immune cell development are knocked into their corresponding mouse loci. The human cytokines support the development and function of monocytes, macrophages and NK cells derived from human fetal liver or adult CD34+ progenitor cells injected into the mice. Human tumor xenograft in MITRG and MISTRG is intruded with human macrophages in a fashion similar to that observed in tumors obtained from human patients. This model may be used to explore the human immune system in circumstances of health and pathology; it may also empower the evaluation of therapeutic nominees in an in vivo venue pertinent to human physiology (56).
On the other hand, cellular-chimera models can be developed by introducing human cells into immune-compromised mice and provide upsurge to human tissues in the mouse. To accomplish these experiments the newborn mice need to be irradiated and then engrafted with human CD34+ hematopoietic stem cells from human umbilical cord blood or bone marrow (57). A xenograft model co-engrafted with human tumors and autologous tumor-associated T and B cells would give us the opportunity to study their interactions in vivo and observe how human immunocompetent cells and tumor cells influence each other. This approach could hypothetically be used to evaluate immunotherapeutic methodologies to cancer.
Conclusions
Avatar models allow evaluation of drug response, safety, and efficacy in cancer patients with good concordance to the corresponding patient original tumors. They have the potential to provide significant improvement in clinical outcomes, and for defining optimal patients for clinical trials. Despite limitations to their use including further investigation in regards to microenvironment mimicry and metastatic case applicability, we feel they provide a promising approach to facilitate future research in OC.
Acknowledgements
Funding: This work was supported by the following United States National Institutes of Health Grants: Mayo Clinic SPORE in Ovarian Cancer, CA136393 and NCI R01 CA184502.
Footnote
Conflicts of Interest: The authors have no conflicts of interest to declare.
References
- Cancer Facts & Figures. Available online: http://www.cancer.org/research/cancerfactsstatistics/cancerfactsfigures2014/
- van Dalen A, Favier J, Burges A, et al. Prognostic significance of CA 125 and TPS levels after 3 chemotherapy courses in ovarian cancer patients. Gynecol Oncol 2000;79:444-50. [PubMed]
- Hutchinson L, Kirk R. High drug attrition rates--where are we going wrong? Nat Rev Clin Oncol 2011;8:189-90. [PubMed]
- Jayson GC, Kohn EC, Kitchener HC, et al. Ovarian cancer. Lancet 2014;384:1376-88. [PubMed]
- Gillet JP, Calcagno AM, Varma S, et al. Redefining the relevance of established cancer cell lines to the study of mechanisms of clinical anti-cancer drug resistance. Proc Natl Acad Sci U S A 2011;108:18708-13. [PubMed]
- Weroha SJ, Becker MA, Enderica-Gonzalez S, et al. Tumorgrafts as in vivo surrogates for women with ovarian cancer. Clin Cancer Res 2014;20:1288-97. [PubMed]
- Elkas JC, Baldwin RL, Pegram M, et al. A human ovarian carcinoma murine xenograft model useful for preclinical trials. Gynecol Oncol 2002;87:200-6. [PubMed]
- Topp MD, Hartley L, Cook M, et al. Molecular correlates of platinum response in human high-grade serous ovarian cancer patient-derived xenografts. Mol Oncol 2014;8:656-68. [PubMed]
- Singh S, Ross SR, Acena M, et al. Stroma is critical for preventing or permitting immunological destruction of antigenic cancer cells. J Exp Med 1992;175:139-46. [PubMed]
- Ochsenbein AF, Klenerman P, Karrer U, et al. Immune surveillance against a solid tumor fails because of immunological ignorance. Proc Natl Acad Sci U S A 1999;96:2233-8. [PubMed]
- Zhang B, Bowerman NA, Salama JK, et al. Induced sensitization of tumor stroma leads to eradication of established cancer by T cells. J Exp Med 2007;204:49-55. [PubMed]
- Orimo A, Gupta PB, Sgroi DC, et al. Stromal fibroblasts present in invasive human breast carcinomas promote tumor growth and angiogenesis through elevated SDF-1/CXCL12 secretion. Cell 2005;121:335-48. [PubMed]
- Bombara MP, Webb DL, Conrad P, et al. Cell contact between T cells and synovial fibroblasts causes induction of adhesion molecules and cytokines. J Leukoc Biol 1993;54:399-406. [PubMed]
- Nazareth MR, Broderick L, Simpson-Abelson MR, et al. Characterization of human lung tumor-associated fibroblasts and their ability to modulate the activation of tumor-associated T cells. J Immunol 2007;178:5552-62. [PubMed]
- Barnas JL, Simpson-Abelson MR, Brooks SP, et al. Reciprocal functional modulation of the activation of T lymphocytes and fibroblasts derived from human solid tumors. J Immunol 2010;185:2681-92. [PubMed]
- Allen M, Louise Jones J. Jekyll and Hyde: the role of the microenvironment on the progression of cancer. J Pathol 2011;223:162-76. [PubMed]
- Whiteside TL. The tumor microenvironment and its role in promoting tumor growth. Oncogene 2008;27:5904-12. [PubMed]
- Simpson-Abelson MR, Sonnenberg GF, Takita H, et al. Long-term engraftment and expansion of tumor-derived memory T cells following the implantation of non-disrupted pieces of human lung tumor into NOD-scid IL2Rgamma(null) mice. J Immunol 2008;180:7009-18. [PubMed]
- Lee CH, Xue H, Sutcliffe M, et al. Establishment of subrenal capsule xenografts of primary human ovarian tumors in SCID mice: potential models. Gynecol Oncol 2005;96:48-55. [PubMed]
- Press JZ, Kenyon JA, Xue H, et al. Xenografts of primary human gynecological tumors grown under the renal capsule of NOD/SCID mice show genetic stability during serial transplantation and respond to cytotoxic chemotherapy. Gynecol Oncol 2008;110:256-64. [PubMed]
- Erez N, Truitt M, Olson P, et al. Cancer-Associated Fibroblasts Are Activated in Incipient Neoplasia to Orchestrate Tumor-Promoting Inflammation in an NF-kappaB-Dependent Manner. Cancer Cell 2010;17:135-47. [PubMed]
- Auersperg N, Wong AS, Choi KC, et al. Ovarian surface epithelium: biology, endocrinology, and pathology. Endocr Rev 2001;22:255-88. [PubMed]
- Kaneko T, Saito H, Toya M, et al. Hyaluronic acid inhibits apoptosis in granulosa cells via CD44. J Assist Reprod Genet 2000;17:162-7. [PubMed]
- Rump A, Morikawa Y, Tanaka M, et al. Binding of ovarian cancer antigen CA125/MUC16 to mesothelin mediates cell adhesion. J Biol Chem 2004;279:9190-8. [PubMed]
- Sale S, Orsulic S. Models of ovarian cancer metastasis: Murine models. Drug Discov Today Dis Models 2006;3:149-54. [PubMed]
- Hunter KW. Host genetics and tumour metastasis. Br J Cancer 2004;90:752-5. [PubMed]
- Cheon DJ, Orsulic S. Mouse models of cancer. Annu Rev Pathol 2011;6:95-119. [PubMed]
- Richmond A, Su Y. Mouse xenograft models vs GEM models for human cancer therapeutics. Dis Model Mech 2008;1:78-82. [PubMed]
- McShane LM, Hunsberger S, Adjei AA. Effective incorporation of biomarkers into phase II trials. Clin Cancer Res 2009;15:1898-905. [PubMed]
- Tentler JJ, Tan AC, Weekes CD, et al. Patient-derived tumour xenografts as models for oncology drug development. Nat Rev Clin Oncol 2012;9:338-50. [PubMed]
- van der Meel R, Gallagher WM, Oliveira S, et al. Recent advances in molecular imaging biomarkers in cancer: application of bench to bedside technologies. Drug Discov Today 2010;15:102-14. [PubMed]
- Hidalgo M, Amant F, Biankin AV, et al. Patient-derived xenograft models: an emerging platform for translational cancer research. Cancer Discov 2014;4:998-1013. [PubMed]
- Kelland LR. Of mice and men: values and liabilities of the athymic nude mouse model in anticancer drug development. Eur J Cancer 2004;40:827-36. [PubMed]
- Ledermann J, Harter P, Gourley C, et al. Olaparib maintenance therapy in patients with platinum-sensitive relapsed serous ovarian cancer: a preplanned retrospective analysis of outcomes by BRCA status in a randomised phase 2 trial. Lancet Oncol 2014;15:852-61. [PubMed]
- Vidal A, Muñoz C, Guillén MJ, et al. Lurbinectedin (PM01183), a new DNA minor groove binder, inhibits growth of orthotopic primary graft of cisplatin-resistant epithelial ovarian cancer. Clin Cancer Res 2012;18:5399-411. [PubMed]
- Kola I, Landis J. Can the pharmaceutical industry reduce attrition rates? Nat Rev Drug Discov 2004;3:711-5. [PubMed]
- An MW, Mandrekar SJ, Sargent DJ. A 2-stage phase II design with direct assignment option in stage II for initial marker validation. Clin Cancer Res 2012;18:4225-33. [PubMed]
- Mandrekar SJ, Sargent DJ. Clinical trial designs for predictive biomarker validation: theoretical considerations and practical challenges. J Clin Oncol 2009;27:4027-34. [PubMed]
- Freidlin B, McShane LM, Korn EL. Randomized clinical trials with biomarkers: design issues. J Natl Cancer Inst 2010;102:152-60. [PubMed]
- Sargent DJ, Conley BA, Allegra C, et al. Clinical trial designs for predictive marker validation in cancer treatment trials. J Clin Oncol 2005;23:2020-7. [PubMed]
- Cree IA, Kurbacher CM, Lamont A, et al. A prospective randomized controlled trial of tumour chemosensitivity assay directed chemotherapy versus physician's choice in patients with recurrent platinum-resistant ovarian cancer. Anticancer Drugs 2007;18:1093-101. [PubMed]
- Kris MG, Johnson BE, Berry LD, et al. Using multiplexed assays of oncogenic drivers in lung cancers to select targeted drugs. JAMA 2014;311:1998-2006. [PubMed]
- Mandrekar SJ, An MW, Sargent DJ. A review of phase II trial designs for initial marker validation. Contemp Clin Trials 2013;36:597-604. [PubMed]
- Maykel J, Liu JH, Li H, et al. NOD-scidIl2rg (tm1Wjl) and NOD-Rag1 (null) Il2rg (tm1Wjl): a model for stromal cell-tumor cell interaction for human colon cancer. Dig Dis Sci 2014;59:1169-79. [PubMed]
- Junttila MR, de Sauvage FJ. Influence of tumour micro-environment heterogeneity on therapeutic response. Nature 2013;501:346-54. [PubMed]
- De Wever O, Mareel M. Role of tissue stroma in cancer cell invasion. J Pathol 2003;200:429-47. [PubMed]
- Gerlinger M, Rowan AJ, Horswell S, et al. Intratumor heterogeneity and branched evolution revealed by multiregion sequencing. N Engl J Med 2012;366:883-92. [PubMed]
- Yachida S, Jones S, Bozic I, et al. Distant metastasis occurs late during the genetic evolution of pancreatic cancer. Nature 2010;467:1114-7. [PubMed]
- Chen K, Ahmed S, Adeyi O, et al. Human solid tumor xenografts in immunodeficient mice are vulnerable to lymphomagenesis associated with Epstein-Barr virus. PLoS One 2012;7:e39294. [PubMed]
- Custer RP, Bosma GC, Bosma MJ. Severe combined immunodeficiency (SCID) in the mouse. Pathology, reconstitution, neoplasms. Am J Pathol 1985;120:464-77. [PubMed]
- Clinical Trials Recruitment and Enrollment: Attitudes, Barriers, and Motivating Factors. Available online: http://cro.rbhs.rutgers.edu/documents/clinical_trials_recruitment_and_enrollment.pdf
- Frank G. Current challenges in clinical trial patient recruitment and enrollment. SoCRA Source 2004:30-8.
- Lee EC, Liang Q, Ali H, et al. Complete humanization of the mouse immunoglobulin loci enables efficient therapeutic antibody discovery. Nat Biotechnol 2014;32:356-63. [PubMed]
- Macdonald LE, Karow M, Stevens S, et al. Precise and in situ genetic humanization of 6 Mb of mouse immunoglobulin genes. Proc Natl Acad Sci U S A 2014;111:5147-52. [PubMed]
- Murphy AJ, Macdonald LE, Stevens S, et al. Mice with megabase humanization of their immunoglobulin genes generate antibodies as efficiently as normal mice. Proc Natl Acad Sci U S A 2014;111:5153-8. [PubMed]
- Rongvaux A, Willinger T, Martinek J, et al. Development and function of human innate immune cells in a humanized mouse model. Nat Biotechnol 2014;32:364-72. [PubMed]
- Kalscheuer H, Danzl N, Onoe T, et al. A model for personalized in vivo analysis of human immune responsiveness. Sci Transl Med 2012;4:125ra30.